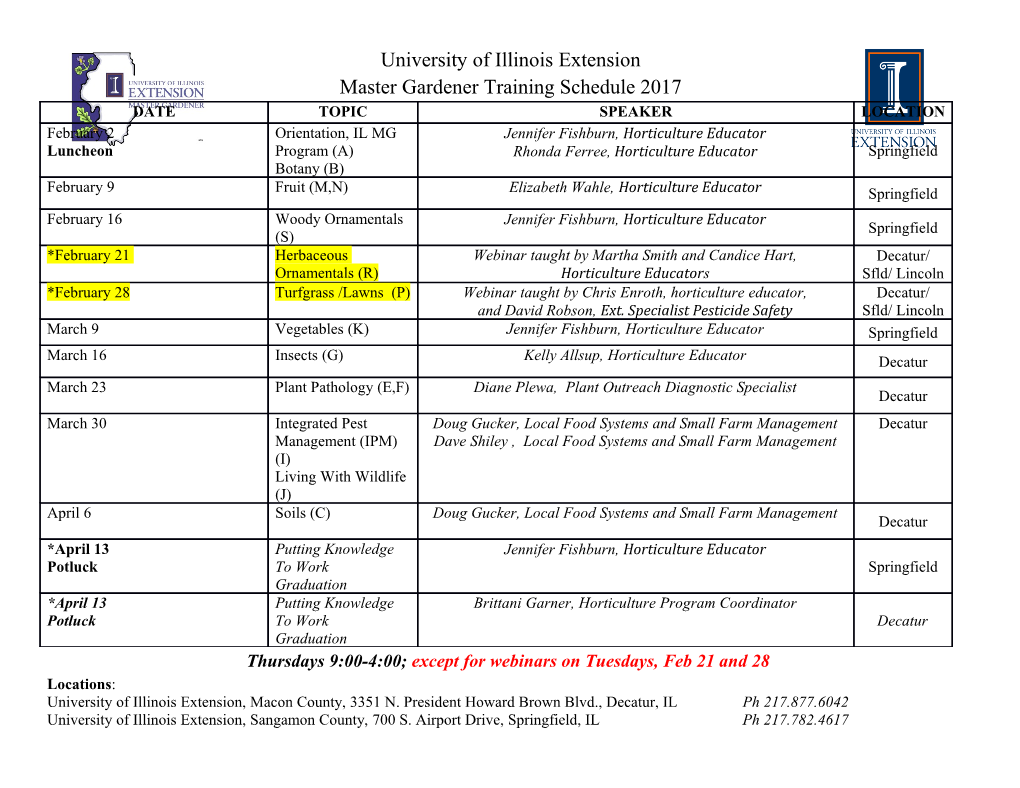
Decentralised Detection of Emergence in Complex Adaptive Systems Eamonn O'Toole A Dissertation submitted to the University of Dublin, Trinity College in fulfillment of the requirements for the degree of Doctor of Philosophy (Computer Science) November 2015 Declaration I declare that this thesis has not been submitted as an exercise for a degree at this or any other university and it is entirely my own work. I agree to deposit this thesis in the University's open access institutional repository or allow the library to do so on my behalf, subject to Irish Copyright Legislation and Trinity College Library conditions of use and acknowledgement. Eamonn O'Toole Dated: November 2, 2015 Acknowledgements The African proverb states that \it takes a village to raise a child" and the same sentiment certainly applies to my career as a researcher. First, and foremost, I want to thank my supervisor, Prof. Siobh´anClarke for offering me support, expertise, encouragement and good humour during the process of my Ph.D. studies. I also owe an huge debt of gratitude to Vivek Nallur, for his enthusiasm, guidance and willingness to discuss any topic at the drop of a hat. A big thank you to Julien Monteil for his advice on matters statistical and signal-filtering, as well as his thoughts on the dynamics of traffic-flow. Thank you to everybody in DSG, especially those of you who shared the office with me over the years. A very special thank you to my colleagues Saeed Hajebi and Amit Raj, for joining me on the both the Ph.D. and TRANSFoRm adventures, providing somebody to talk to about both. I'd also like to thank my friends away from Trinity, especially Simon Tuohy, Will O’Keeffe, Niall Curtin and Declan Bourke, for encouraging me and reminding me that there is more to life than academia. Thank you to my entire family, especially to my parents who have supported and encouraged my education from the very beginning. I dedicate this thesis to them. Finally, Sinead, you have been my rock throughout the past 5 years. Thank you for your patience, love and constant support. This would not have been possible without you. Eamonn O'Toole University of Dublin, Trinity College November 2015 iii Abstract Emergence is a hallmark of Complex Adaptive Systems (CAS), where non-deterministic inter- actions between agents can give rise to emergent behaviour or properties at the system level. The nature, timing and consequence of emergent behaviour are fundamentally unpredictable and may be harmful to the system or individual agents. This unpredictability, coupled with the decentralised structure of these systems, means that detecting emergence at run time presents a significant challenge. No single component of a decentralised system can possess a global view of system state, or reliably know in advance the relevant system properties that indicate emergence. Existing approaches to emergence detection have used statistical analysis of system variables that represent global features of the system. These techniques depend on a centralised system monitor with access to information on global system state. However, neither of these assumptions are met in CAS, as these are distributed and composed entirely of decentralized autonomous agents. Additionally, these approaches require prior knowledge of the system properties relevant for emergence detection, which may not be obvious or available for all systems. Other approaches use formal methods to define and predict emergence, but are intended for use at design time or are limited to well-defined, closed systems. This thesis presents Decentralised Emergence Detection (DETect), a novel distributed algo- rithm that enables constituent agents to collaboratively detect emergent events in CAS. The main contribution of DETect is that it does not require any centralised controller or system monitor, and instead runs locally on each agent. In addition, DETect uses only the available informa- tion from an agent's local environment at run time to facilitate detection. DETect relies on the feedback that occurs from the system level (macro) to the agent level (micro) when emergence is present. This feedback constrains agents at the micro-level, and results in changes occurring in the relationship between an agent and its environment. DETect uses statistical methods to iv automatically select the properties of the agent and environment to monitor, and tracks the relationship between these properties over time for changes. When a significant change is de- tected, the algorithm uses distributed consensus to determine if a sufficient number of agents have simultaneously experienced a similar change, before a shared conclusion is reached that emergence has occurred. On agreement of emergence, DETect raises an event, which its agent or other interested observers can use to act appropriately. The evaluation of DETect uses three multi-agent simulation case studies: flocking, pedestrian counter-flow and traffic. Each simulation model exhibits emergence allowing performance to be assessed across a range of system scales. The case studies are structured to verify the efficacy of DETect in three competences: autonomously selecting what properties to monitor, detecting feedback from emergence and forming consensus among agents on the presence of emergence. Performance is evaluated for detection of both the formation and evaporation of emergence in each system. The results of these studies demonstrate that DETect generally achieves its design objectives, facilitating the decentralised detection of emergence formation in each case study. However, the general applicability of DETect during periods of emergence evaporation is limited, with successful detection achieved in the pedestrian and traffic case studies only. v Publications Related to this Ph.D. 1. O'Toole, Eamonn, and Siobh´anClarke.\Dynamic forecasting and adaptation for demand optimization in the Smart Grid" Proceedings of the First International Workshop on Soft- ware Engineering Challenges for the Smart Grid. IEEE Press, 2012. 2. O'Toole, Eamonn, Vivek Nallur, and Siobh´anClarke.\Towards Decentralised Detection of Emergence in Complex Adaptive Systems" Self-Adaptive and Self-Organizing Systems (SASO), 2014 IEEE Eighth International Conference on. IEEE, 2014. 3. O'Toole, Eamonn, Vivek Nallur, and Siobh´anClarke.\Decentralised Detection of Emer- gence in Complex Adaptive Systems" submitted to ACM Transactions on Autonomous and Adaptive Systems (TAAS) (submitted September 2015). vi Contents Acknowledgements iii Abstract iii List of Tables xi List of Figures xiii Chapter 1 Introduction 1 1.1 Motivation . .1 1.2 Emergence in CAS . .2 1.3 Challenges . .4 1.3.1 Existing Solutions . .6 1.4 Thesis Approach . .6 1.5 Thesis Contribution . .9 1.6 Thesis Structure . 10 1.7 Chapter Summary . 11 Chapter 2 Background and Related Work 12 2.1 Emergence . 12 2.1.1 Characteristics of Emergence . 12 2.1.2 Studying Emergence . 15 2.1.3 Types of Emergence . 17 2.1.4 Emergence and Self-Organisation . 21 2.1.5 Summary . 22 vii 2.2 Complex Adaptive Systems . 23 2.2.1 Introduction . 23 2.2.2 Modelling CAS . 25 2.2.3 Multi-Agent Systems . 29 2.2.4 Summary . 32 2.3 Detecting Emergence . 33 2.3.1 Variable based approaches . 33 2.3.2 Formal approaches . 38 2.3.3 Event-based approaches . 42 2.3.4 Analysis . 43 2.4 Summary . 44 Chapter 3 Design 46 3.1 Design Objectives . 46 3.2 System Model . 49 3.2.1 Terminology . 50 3.2.2 Emergence . 51 3.2.3 Assumptions . 51 3.3 Design Decisions . 52 3.3.1 Decentralised Detection . 52 3.3.2 Detection in the context of adaptation . 53 3.3.3 Detecting Feedback From Emergence . 54 3.3.4 Consensus Formation . 61 3.4 Proposed Solution . 64 3.4.1 Modelling Unit . 65 3.4.2 Change Detection Unit . 72 3.4.3 Collaboration Unit . 75 3.4.4 DETect and Design Objectives . 77 3.5 Limitations Due to Design Decisions . 78 3.6 Summary . 80 viii Chapter 4 Implementation and Simulation Environment 81 4.1 Simulation Framework . 81 4.2 Generic DETect Agent Implementation . 86 4.2.1 Agent Initialisation . 86 4.2.2 Generic Time Step . 87 4.3 Model 1: Flocking . 89 4.3.1 Behavioural Rules . 90 4.3.2 DETect Integration . 91 4.3.3 Monitoring Emergence Objectively . 93 4.4 Model 2: Pedestrian Counter Flow . 94 4.4.1 Behavioural Rules . 95 4.4.2 DETect Integration . 96 4.4.3 Monitoring Emergence Objectively . 96 4.5 Model 3: Traffic . 98 4.5.1 Street Network and Navigation . 99 4.5.2 Behavioural Rules . 100 4.5.3 DETect Integration . 101 4.5.4 Monitoring Emergence Objectively . 103 4.5.5 Summary . 104 Chapter 5 Evaluation 106 5.1 Experimental Setup . 107 5.1.1 General Setup . 107 5.1.2 Stage 1: Model Selection . 109 5.1.3 Stage 2: Feedback Detection . 110 5.1.4 Stage 3: Consensus Formation . 111 5.2 Evaluation Baselines . 113 5.3 Results and Analysis . 116 5.3.1 Model Selection . 116 5.3.2 Feedback Detection . 117 5.3.3 Consensus Formation . 123 5.4 Discussion . 136 ix 5.4.1 Model Selection . 136 5.4.2 Feedback Detection . ..
Details
-
File Typepdf
-
Upload Time-
-
Content LanguagesEnglish
-
Upload UserAnonymous/Not logged-in
-
File Pages203 Page
-
File Size-