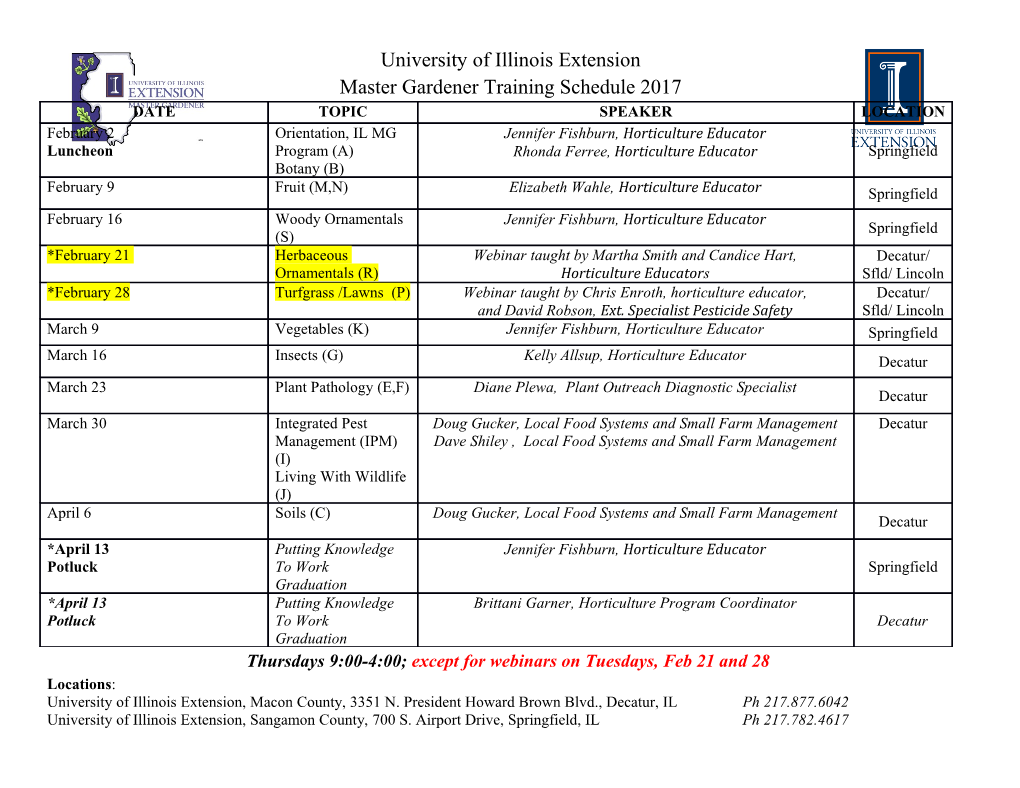
International Journal of Pure and Applied Mathematics Volume 119 No. 12 2018, 14749-14767 ISSN: 1314-3395 (on-line version) url: http://www.ijpam.eu Special Issue ijpam.eu Offline Handwritten Gurmukhi Word Recognition Using Deep Neural Networks 1Neeraj Kumar and 2Sheifali Gupta 1Dept of Electronics & Communication Engineering, Chitkara University, Himachal Pradesh, India. [email protected] 2Dept of Electrical & Electronics Engineering, Chitkara University, Punjab, India. [email protected] Abstract In spite of the advances achieved in pattern recognition technology, Handwritten Gurmukhi Word Recognition (HGWR) is still a challenging problem due to the presence of many similar characters and excessive cursiveness in Gurmukhi handwriting. Even the finest presented recognizers don’t give reasonable performance for realistic applications. Also due to variations in handwriting styles and speed of writing it is very hard to recognize the handwritten Gurmukhi characters. A majority of the work has already been reported on the online handwritten scripts like English, Bangla etc. Now research is being shifted towards the recognition of offline handwritten scripts. In order to recognize the handwritten Gurmukhi word, we present a novel method based on deep neural networks which has recently shown exceptional performance in various pattern recognition and machine learning applications, but has not been endeavored for HGWR. We present Handwritten Gurumukhi Word recognition method based on LBP features, Directional Features & Geometric features. A total of 117 features have been extracted which will be utilized to recognize the individual character for further word recognition. Also to map the recognized Gurmukhi text with Devanagari a suitable mapping technique has also been implemented. The proposed system effectively segments the words into individual characters and then recognizes the individual character, concatenates them and maps the 14749 International Journal of Pure and Applied Mathematics Special Issue Gurmukhi text into Devanagari text using suitable unicodes. Keywords: Word Recognition, LBP features, Directional features, Regional features, Deep neural networks, Mapping. 14750 International Journal of Pure and Applied Mathematics Special Issue 1. Introduction Computers have an excessive influence on everyone in these days and process almost all the important works of our lives electronically. Keeping in mind the use of computers these days, there is a requirement of developing proficient, uncomplicated and speedy methods for the transfer of data between humans and computers. Document Analysis and Recognition (DAR) systems play a main role in transfer of data between humans and computers. Character Recognition system is an essential part of a document analysis and recognition system. Character Recognition systems have been developed to recognize printed texts and handwritten texts [1]. Printed character recognition system deals with the recognition of printed characters whereas handwritten character recognition system (HCRS) deals with the recognition of handwritten characters. Handwritten character recognition system (HCRS) can be categorized into two streams namely, online HCRS and offline HCRS. In online stream, user writes on an electronic surface by the aid of a unique pen and during the writing process, data is captured in terms of (x, y) coordinates. Offline HCRS converts offline handwritten text into such a form which can be easily understood by the machines. It involves the processing of documents containing scanned images of a text written by a user, usually on a plain paper [2]. In this kind of systems, characters are digitized to obtain 2 dimensional images. Developing a realistic HCRS which is capable of retaining lofty recognition accuracy is still a very challenging task. Moreover, recognition accuracy depends upon the input text quality. A number of devices, including personal digital assistant and tablet PCs are available these days that can be used for data capturing. In these systems, characters are captured as a sequence of strokes. Features are then extracted from these strokes and strokes are recognized with the help of these features. Generally, a post-processing module helps in forming the characters from the stroke(s). India is a multilingual country. Multiple scripts are used among billions of people, mainly based on their geographic location. Based on the literature studied so far, it is found that the majority of the work is being done on individual character recognition in different languages like English, Bangla, Devanagari, Gujrati etc. Now researchers are shifting towards the recognition of Gurmukhi script. Gurmukhi script is one most widely used script especially in the north part of the country. So there is a need to develop a recognition system for Gurmukhi script that will reduce the complexity of the work done in Government offices, public banks where majority of the work is done in Gurmukhi script. Research in this script is currently limited to single character recognition only. So there is a huge scope in the Gurmukhi script for researchers to take the work forward from character level to the Word level. In this article an attempt has been made to recognize the Gurmukhi words using combined feature extraction and deep neural networks. 14751 International Journal of Pure and Applied Mathematics Special Issue 2. Gurmukhi Script Normally, each Indian script is having several scrupulous set of laws for the amalgamation of consonants, vowels and modifiers. Gurmukhi script is widely used in North part of the India. Gurmukhi script is used for inscribing the Punjabi language. It is derived from the old Punjabi word “Gurumukhi”, which means “from the mouth of the Guru”. Gurmukhi script has basic 35 characters, 10 vowels and modifiers, 6 additional modified consonants. There is no conception of upper or lowercase characters in Gurmukhi script. The writing style of the Gurmukhi script is from left to right and top to bottom. The basic 35 characters of Gurmukhi script are shown in Fig 1: Fig.1 Consonants in Gurmukhi Script 3. Literature Review R. Sharma et al [1] projected two stages for the character recognition. In the first phase unidentified strokes are recognized and in the second stage the author has evaluated the characters with the help of strokes that are found in the first stage. Using the elastic matching a maximum recognition rate of 90.08% is achieved. N. Kumar et al [3] presented a technique for effective character recognition system based on deep neural networks. The authors extracted three kind of features namely LBP features, directional features and geometric features in order to correctly recognize the text. U. Garain et al [4] proposed a technique which works on fuzzy analysis and authors developed an algorithm to segment touching characters. M. Kumar et al [5] projected a scheme to recognize Gurmukhi characters which is based on transition & diagonal features extraction 14752 International Journal of Pure and Applied Mathematics Special Issue and the classifier used in the work is k-NN. To determine the k-nearest neighbors, the authors calculated the Euclidian distance b/w test point and reference point. Rajiv Kumar et al [6] showed how segmentation can be done in character recognition of Gurmukhi script. According to R.K Sharma et al [7] that with the help of various features like diagonal, directional & zoning & K- NN, Bayesian classifiers the handwriting of writers can be compared. Narang et al [8] projected an approach based on parts or technique suitable for scene images. The author has taken the corner points which are served as parts and these parts are found to make a part based model. N. Kumar et al [9] has discussed a number of feature extraction techniques & classifiers like power arc fitting, parabola arc fitting, diagonal feature extraction, transition feature extraction, k-NN and SVM. M. Kumar et al [10] projected a technique to recognize isolated characters using horizontally & vertically peak extent features, centroid features &diagonal features. A. Dixit et al [11] proposed a feature extraction & classification approach named as the wavelet transform. The proposed technique achieves an accuracy of 70%. N. Arica et al [12] proposed a method for offline cursive handwriting recognition. Firstly, authors found various parameters like baselines, slant angle, stroke width and height. After that, character segmentation paths were found by merging both gray scale and binary information. M. Kumar et al [13] proposed two schemes for feature extraction namely, power and parabola curve fitting & the classifier used in the work is k-NN & SVM. M. Kumar et al [14] presents a novel technique for efficient feature extraction. The authors extracted various features, namely, peak‑ extent features, shadow features and centroid features. They also proposed a novel feature set by using horizontal peak extent features and the vertical peak extent features. A. Aggarwal [16] proposed a feature extraction technique namely gradient based feature extraction for recognition of Gurmukhi characters. The proposed system achieves an accuracy of 97.38%. N. Kumar et al [17] proposed a technique for effective character recognition system based on deep neural networks. The authors also compared the results of proposed technique with various classifiers like SVM & K-NN. 4. Research Methodology The research methodology for word recognition has been divided into
Details
-
File Typepdf
-
Upload Time-
-
Content LanguagesEnglish
-
Upload UserAnonymous/Not logged-in
-
File Pages20 Page
-
File Size-