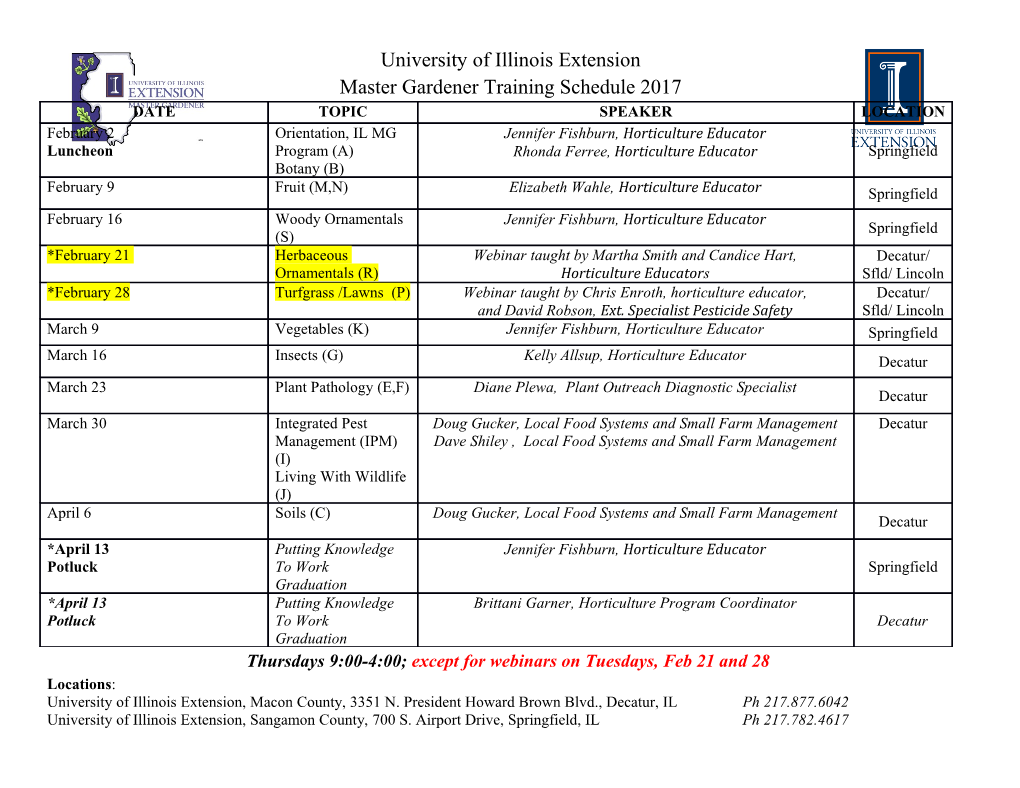
Dealing with Spelling Variation in Early Modern English Texts Alistair Baron B.Sc. (Hons) School of Computing and Communications Lancaster University A thesis submitted for the degree of Doctor of Philosophy February 2011 Abstract Early English Books Online contains digital facsimiles of virtually every English work printed between 1473 and 1700; some 125,000 publications. In September 2009, the Text Creation Partnership released the second instalment of transcrip- tions of the EEBO collection, bringing the total number of transcribed works to 25,000. It has been estimated that this transcribed portion contains 1 billion words of running text. With such large datasets and the increasing variety of historical corpora available from the Early Modern English period, the opportunities for historical corpus linguistic research have never been greater. However, it has been observed in prior research, and quantified on a large-scale for the first time in this thesis, that texts from this period contain significant amounts of spelling variation until the eventual standardisation of orthography in the 18th century. The problems caused by this historical spelling variation are the focus of this thesis. It will be shown that the high levels of spelling variation found have a significant impact on the accuracy of two widely used automatic corpus linguistic methods { Part-of-Speech annotation and key word analysis. The development of historical spelling normalisation methods which can alleviate these issues will then be presented. Methods will be based on techniques used in modern spellchecking, with various analyses of Early Modern English spelling variation dictating how the techniques are applied. With the methods combined into a single procedure, automatic normalisation can be performed on an entire corpus of any size. Evaluation of the normalisation performance shows that after training, 62% of required normalisations are made, with a precision rate of 95%. Declaration I declare that the work presented in this thesis is, to the best of my knowledge and belief, original and my own work. The material has not been submitted, either in whole or in part, for a degree at this, or any other university. Alistair Baron Acknowledgements Without the help of friends and family, it would have been impossible for me to even come close to completing this thesis. First and foremost, I'd like to thank my supervisor, Paul Rayson, who has been unbelievably supportive and patient throughout. Paul provided the spark I needed during my undergraduate project back in 2005 and gave me the confidence I needed to apply for the PhD process { he has put up with me and inspired me ever since. Many others have given a helping hand with my research and the writing of this thesis. In particular, I'd like to thank Dawn Archer who has provided invaluable advice at all stages of my PhD. Many people at Infolab21 have made it such a brilliant place to work, special thanks to Eddie Bell, Yehia El-khatib, John Mariani, Andy Molineux, Chris Paice, Nick Smith and Gaz Tyson for their help, as well as Awais, James and Phil for their patience during the write-up! I'd also like to thank all of the people who have taken the time to try out the VARD 2 software, your feedback has been invaluable. Just as important, I'd like to thank all those who have supported me at home: My parents, brothers, sisters, future in-laws and other family members for their love and support { particularly Judith for taking the time to read my thesis and provide brilliant feedback { and to all of my friends for the fun times when I've needed a break. Leaving the most important until last, with me every step of the way has been Mel, my wonderful fiance´e.Good things come to those who wait { I've finished now, so we can get married! Publications The following have been published as part of the research presented in this thesis. Where appropriate, portions of this thesis are based on my contributions to these publications without citation. Where research and text should be credited to a co-author, rather than myself, the work has been cited accordingly. Baron, A. (2006). Standardising Spelling in Early Modern English Texts. Poster at the Faculty of Science and Technology Research Conference, Lancaster University, UK, 20th December 2006. Rayson, P., Archer, D., Baron, A. and Smith, N. (2007). Tagging historical corpora - the problem of spelling variation. In Proceedings of Digital Historical Corpora, Dagstuhl-Seminar 06491, International Conference and Research Center for Computer Science, Schloss Dagstuhl, Wadern, Germany, December 3rd-8th 2006. ISSN 1862-4405. Rayson, P., Archer, D., Baron, A., Culpeper, J. and Smith, N. (2007). Tagging the Bard: Evaluating the accuracy of a modern POS tagger on Early Modern English corpora. In Davies, M., Rayson, P., Hunston, S. and Danielsson, P. (eds.) Proceedings of the Corpus Linguistics Conference: CL2007, University of Birmingham, UK, 27-30 July 2007. Rayson, P., Archer, D., Baron, A. and Smith, N. (2008). Travelling Through Time with Corpus Annotation Software. In Lewandowska-Tomaszczyk, B. (ed.) Corpus Linguistics, Computer Tools, and Applications State of the Art: PALC 2007. Peter Lang, Frankfurt am Main. Baron, A. and Rayson, P. (2008). VARD 2: A tool for dealing with spelling variation in historical corpora. Proceedings of the Postgraduate Conference in Corpus Linguistics, Aston University, Birmingham, 22 May 2008. Baron, A., Rayson, P. and Archer, D. (2009). The extent of spelling variation in Early Modern English. Presented at ICAME 30, Lancaster University, UK, 27-31 May 2009. Baron, A., Rayson, P. and Archer, D. (2009). Automatic Standardization of Spelling for Historical Text Mining. In Proceedings of Digital Humanities 2009, University of Maryland, USA, 22-25 June 2009, pp. 309{312 Baron, A. and Rayson, P. (2009). Automatic standardization of texts containing spelling variation, how much training data do you need? In Mahlberg, M., Gonzlez-Daz, V. and Smith, C. (eds.) Proceedings of the Corpus Linguistics Conference, CL2009, University of Liverpool, UK, 20-23 July 2009. Baron, A., Rayson, P. and Archer, D. (2009). Word frequency and key word statistics in historical corpus linguistics. Anglistik: International Journal of English Studies, 20 (1), pp. 41{67. Lehto, A., Baron, A., Ratia, M. and Rayson, P. (2010). Improving the precision of corpus methods: The standardized version of Early Modern English Medical Texts. In Taavitsainen, I. and Pahta, P. (eds.) Early Modern English Medical Texts: Corpus description and studies, pp. 279{290. John Benjamins, Amsterdam. Contents List of Figures ix List of Tables xi 1 Introduction 1 1.1 Problem Overview . .1 1.2 Research Questions . .4 1.3 Structure of Thesis . .6 2 Background and Related Work 8 2.1 Early Modern English . .9 2.1.1 History . .9 2.1.2 Spelling Variation . 11 2.1.3 Corpus Linguistics and Early Modern English . 14 2.2 Spelling Error Detection and Correction . 21 2.2.1 Spelling Error Tools and Applications . 21 2.2.2 Non-word Error Detection . 24 2.2.3 Isolated Error Correction . 26 2.2.4 Context Sensitive Error Detection and Correction . 32 2.2.5 Summary . 36 2.3 Specific Research Dealing with Historical Spelling Variation . 38 2.3.1 Studies Concerning English . 38 2.3.2 Studies Concerning Other Languages . 43 2.4 Chapter Summary . 47 vi CONTENTS 3 Analysis of Early Modern English Spelling Variation 49 3.1 Levels of Spelling Variation in Early Modern English . 50 3.2 Effect on Corpus Linguistics . 58 3.2.1 Part-of-Speech Annotation . 58 3.2.2 Key Word Analysis . 61 3.3 Spelling Variation Characteristics . 67 3.3.1 Real-Word Spelling Variants . 68 3.3.2 Character Level Variation . 71 3.4 Chapter Summary . 82 4 Normalising Early Modern English Spelling Variation 85 4.1 Spelling Variant Detection . 86 4.1.1 Dictionary Source . 86 4.1.2 Dictionary Lookup . 89 4.1.3 Tokenization . 93 4.2 Spelling Variant Normalisation . 94 4.2.1 Producing a List of Candidates . 95 4.2.2 Ranking Candidates . 109 4.2.3 Improvement Through Training . 120 4.2.4 Summary of Normalisation Procedure . 126 4.3 VARD 2 . 128 4.3.1 Interactive Processing . 129 4.3.2 Automatic Processing . 131 4.3.3 Training and Customisation . 133 4.4 DICER . 134 4.5 Chapter Summary . 140 5 Evaluation of Spelling Variant Normalisation 142 5.1 Spelling Variant Detection . 143 5.2 Automatic Normalisation . 148 5.2.1 Training . 149 5.2.2 Normalisation Threshold . 154 5.3 Normalising the Early Modern English Medical Text Corpus . 160 5.3.1 Training Through Manual Normalisation . 161 5.3.2 Initial Results and Analysis . 162 vii CONTENTS 5.3.3 Selecting a Normalisation Threshold . 166 5.3.4 Summary and Results of Final Automatic Normalisation . 168 5.4 Chapter Summary . 170 6 Conclusions 173 6.1 Summary of Work . 173 6.2 Research Questions Revisited . 179 6.3 Contributions . 181 6.4 Future Work . 183 References 186 viii List of Figures 3.1 Graph showing variant types % in all corpora over time. 54 3.2 Graph showing variant tokens % in all corpora over time. 54 3.3 Average variant percentage over corpora available for each decade. 55 3.4 Comparison of variant counts in EEBO corpus samples with (=original) and without initial capital words. 57 3.5 Graphs showing the rank correlation coefficients comparing EEBO decade samples' key word lists before and after normalisation. 66 3.6 Graphs showing the frequency of spelling variants in the EEBO samples before and after automatic normalisation. 67 4.1 Trie example. 90 4.2 Screenshot of interactive processing mode of VARD 2. 130 4.3 Screenshot of batch processing mode of VARD 2.
Details
-
File Typepdf
-
Upload Time-
-
Content LanguagesEnglish
-
Upload UserAnonymous/Not logged-in
-
File Pages219 Page
-
File Size-