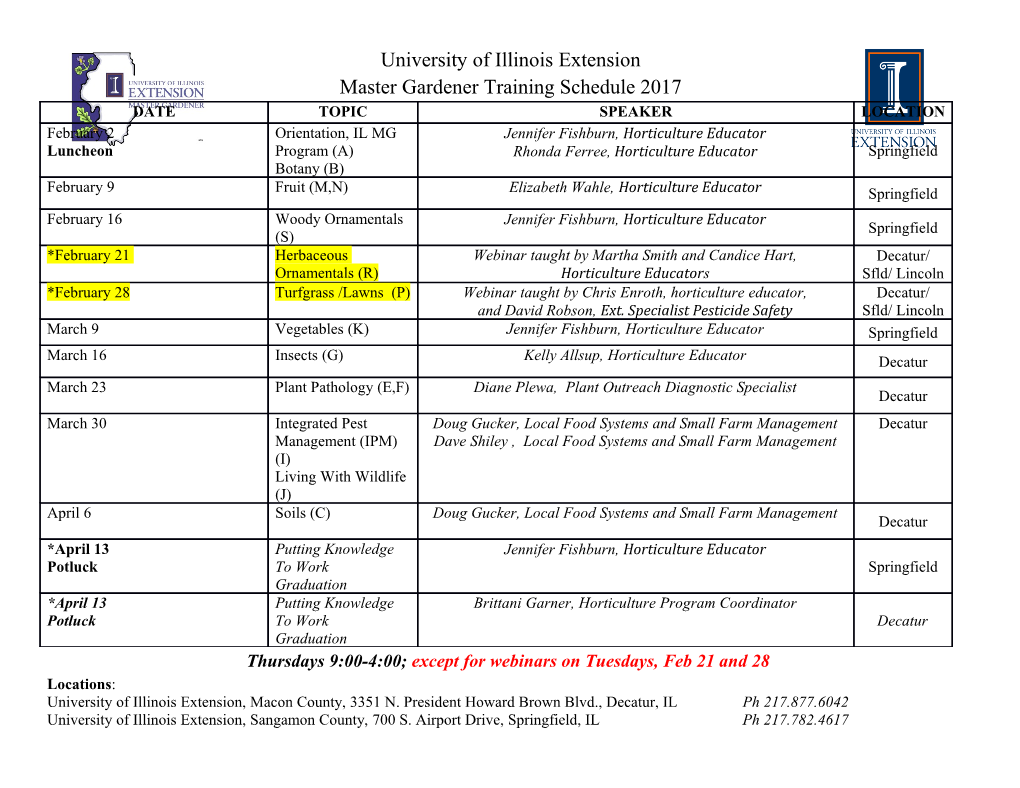
QUANTUM NEURAL NETWORKS A Dissertation by Nam H. Nguyen Master of Science, Wichita State University, 2016 Bachelor of Arts, Eastern Oregon University, 2014 Submitted to the Department of Mathematics, Statistics, and Physics and the faculty of the Graduate School of Wichita State University in partial fulfillment of the requirements for the degree of Doctor of Philosophy May 2020 © Copyright 2020 by Nam H. Nguyen All Rights Reserved QUANTUM NEURAL NETWORKS The following faculty members have examined the final copy of this dissertation for form and content, and recommend that it be accepted in partial fulfillment of the requirement for the degree of Doctor of Philosophy with a major in Applied Mathematics. Elizabeth Behrman, Committee Chair James Steck, Committee Member Buma Fridman, Committee Member Ziqi Sun, Committee Member Terrance Figy, Committee Member Accepted for the College of Liberal Arts and Sciences Andrew Hippisley, Dean Accepted for the Graduate School Coleen Pugh, Dean iii DEDICATION To my late grandmother Phan Thi Suu , and my loving mother Nguyen Thi Kim Hoa iv ACKNOWLEDGEMENTS First and foremost, I would like to express my profound gratitude to my advisor, Dr. Elizabeth Behrman, for her advice, support and guidance toward my Ph.D. degree. She taught me not only the way to do scientific research, but also the way to become a profes- sional scientist and mathematician. Her endless encouragements and patient throughout my graduate studied have positively impacted my life. She would always allowed me to work on different research areas and ideas even if it's outside the scope of my dissertation. This allows me to learn and investigate problems in many different areas of mathematics, which have benefited me greatly. Her scientific vigor and dedication makes her a lifetime role model for me. I will forever be indebted to her. I also would like to extend my gratitude to Dr. James Steck for his guidance through- out this journey. I have gained a tremendous amount of knowledge in neural networks in both theory and applications as whole from working with him for the past four years. I also would like to express my deepest gratitude to Dr. Buma Fridman for spending time to teach me many important concepts in mathematics. I am especially indebted to him for spending the summer of 2017 to worked with me, helped me to build a better under- standing of the Kolmogorov's superposition theorem and all its extension results including a result of his own. Our various discussions through the years have helped me become a better mathematician than I would have been. I also would like to pay my special regards to Dr. Ziqi Sun and Dr. Terrance Figy for their willingness to be on my dissertation committee. Furthermore, I want to acknowledge v Dr. Ziqi Sun for his countless encouragements throughout my Ph.D studied and how much he helped to understand functional analysis better; seeing his passion and curiosity for math- ematics and physics makes me to always strive to be a better scientist and mathematician. I am also deeply indebted to Professor Edward Behrman of The Ohio State University for his financial support during my graduate studied through the Behrman's Family Foun- dation. Because of his generosity, I was able to have a smaller teaching load and dedicated much more time in to my research and studied. Furthermore, I would like to acknowledge and thank all my colleagues and friends (Nathan, Bill, Henry, Saideep, Mo), with whom I collaborated on most of my research work. This work would not be possible without all their helps. They all have made great mean- ingful impact in my life. Especially, I want to give a special acknowledge to Dr. Tianshi Lu and Sirvan Rhamati (my best friend at WSU) for there willingness to discuss and work with me on various problems/ideas during my time at WSU; from number theory to graph theory to fractional derivatives to probability theory to math competition problems, even. They shared with me many great ideas throughout the years. Their constant questionings, ways of thinking, and supports have positively influenced me with great impact. It has been a joy to have a true friend like Sirvan who I can share my problems as well as happiness with. Most of all, I would like to thank my dear mother and my late grandmother. They have supported me throughout my academic journey and always motivated me to strive forward. Their unconditional love has never been affected by the physical distance between us. This dissertation is dedicated to them. vi ABSTRACT Quantum computing is becoming a reality, at least on a small scale. However, de- signing a good quantum algorithm is still a challenging task. This has been a huge major bottleneck in quantum computation for years. In this work, we will show that it is possible to take a detour from the conventional programming approach by incorporating machine learn- ing techniques, specifically neural networks, to train a quantum system such that the desired algorithm is \learned," thus obviating the program design obstacle. Our work here merges quantum computing and neural networks to form what we call \Quantum Neural Networks" (QNNs). Another serious issue one needs to overcome when doing anything quantum is the problem of \noise and decoherence". A well-known technique to overcome this issue is using error correcting code. However, error correction schemes require an enormous amount of additional ancilla qubits, which is not feasible for the current state-of-the-art quantum computing devices or any near-term devices for that matter. We show in this work that QNNs are robust to noise and decoherence, provide error suppression quantum algorithms. Furthermore, not only are our QNN models robust to noise and decoherehce, we show that they also possess an inherent speed-up, in term of being able to learned a task much faster, over various classical neural networks, at least on the set of problems we benchmarked them on. Afterward, we show that although our QNN model is designed to run on a fundamental level of a quantum system, we can also decompose it into a sequence of gates and implement it on current quantum hardware devices. We did this for a non-trivial problem known as the \entanglement witness" calculation. We then propose a couple of different hybrid quan- tum neural network architectures, networks with both quantum and classical information processing. We hope that this might increase the capability over previous QNN models in terms of the complexity of the problems it might be able to solve. vii TABLE OF CONTENTS Chapter Page 1 INTRODUCTION...................................1 1.1 Motivation.....................................1 1.2 Scope of this Dissertation............................2 1.3 Literature Review.................................4 1.4 Contributions...................................8 1.5 Structure of this Dissertation..........................8 2 OVERVIEW OF QUANTUM MECHANICS..................... 10 2.1 Quantum States and Density Operators.................... 11 2.2 Composite Systems and Entanglement..................... 13 2.3 Time-Evolution of a Closed System....................... 20 2.4 Quantum Measurements............................. 22 3 QUANTUM COMPUTING.............................. 26 3.1 Qubits....................................... 27 3.2 Quantum Gates and Circuits Model....................... 33 3.3 Universal Quantum Computation and The Solovay-Kitaev Theorem..... 40 3.4 Quantum Speed-up and Quantum Algorithms................. 43 3.5 Adiabatic Quantum Computation Model.................... 46 3.6 Quantum Decoherence, Noise, and Error Correction.............. 49 4 CLASSICAL ARTIFICIAL NEURAL NETWORKS................ 53 4.1 Introduction.................................... 54 4.2 Artificial Neurons................................. 55 4.2.1 Perceptron................................. 57 4.2.2 Sigmoid Neuron.............................. 59 4.3 Multi-Layer Neural Networks.......................... 60 4.3.1 Networks Architecture.......................... 61 4.3.2 Error Backpropagation and The Gradient Descent Learning Rule.. 62 4.4 Universal Approximation............................. 66 4.4.1 Approximation with Boxes........................ 67 4.4.2 Approximation with Modern Analysis.................. 74 5 QUANTUM NEURAL NETWORK.......................... 77 5.1 Fundamental Structure.............................. 78 5.2 Learning Algorithm................................ 81 5.3 Simulation of Classical and Quantum Logic Gates, and Quantum Circuit.. 84 5.4 Universal Property of Quantum Neural Network................ 89 5.5 An Alternative Learning Approach....................... 92 viii TABLE OF CONTENTS (continued) Chapter Page 6 ROBUSTNESS OF QUANTUM NEURAL NETWORK.............. 95 6.1 Quantum Computing in The Classical World.................. 96 6.2 Dealing with Noise and Decoherence...................... 97 6.3 Entanglement Calculation For Two-Qubit System............... 98 6.3.1 Learning with noise............................ 105 6.3.2 Learning with Decoherence........................ 114 6.3.3 Learning with Noise plus decoherence.................. 121 6.4 Entanglement Calculation on Higher-Order Qubit Systems.......... 128 6.4.1 Results for the Three-Qubit System: Training and Testing...... 128 6.4.2 Results for the four- and five-qubit systems: Training and Testing.. 138 6.4.3 Quantifying the improvement in robustness with increasing size of the system................................... 139 6.4.4 Learning with other types of noise...................
Details
-
File Typepdf
-
Upload Time-
-
Content LanguagesEnglish
-
Upload UserAnonymous/Not logged-in
-
File Pages256 Page
-
File Size-