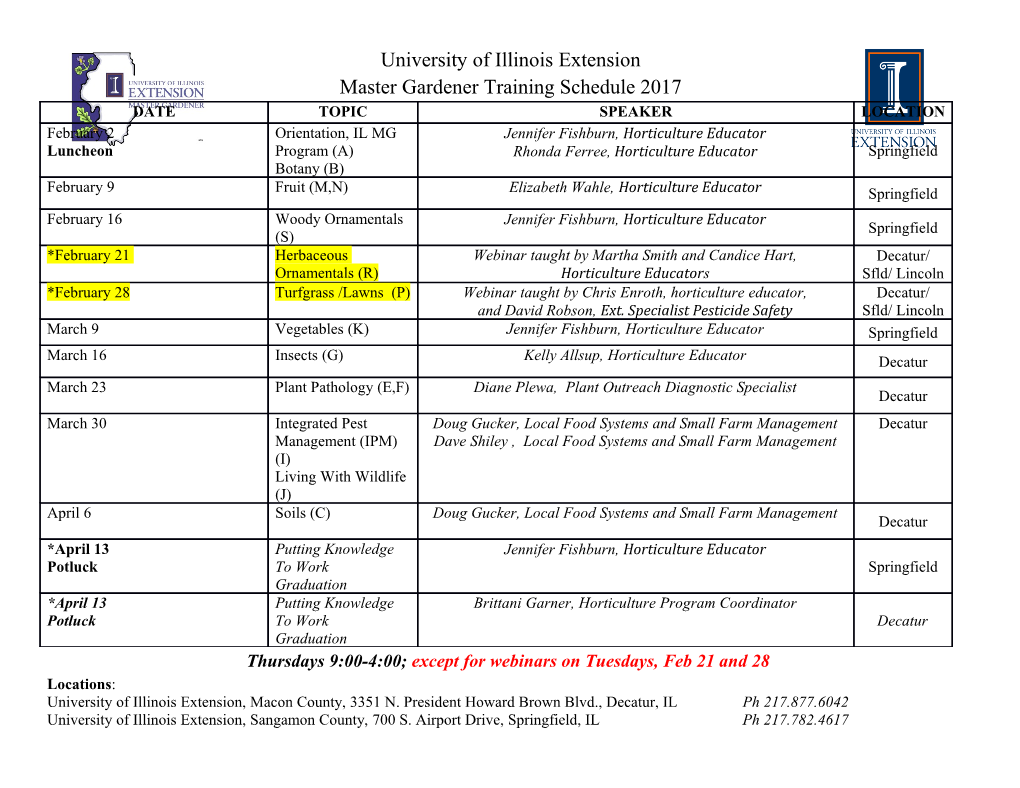
Time Series Analysis: 4. Linear filters P. F. Góra http://th-www.if.uj.edu.pl/zfs/gora/ 2012 Linear filters in the Fourier domain Filtering: Multiplying the transform by a transfer function. gn −! Gn −! H(fn)Gn −! ~gn (1) DFT inverse DFT where H(fn) is the transfer function discretized over fn. The most important drawback: You need to know the whole series, collect all data, prior to filtering. Copyright c 2009-12 P. F. Góra 4–2 Example 1 8 <1 jfj 6 fc 0.8 H(f) = :0 jfj > fc 0.6 4 H(f) 2 exp −(f=fc) H(f) = 4 0.4 1 + exp −(f=fc) 0.2 0 0 1 2 3 4 f Copyright c 2009-12 P. F. Góra 4–3 2 1 0 -1 -2 4 6 8 10 12 14 16 18 2 1 0 -1 -2 4 6 8 10 12 14 16 18 2 1 0 -1 -2 4 6 The signal8 before10 and12 after filtering14 16 18 Copyright c 2009-12 P. F. Góra 4–4 A reason for the strange result of the “blue” filtering 2 1 0 -1 -2 4 6 8 10 12 14 16 18 When filtering in the Fourier domain, we usually do not smooth out the filters. Copyright c 2009-12 P. F. Góra 4–5 Linear filters Filtering in Fourier domain is very easy: multiply the DFT of the input by the transfer function. Filtering in the signal domain can be tricky: a FIR filter: x(t) y(t) r(t) an IIR filter: x(t) y(t) r(t) Copyright c 2009-12 P. F. Góra 4–6 The transfer function Even though the filters are realized in the signal domain, it is convenient to ana- lyse them in Fourier domain. Every possible linear filter is represented by Y (f) = H(f)X(f) : (2) H(f) is the transfer function. Copyright c 2009-12 P. F. Góra 4–7 Terminology •H (f) = 0 for jfj > f0 — a low-pass filter. •H (f) = 0 for jfj 6 f0 — a high-pass filter. •H (f) 6= 0 for f1 6 jfj 6 f2 — a band-pass filter. •H (f) = 0 for f1 6 jfj 6 f2 — a band-stop filter; if f2 − f1 is small, also known as a notch filter. Copyright c 2009-12 P. F. Góra 4–8 A general linear filter in the signal domain has the form q p X X yn = αkxn−k + βkyn−k (3) k=−s k=1 xn is a (discretized) input signal, yn is the output signal. If s > 0, future values of the input are needed to construct the output. Such filter is non-causal. It cannot be realized on-line (or in real time). Copyright c 2009-12 P. F. Góra 4–9 Eq. (3): q p X X yn = αkxn−k + βkyn−k k=−s k=1 • If p = 0, the filter is a Finite Impulse Response filter (FIR), or a moving average filter. The output of such a filter dies out in a finite time after the input has died out. • If p > 0, the filter is Infinite Impulse Response filter (IIR), or an autoregres- sive filter. Its output can go on infinitely long after the input has died out (in fact, this is a parasitic behaviour). Copyright c 2009-12 P. F. Góra 4–10 Causal FIR filters q X yn = αkxn−k : (4) k=0 If the input is stationary, the output also is. q is called the order of the filter. Copyright c 2009-12 P. F. Góra 4–11 To find the transfer function, we Fourier transform Eq. (4). N−1 N−1 q 1 X 2πimn=N 1 X 2πimn=N X Ym = p e yn = p e αkxn−k N n=0 N n=0 k=0 q N−1 q N−1−k X 1 X 2πimn=N X 1 X 2πim(n0+k)=N = αkp e xn−k = αkp e xn0 : k=0 N n=0 k=0 N n0=−k (5) Copyright c 2009-12 P. F. Góra 4–12 By the assumption of periodicity of the input, x−l ≡ xN−l. On the other hand, exp(2πim(N−l)=N)= exp(2πim) exp(2πim(−l)=N)= exp(2πim(−l)=N). Thus q N−1 q X 2πimk=N 1 X 2πimn0=N X 2πimk=N Ym = αke p e xn0 = αke Xm : (6) k=0 N n0=0 k=0 | {z } | {z } Xm Hm m=N = m=(N∆) ∆ = fm∆, where fm is the m-th discrete Fourier frequency. Therefore. Copyright c 2009-12 P. F. Góra 4–13 Transfer function of a causal FIR filter has the form q k X 2πifm∆ 2πifm∆ H(fm) = αk e = α e ; (7) k=0 where α(z) is the following polynomial of order q q X k α(z) = αkz (8) k=0 (coefficients of the filter become coefficients of the polynomial (8)). Usually, for the sake of simplicity, we assume that ∆ = 1, or that the sam- pling time is the time unit. Remember: With this notation, frequencies become dimensionless and the Nyquist interval equals [−1=2; 1=2]. Copyright c 2009-12 P. F. Góra 4–14 Transfer function of a general FIR filter The above can be easily generalized to arbitrary (non-causal) FIR filters. The transfer function becomes q k X 2πifm∆ 2πifm∆ H(fm) = αk e = α e ; (9) k=−s where α(·) is now an appropriate rational function. Copyright c 2009-12 P. F. Góra 4–15 Simple low- and high-pass filters Consider a filter 1 1 1 y = x + x + x : (10) n 4 n−1 2 n 4 n+1 Its transfer function reads 1 2πif 1 1 −2πif 1 1 2 Hc(f) = e + + e = + cos 2πf = cos πf : (11) 4 2 4 2 2 Similarly, the transfer function of the filter 1 1 1 y = − x + x − x : (12) n 4 n−1 2 n 4 n+1 reads 1 2πif 1 1 −2πif 1 1 2 Hs(f) = − e + − e = − cos 2πf = sin πf : (13) 4 2 4 2 2 Copyright c 2009-12 P. F. Góra 4–16 (10) is a (poor) low-pass filter. (12) is a (poor) high-pass filter. 1 1 0.75 0.75 f f π π 2 0.5 2 0.5 sin cos 0.25 0.25 0 0 -0.5 -0.25 0 0.25 0.5 -0.5 -0.25 0 0.25 0.5 f f Copyright c 2009-12 P. F. Góra 4–17 The “usual” moving average The (unweighted) moving average 1 y = x + x + ··· + x + ··· + x + x ; (14) n 2l + 1 n−l n−l+1 n n+l−1 n+l with the transfer function 1 H (f) = (1 + 2 cos 2πf + 2 cos 4πf + ··· + 2 cos 2lπf) (15) MA 2l + 1 is a poor low-pass filter. Copyright c 2009-12 P. F. Góra 4–18 Transfer functions of unweighted moving averages 1 l=1 l=2 l=3 l=5 0.75 (f)| 0.5 MA |H 0.25 0 -0.5 -0.25 0 0.25 0.5 f Copyright c 2009-12 P. F. Góra 4–19 An example of the usual moving average (the noise of the order of the signal) l= 0 l= 1 Moving Averages l= 5 l=16 Copyright c 2009-12 P. F. Góra 4–20 Differentiating filters First derivative: dg 1 g(x) − g(x − ∆) g(x + ∆) − g(x) 1 1 ' + = g(x + ∆) − g(x − ∆) (16a) dx x 2 ∆ ∆ 2∆ 2∆ 1 1 y = x − x 1 (16b) n 2∆ n+1 2∆ n− i H(f) = sin(2πf∆) (16c) ∆ Second derivative: 1 1 1 y = x − x + x 1 (17a) n 4∆2 n+1 2∆2 n 4∆2 n− 1 H(f) = − sin(πf∆) (17b) ∆2 Copyright c 2009-12 P. F. Góra 4–21 2.5 2.0 1.5 1.0 n y 0.5 0.0 -0.5 -1.0 -1.5 0 5 10 15 20 25 30 n Copyright c 2009-12 P. F. Góra 4–22 2.0 1.5 1.0 0.5 n y 0.0 -0.5 -1.0 -1.5 -2.0 0 5 10 15 20 25 30 n Copyright c 2009-12 P. F. Góra 4–23 Phase of the transfer function In Fourier domain, Y (f) = H(f)X(f) : (18) iφ(f) H(f) = R(f)e , R(f) > 0. The modulus of the transfer function ampli- fies/reduces contributions from the corresponding frequencies. What does the phase, φ(f), do? Copyright c 2009-12 P. F. Góra 4–24 The filter (10) is non-causal, but it is easy to find its causal version: we need to introduce a time delay: 1 1 1 y = x + x + x (19) n 4 n−2 2 n−1 4 n with the transfer function H(f) = e2πif cos2 πf : (20) This transfer function differs from that of (10) only by a phase factor. The phase factor in (20) is responsible for the time delay! Copyright c 2009-12 P. F. Góra 4–25 Suppose that the phase of the transfer function depends linearly on frequencies, φ(f) = af∆. Calculate the inverse transform: X −2πifnk∆ X iafn∆ −2πifnk∆ yk(t) ∼ H(fn)X(fn)e = R(fn)e X(fn)e n n X −2πif (k−a)∆ = R(fn)X(fn)e n ; (21) n which corresponds to a time shift of a units (“channels”).
Details
-
File Typepdf
-
Upload Time-
-
Content LanguagesEnglish
-
Upload UserAnonymous/Not logged-in
-
File Pages52 Page
-
File Size-