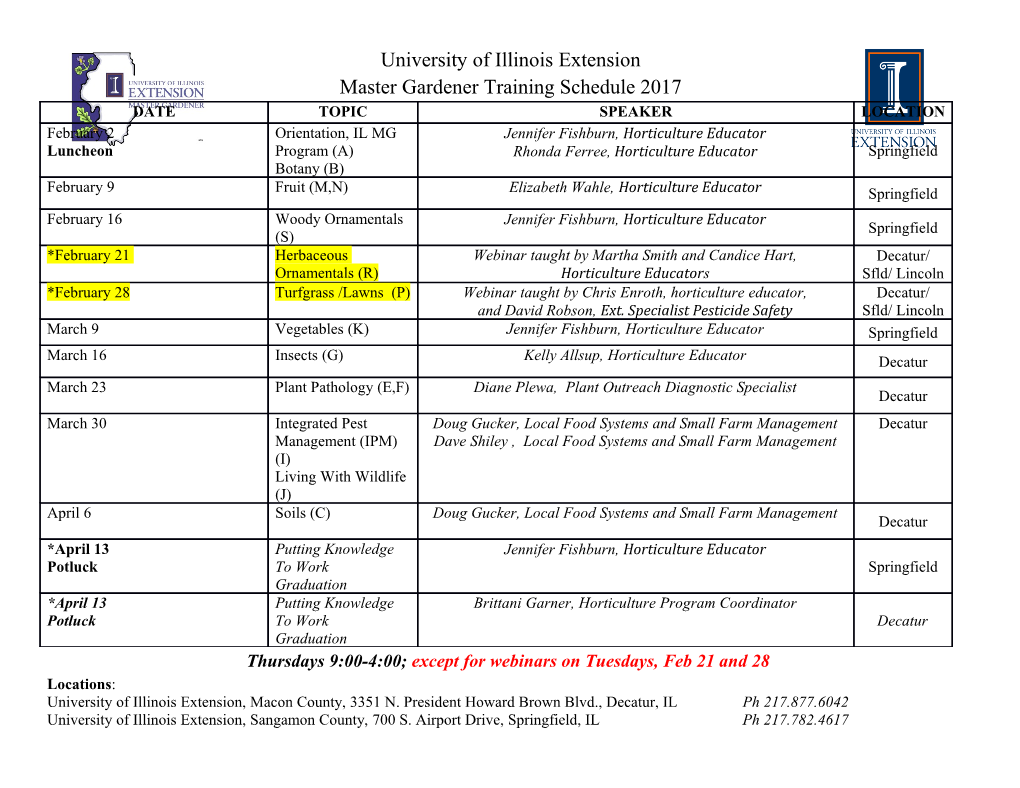
Offline and Online Optimization in Machine Learning Peter Sunehag ANU September 2010 Section Introduction: Optimization with gradient methods Introduction:Machine Learning and Optimization Convex Analysis (Sub)Gradient Methods Online (sub)Gradient Methods Steepest decent I We want to find the minimum of a real valued function n f : R ! R. I We will primarily discuss optimization using gradients I Gradient Decent is also called the steepest decent method Problem: Curvature I If we the level sets are very curved (far from round), gradient decent might take a long route to the optimum I Newton's method takes curvature into account and "corrects" for it. It relies on calculating inverse Hessian (second derivatives) of the objective function I Quasi-Newton methods estimates the "correction" by looking at the path so far. Red Newton, Green Gradient Decent Problem: Zig-Zag I Gradient Descent has a tendency to Zig-Zag I This is particularly problematic in valleys I One solution: Introduce momentum I Another solution: Conjugate gradients Section Introduction: Optimization with gradient methods Introduction:Machine Learning and Optimization Convex Analysis (Sub)Gradient Methods Online (sub)Gradient Methods Optimization in Machine Learning I Data is becoming "unlimited" in many areas. I Computational resources are not I Taking advantage of the data requires efficient optimization I Machine Learning researchers have realized that we are in a different situation compared to the contexts in which the optimization (say convex optimization) field developed. I Some of those methods and theories are still useful. I Different ultimate goal. I We optimize surrogates for what we really want. I We also often have much higher dimensionality than other applications. Optimization in Machine Learning I Known training data A, unknown test data B I We want optimal performance on the test data I Alternatively we have streaming data (or pretend that we do). I Given a loss function L(w; z) (parameters w 2 W , data P sample(s) z), we want as low loss z2B L(z; w) as possible on the test set. I Since we do not have access to the test set, we minimize regularized empirical loss on the training set X L(z; w) + λR(w): z2A I Getting extremely close to the minimum of this objective might not increase performance on the test set. I In Machine learning, the optimization methods of interest are ∗ those that fast get a good estimate wk to the optimum w Optimization in Machine Learning ∗ I How large k to get k = f (wk ) − f (w ) ≤ for a given > 0? 2 p 1 I Is this number like 1/, 1/ ; 1= , log ? 2 I Is k like 1=k; 1=k , etc I How long does an iteration take? I Quasi-Newton methods (BFGS, L-BFGS), are rapid when we are already close to the optimum but otherise not better than methods (gradient decent) with much cheaper iterations. I We care the most about the early phase when we are not close to the optimum Examples T I linear classifier (classes ±1) f (x) = sgn(w x + b). I Training data f(xi ; yi )g I SVMs (hinge loss), P T 2 minw i min(0; 1 − yi (w xi + b)) + λkwk2 exp w T x P yi i 2 I Logistic multi-class: minw i log P T + λkwk2 l exp wl xi ∗ T I Decision l = argmaxl fwl xg I Different regularizers, e.g. kxk1 encourages sparsity. I hinge loss and `1 regularization causes non-differentiability on null sets. Section Introduction: Optimization with gradient methods Introduction:Machine Learning and Optimization Convex Analysis (Sub)Gradient Methods Online (sub)Gradient Methods Gradients I We will make use of gradients with respect to a given coordinate system and its associated Euclidean geometry. I If f (x) is a real valued function that is differentiable at x = (x1; :::; xd ), then @f @f rx f = ( ; :::; ) @x1 @xd I The gradient points in the direction of steepest ascent Subgradients d I If f (x) is a convex real valued function, and if g 2 R is such that f (x) − f (y) ≥ g T (x − y) for all y in some open ball around x, then g is in the subgradient set of f at x. I If f is differentiable, then only rf (x) is in the subgradient set. I A line with slope g can be drawn that only meet f at x Convex set n I A set X ⊂ R is convex if for all x; y 2 X and 0 ≤ λ ≤ 1, (1 − λ)x + λy 2 X Convex function n n I A function f : R ! R is convex if 8λ 2 [0; 1] and x; y 2 R , f ((1 − λ)x + λy) ≤ (1 − λ)f (x) + λf (y): I The set above the function's curve is called its epigraph I A function is convex iff its epigraph isa convex set I A convex function is called closed if the epigraph is closed Strong Convexity n I A function f : R ! R is ρ-strongly convex (ρ > 0) if n 8λ 2 [0; 1] and x; y 2 R , λ(1 − λ) f ((1 − λ)x + λy) ≤ (1 − λ)f (x) + λf (y) − ρ kx − yk2 2 2 2 I or equivalently if f (x) − (ρ/2)kxk2 is convex I or equivalently 2 f (y) ≥ f (x)+ < rf (x); y − x > +(ρ/2)kx − yk2 2 I or equivalently (if twice diff) r f (x) ≥ ρI (id matrix) 4 2 I Green (y = x ) is too flat. Blue (y = x ) is not too flat. Lipschitz Continuity I f is L-Lipschitz if jf (x) − f (y)j ≤ Lkx − yk2 I Lipschitz implies continuity but not differentiability I If differentiable, then bounded (norm) gradients implies Lipschitz continuity. I The secant slopes are bounded by L Lipschitz Continuous Gradients I If f is differentiable, then having L-Lipschitz gradients (L-LipG) means that krf (x) − rf (y)k2 ≤ Lkx − yk2 which is equivalent to L f (y) ≤ f (x)+ < rf (x); y − x > + kx − yk2 2 2 which if f is twice differentiable is equivalent to r2f (x) ≤ L · I . Legendre transform I Transformation between points and lines (set of tangents) I One dimensional functions (generalizes to Fenchel transform) ∗ I f (p) = maxx (px − f (x)). I If f differentiable, the maximizing x has the property that f 0(x) = p. Fenchel duality I The fenchel dual of a function f is f ∗(s) = sup < s; x > −f (x) x ∗∗ I If f is a closed convex function, then f = f . ∗ I f (0) = maxx (−f (x)) = − minx f (x) I rf ∗(s) = argmax < s; x > −f (x): x rf (x) = argmax < s; x > −f ∗(s): s ∗ ∗ I rf (rf (x)) = x and rf (rf (s)) = s Duality between strong convexity and Lipschitz gradients ∗ 1 I f is ρ strongly convex iff f has ρ -Lip. gradients ∗ ∗ 1 I f has L-Lip. gradients iff f is L strongly convex ∗ I If a non-differentiable funtion f can be identified as g we can 2 ∗ "smooth it out" by considering( g + δk · k2) which is 1 differentiable and has δ -Lip gradients. µ I δ = 2 below Section Introduction: Optimization with gradient methods Introduction:Machine Learning and Optimization Convex Analysis (Sub)Gradient Methods Online (sub)Gradient Methods (Sub)Gradient Methods I Gradient descent: wt+1 = wt − at gt , gt (sub)gradient of objective f at wt . I at can be decided by line search or by a predetermined scheme I If we are constrained to a convex set X , then use wt+1 = ΠX (wt − at gt ) = argmin kw − (wt − at gt )k2 w2X First and Second order information I First order information: rf (wt ); t = 0; 1; 2; :::; n 2 I Second order information is about r f and is expensive to compute and store for high dimensional problems. I Newton's method is a second order method where −1 wt+1 = wt − (Ht ) gt . I Quasi-Newton methods use first order information to try to approximate Newton's method. I Conjugate gradient also use first order information Lower Bounds I There are lower bounds for how well a first order method, i.e. a method for which wk+1 − wk 2Span(rf (x0); :::; rf (xk )) can do. They depend on the function class! I Given w0 ( and L, ρ), the bound is saying that for any > 0 there exists f in the class such that it takes at least n steps for any first order method and a statement about n is made. I Differentiable functions with Lipschitz continuous gradients: q 1 Lower bound is O( ) I Lipschitz continuous gradients and strong convexity: Lower 1 bound is O(log ). Gradient Descent with Lipschitz gradients I If we have L-Lipschitz continuous gradients, then define gradient decent by 1 w = w − rf (w ): k+1 k L k I Monotone: f (wk+1) ≤ f (wk ). I If f is also ρ-strongly convex (ρ > 0), then ρ (f (w ) − f (w ∗)) ≤ (1 − )k (f (w ) − f (w ∗)): k L 0 1 I This result translates into the optimal O(log ) rate for first order methods Gradient Descent without strong convexity I Suppose that we do not have strong convexity (ρ = 0) but we have L-LipG. 1 I Then the gradient descent procedure wk+1 = wk − L rf (wk ) 1 ∗ L ∗ 2 has rate O( ).(f (wk ) − f (w )) ≤ k kw − w0k . q 1 I The lower bound for this class is O( ). I There is a gap! I The question arose if the bound was loose or if there is a better first order method Nesterov's methods I Nesterov answered the question in 1983 by constructing an q 1 "Optimal Gradient Method" with the rate O( ) q L (O( ) if we have not fixed L).
Details
-
File Typepdf
-
Upload Time-
-
Content LanguagesEnglish
-
Upload UserAnonymous/Not logged-in
-
File Pages45 Page
-
File Size-