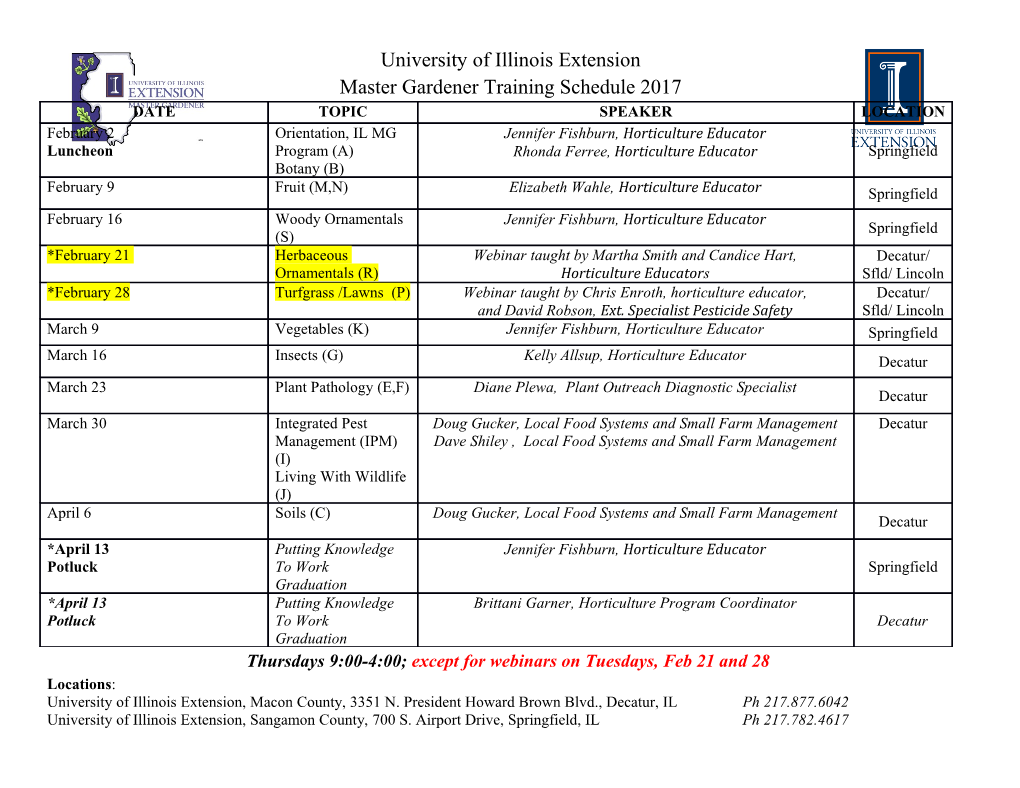
Linköping Studies in Science and Technology Dissertation No. 1851 Gated Bayesian Networks Marcus Bendtsen Linköping University Department of Computer and Information Science Division for Database and Information Techniques SE-581 85 Linköping, Sweden Linköping 2017 c Marcus Bendtsen, 2017 ISBN 978-91-7685-525-6 ISSN 0345-7524 URL http://urn.kb.se/resolve?urn=urn:nbn:se:liu:diva-136761 Published articles have been reprinted with permission from the respective copyright holder. Typeset using LATEX Printed by LiU-Tryck, Linköping 2017 ii Dedicated to my family – past, present and future – near and far. iii Gated Bayesian Networks ABSTRACT Bayesian networks have grown to become a dominant type of model within the domain of probabilistic graphical models. Not only do they empower users with a graphical means for describing the relationships among random variables, but they also allow for (potentially) fewer parameters to estimate, and enable more efficient inference. The random variables and the relation- ships among them decide the structure of the directed acyclic graph that repre- sents the Bayesian network. It is the stasis over time of these two components that we question in this thesis. By introducing a new type of probabilistic graphical model, which we call gated Bayesian networks, we allow for the variables that we include in our model, and the relationships among them, to change over time. We introduce algorithms that can learn gated Bayesian networks that use different variables at different times, required due to the process which we are modelling going through distinct phases. We evaluate the efficacy of these algorithms within the domain of algorithmic trading, showing how the learnt gated Bayesian networks can improve upon a passive approach to trading. We also intro- duce algorithms that detect changes in the relationships among the random variables, allowing us to create a model that consists of several Bayesian net- works, thereby revealing changes and the structure by which these changes occur. The resulting models can be used to detect the currently most appro- priate Bayesian network, and we show their use in real-world examples from both the domain of sports analytics and finance. v Populärvetenskaplig sammanfattning En grafisk modell är en beskrivning av hur olika fenomen står i relation till varandra. Ordet grafisk används för att förtydliga att denna beskrivning görs med hjälp av en graf, där fenomen är noder och relationer är bågar. Till ex- empel kan en grafisk modell beskriva hur olika levnadsvanor påverkar varan- dra, så som fysisk aktivitet, alkoholkonsumtion, matvanor och rökning. Den grafiska komponenten beskriver antaganden som leder till att beräkningar kring de fenomen som man mäter är genomförbara. Utan antaganden är det inte alltid möjligt att skapa en modell, då det kan kräva stora mängder insamlad data för att specificera modellen och beräkningarna kan ta orim- ligt lång tid. Felaktiga antaganden leder till att modellen presterar sämre, och idealet inträffar naturligtvis när antaganden man gjort sammanfaller med verkligheten. Verkligheten är dock ej konstant. Över tid kan det krävas att man byter fokus till andra fenomen än tidigare, eller så ändras relationerna mellan de fenomenen som man inkluderat i sin modell. Om vi då använder en mod- ell som inte har kapaciteten att byta fokus, eller ändra relationer över tid, så riskerar vi att få en underpresterande modell. I denna avhandling introducerar vi en ny grafisk modell som tar i beaktning det faktum att fokus och relationer förändras över tid. Vår nya grafiska modell, som vi kallar gated Bayesian networks, är en förlängning av den populära grafiska modellen Bayesian net- works. Modellen kombinerar flera olika Bayesian networks och kan välja mellan dessa för att upprätthålla prestandan. I denna avhandling introducerar vi algoritmer som tillåter oss att lära gated Bayesian networks från data. Algoritmerna varierar beroende på vad som är målet med inlärningen, samt vilka förutsättningar i form av data och kunskap man har innan själva inlärningen börjar. Till exempel visar vi hur vi kan lära oss modeller som automatiskt sköter aktiehandel på ett sådant sätt att vi minskar riskerna för förluster av det investerade kapitalet. Vi tillämpar även våra inlärningsalgoritmer för att identifiera de förändringar som sker över tid, specifikt när det gäller förändringar i volatilitet av finansiella mark- nader samt professionella atleters prestation. vii Acknowledgments Writing a thesis is an amazing adventure that cannot be fully appreciated until completed. However, the people that are key to your success, and the people who make the adventure a truly enjoyable endeavour, can definitely be appreciated from the start. I have been fortunate to have two fantastic supervisors that have helped me navigate the world of research and academia. To my supervisor Jose M. Peña: I cannot thank you enough for your support, guidance, patience and humour. You have allowed me independence, and I cannot remember a meeting where we did not break out laughing at least once. You have an admirable ability of seeing things for what they are, and I can only hope that some of this clarity has rubbed off on me. To my second supervisor Nahid Shahmehri: I will always be grateful that you were willing to give me a chance to prove myself, and your support has never wavered. You have given me invaluable advice throughout my years at ADIT, and I carry with me many memorable moments from working along your side. Throughout my years at IDA I have had the chance to work with many inspiring people, and while I wish to thank you all, I must extend a special thank you to Mariam Kamkar and Jalal Maleki. You both played a big part in me staying in academia, as you offered me a teaching position at an early stage and later facilitated my move to Ph.D. studies. You both see possibili- ties where others would see obstacles. I am also thankful for my colleagues at ADIT, with a special shout-out to Vengatanathan Krishnamoorthi, Zlatan Dragisic, Valentina Ivanova, and Patrick Lambrix for being loyal coffee and lunch mates. Conversing with you has often been the highlight of my day. Also a big thank you to Ulf Kargén and Niklas Carlsson for all their hard work in the courses we have given together. And then there is Dag Sonntag, my Ph.D. travelling companion and all-round good guy. I have so many won- derful memories from our travels together (often involving food), from the Christmas dinner mirage in Aalborg to incredible food in a restaurant with a somewhat dodgy entrance in Amsterdam. All I can say is that it has been a privilege that I will never forget. I have been fortunate to not only be part of a great working environment at IDA, but also the LIIR group at IMH. It has been invaluable to work in two different research areas in parallel, exposing me to two very different research cultures. I therefore thank Ulrika Müssener, Kristin Thomas, Nadine ix Karlsson, Catharina Linderoth, Matti Leijon and Jim McCambridge. I look forward to future collaborations with you all. I want to take this opportunity to extend a profound thank you to my family. To my extraordinary siblings Maria, Emma and Vilhelm: you are a source of rejuvenating energy, and I am looking forward to many more family activities throughout the coming years. It is also remarkable to see the next generation come to life through little Tyra. To my mother Hélène and father Preben: there are no words that can describe the support you both have given me, not only during the last few years, but throughout my life. You have cultivated in me a mindset of optimism, curiosity and gratefulness, all three of which I could never have made it this far without. You are, and have always been, my one fixed point in my otherwise sometimes impulsive lifestyle. I also wish to thank my grandparents, whose love and devotion is constantly strong, and views on life reminds me that age is just a number. Finally, from the bottom of my heart, I thank the love of my life Evelina Johansson. You are the strongest person I know, and you achieve your goals with great determination. I adore your kindness and positive attitude. Our life together is perfect, and I promise to always be there for you, as you are always there for me. Marcus Bendtsen May 2017 Linköping, Sweden x Contents 1 Introduction 1 1.1 Contributions . 4 1.2 Publications . 5 1.3 Disposition . 6 2 Background 7 2.1 Bayesian networks . 7 2.1.1 Reading independencies using d-separation . 9 2.1.2 Parameter estimation . 11 2.1.3 Structure learning . 13 2.1.4 Inference . 16 2.1.5 Summary . 20 2.2 Gated Bayesian networks . 21 2.2.1 Structural definitions . 22 2.2.2 Strategy encoding and decisions . 25 2.2.3 Execution of a gated Bayesian network . 26 2.2.4 Execution and modelling examples . 30 2.3 Related formalisms . 37 2.3.1 Influence diagrams and Markov decision processes . 37 2.3.2 Hidden Markov models . 39 2.3.3 Context specific independence . 40 2.3.4 Other formalisms . 41 2.4 Summary . 41 3 Learning gated Bayesian networks for algorithmic trading 43 3.1 Introduction to algorithmic trading . 43 3.1.1 Evaluating alpha models . 45 3.1.2 Benchmark . 49 3.1.3 Technical analysis . 49 3.2 Template based learning . 52 xiii 3.2.1 Gated Bayesian network template . 53 3.2.2 Multiple simulations . 54 3.2.3 Algorithm . 54 3.3 Learning gated Bayesian networks for trading stocks using template based learning . 56 3.3.1 Methodology . 56 3.3.2 Results and discussion .
Details
-
File Typepdf
-
Upload Time-
-
Content LanguagesEnglish
-
Upload UserAnonymous/Not logged-in
-
File Pages243 Page
-
File Size-