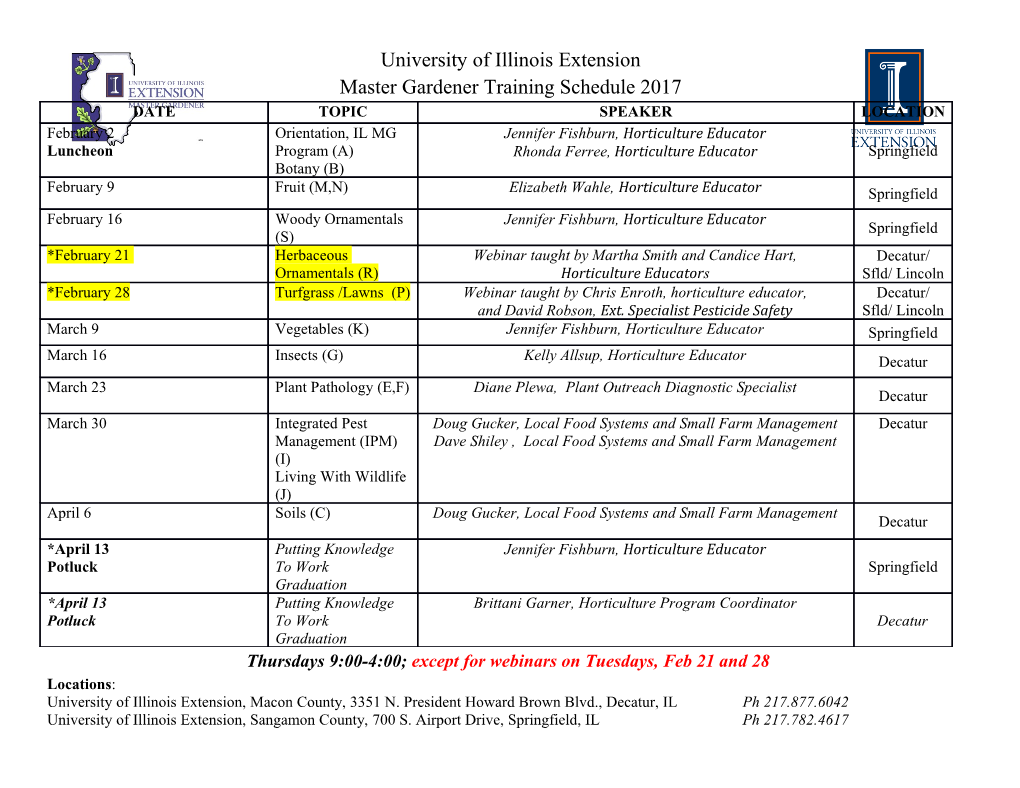
Statistical Methods in Particle Physics 2. Probability Distributions Prof. Dr. Klaus Reygers (lectures) Dr. Sebastian Neubert (tutorials) Heidelberg University WS 2017/18 Gaussian https://en.wikipedia.org/wiki/Normal_distribution 1 (x µ)2 g(x; µ, σ)= exp − p − 2σ2 2⇡ ✓ ◆ Mean: E[x]=µ Variance: V [x]=σ2 1 x2 μ = 0, σ = 1 ("standard normal distribution"): φ(x)= e− 2 p2⇡ Cumulative distribution related to error function: x 1 z2 1 x Φ(x)= e− 2 dz = erf +1 p2⇡ 2 p2 Z1 ✓ ◆ Statistical Methods in Particle Physics WS 2017/18 | K. Reygers | 2. Probability Distributions 2 p-value Probability for a Gaussian distribution corresponding to [μ – Zσ, μ +Zσ]: +Z 1 x2 Z P(Zσ)= e− 2 dx =Φ(Z) Φ( Z)=erf p2⇡ Z − − p2 Z− ✓ ◆ 68.27% of area within ±1σ 90% of area within ±1.645σ 95.45% of area within ±2σ 95% of area within ±1.960σ 99.73% of area within ±3σ 99% of area within ±2.576σ p-value: Two-sided Gaussian p-values probability that a random process produces a measurement thus far, or Deviation p-value (%) further, from the true mean 1 σ 31.7 2 σ 4.56 p-value =1 P(Zσ) 3 σ 0.270 − 4 σ 0.006 33 standard to report In root: TMath::Prob a “discovery” 5 σ 0.000 057 3 Statistical Methods in Particle Physics WS 2017/18 | K. Reygers | 2. Probability Distributions 3 Why Are Gaussians so Useful? Central limit theorem: When independent random variables are added, their properly normalized sum tends toward a normal distribution (a bell curve) even if the original variables themselves are not normally distributed. More specifically: Consider n random variables with finite variance σi2 and arbitrary pdf: n n n n y = x 2 i !1 E[y]= µi V [y]= σi i=1 i=1 X X Xi=1 Measurement uncertainties are often the sum of many independent contributions. The underlying pdf for a measurement can therefore be assumed to be a Gaussian. Many convenient features in addition, e.g., sum or difference of two Gaussian random variables is again a Gaussian. Statistical Methods in Particle Physics WS 2017/18 | K. Reygers | 2. Probability Distributions 4 The CLT at Work A: x taken from a uniform PD in [0,1], B: X = x1+x2 from A, 2 with µ=0.5 and σ =1/12, N=5000 N=5000, flat shoulders C: X = x1+x2+x3 from A, curved shoulders D: X=x1+x2+…+x12 from A, almost Gaussian Statistical Methods in Particle Physics WS 2017/18 | K. Reygers | 2. Probability Distributions 5 Multivariate Gaussian transposed column (row) vectors vectors 1 1 T 1 f (~x; µ~, V )= exp (~x µ~) V − (~x µ~) (2⇡)N/2 V 1/2 −2 − − | | ~x =(x1,...,xn), µ~ =(µ1,...,µn) E[x ]=µ V = cov[x , x ]= (x µ )(x µ ) i i i,j i j h i − i j − j i For n = 2: 2 2 σx ⇢x σy 1 1 1/σx ⇢/(σx σy ) V = 2 V − = − 2 ⇢x σy σ (1 ⇢2) ⇢/(σx σy )1/σ ✓ y ◆ − ✓− y ◆ ρ = correlation coefficient Statistical Methods in Particle Physics WS 2017/18 | K. Reygers | 2. Probability Distributions 6 2d Gaussian Distribution and Error Ellipse We obtain the 2d Gaussian distribution: 1 f (x1, x2; µ1, µ2, σ1, σ, ⇢)= 2⇡ σ 1 ⇢2 ⇥ 1 2 − 2 2 1 x1 µ1 p x2 µ2 x1 µ1 x2 µ2 exp − + − 2⇢ − − −2(1 ⇢2) σ σ − σ σ − "✓ 1 ◆ ✓ 2 ◆ ✓ 1 ◆✓ 2 ◆#! where ρ = cov(x1, x2)/(σ1σ2) is the correlation coefficient. Lines of constant probability correspond to constant argument of exp → this defines an ellipse 1σ ellipse: f(x1, x2) has dropped to 1/√e of its maximum value (argument of exp is –1/2): x µ 2 x µ 2 x µ x µ 1 − 1 + 2 − 2 2⇢ 1 − 1 2 − 2 =1 ⇢2 σ σ − σ σ − ✓ 1 ◆ ✓ 2 ◆ ✓ 1 ◆✓ 2 ◆ Statistical Methods in Particle Physics WS 2017/18 | K. Reygers | 2. Probability Distributions 7 2d Gaussian: Error Ellipse 1D projections http://www.phas.ubc.ca/~oser/p509/Lec_07.pdf s =21 fy (x)=x f (x, y)dy s =1Z1 y • PDF projections are2 (1D) Gaussians: Ellipse which contains 1 1 x µx r==0.8• Areasexp of 1σ and− 2σ 68% of the events p2⇡ −2 σx contoursx differ✓ ◆ ! 1σ ellipse (1/√e of Red ellipse:in 1D and 2D! maximum values) contour with 1 1 y µ 2 f (y)=argumentexp of − y x p −2 σ exponential2⇡y ✓ y ◆ ! set to equal y -1/2 P1D P2D Blue1σ ellipse:0.6827 0.3934 2σ 1σ x contour2σ 0.9545 0.8647 containing3 0.9973 0.9889 68%σ of 2D probability1.515σ 0.6827 1σ content.2.486σ 0.9545 2σ 3.439σ 0.9973 Physics 509 17 Luca Lista Statistical Methods for Data Analysis 43 Probability for an event to be within 1σ ellipse: 39.34% Statistical Methods in Particle Physics WS 2017/18 | K. Reygers | 2. Probability Distributions 8 Poisson Distribution https://en.wikipedia.org/wiki/Poisson_distribution k µ µ p(k; µ)= e− μ = 1 k! μ = 4 E[k]=µ, V [k]=µ μ = 10 Properties: ‣ n1, n2 follow Poisson distr. → n1+n2 follows Poisson distr., too ‣ Can be approximated by a Gaussian for large ν Examples: ‣ Clicks of a Geiger counter in a given time interval ‣ Number of Prussian cavalrymen killed by horse-kicks Statistical Methods in Particle Physics WS 2017/18 | K. Reygers | 2. Probability Distributions 9 Binomial Distribution N independent experiments ‣ Outcome of each is 'success' or 'failure' ‣ Probability for success is p N k N k f (k; N, p)= p (1 p) − E[k]=Np V [k]=Np(1 p) k − − ✓ ◆ N N! = binomial coefficient: number of different ways k k!(N k)! (permutations) to have k successes in N tries ✓ ◆ − Use binomial distribution to model processes with two outcomes ‣ Example: Detection efficiency (either we detect particle or not) For small p, the binomial distribution can be approximated by a Poisson distribution (more exactly, in the limit N → ∞, p → 0, N·p constant) Statistical Methods in Particle Physics WS 2017/18 | K. Reygers | 2. Probability Distributions 10 Negative Binomial Distribution Keep number of successes k fixed and ask for the probability of m failures before having k successes: 1 p m + k 1 E[m]=k − P(m; k, p)= − pk (1 p)m p m − ✓ ◆ 1 p m = 0, 1, ..., V [m]=k − 1 p2 Another representation: E[m]=µ m m + k 1 µ P(m; µ, k)= − k µ m µ m+k V [m]=µ 1+ ✓ ◆ 1+ k k ⇣ ⌘ 1 [relation btw. Use Gamma-fct. for non-integer values p = µ parameters] 1+ k x!:=Γ(x + 1) Example: Distribution of the number of produced particles in e+e– and proton-proton collisions reasonably well described by a NBD. Why? Empirical observation, not so obvious. Statistical Methods in Particle Physics WS 2017/18 | K. Reygers | 2. Probability Distributions 11 72 KAPITEL 4. STATISTIK in den einzelnen Intervallen stark unterschiedlich sind, kann man die Genauigkeit der einzel- nen Datenwerte nicht leicht auf einen Blick einsch¨atzen, weil sie alle verschiedene Varianzen haben. Die folgende Formel transformiert die Zahl der Eintr¨a g e i n j e d e m I n t e r v a l l ri zu neuen Variablen yi,welchealleungef¨ahrdieselbeVarianzvon1haben: y = 2 √r oder auch y = √r + r + 1. i · i i i i Dies sieht man leicht durch Anwendung von Gleichung! (4.51). Die letztere Transformation hat eine Varianz von 1.0 ( 6%) f ¨ur alle Argumente r > 1. ± i 4.5 Spezielle Wahrscheinlichkeitsdichten 4.5.1 Gleichverteilung Diese Wahrscheinlichkeitsdichte ist konstant zwischen denGrenzenx = a und x = b 1 a x < b f (x)= b a ≤ . (4.17) ⎧ − ⎨0außerhalb Sie ist in Abbildung⎩ 4.5 gezeigt. Mittelwert und Varianz sind Uniform Distribution a + b (b a)2 x = E[x]= V [x] = σ2 = − . ⟨ ⟩ 2 12 1 b a , a x b f (x; a, b)= − (0, otherwise 1 b a − Properties: Abbildung 4.5: Die Gleichverteilung mit 1 konstanter Dichte zwischen den Grenzen a E[x]= (a + b) 0 2 a b und b. 1 2 V [x]= (b a) Die Gleichverteilung wird oft U(a, b) geschrieben. Besonders wichtig ist die Verteilung U(0, 1) 12 − mit den Grenzen 0 und 1, die eine Varianz 1/12 (Standardabweichung σ = 1/√12) hat. Example: 4.5.2 Normalverteilung ‣ Strip detector: Die Normal- oder Gauß-Verteilung4 ist die wichtigste Wahrscheinlichkeitsdichte wegen ihrer resolution for one-strip clusters: großen Bedeutung in der Praxis (siehe Kapitel 4.6.3). Die Wahrscheinlichkeitsdichte ist pitch/√12 (x µ)2 1 − f (x)= e− 2σ2 x ( ∞, ∞) .(4.18) √2πσ ∈ − Die Abbildung 4.6 zeigt eine Seite aus der Originalarbeit von Gauß “Motus Corporum Coele- Statistical Methods in Particle Physicsstium”, WS wo2017/18 er | dieK. Reygers Gauß-Funktion | 2. Probability Distributions einf¨uhrt. 12 Die Normalverteilung wird von zwei Parametern bestimmt, µ und σ.DurchdirekteRechnung zeigt man, daß µ = E [x] der Mittelwert ist und σ = V [x] die Standardabweichung. Die 4Korrekt: Gauß’sche Wahrscheinlichkeitsdichte;demallgemeinenBrauchfolgendwirdsiehierauchals! Gauß- Verteilung bezeichnet. Exponential Distribution 1 x/⇠ e− x 0 f (x; ⇠)= ⇠ ≥ (0 otherwise E[x]=⇠ V [x]=⇠2 Example: Decay time of an unstable particle at rest 1 t/⌧ f (t, ⌧)= e− ⌧ = mean lifetime ⌧ Lack of memory (unique to exponential): f (t > t + t t > t )=f (t > t ) 0 1| 0 1 Probability for an unstable nucleus to decay in the next minute is independent of whether the nucleus was just created or already existed for a million years Statistical Methods in Particle Physics WS 2017/18 | K.
Details
-
File Typepdf
-
Upload Time-
-
Content LanguagesEnglish
-
Upload UserAnonymous/Not logged-in
-
File Pages21 Page
-
File Size-