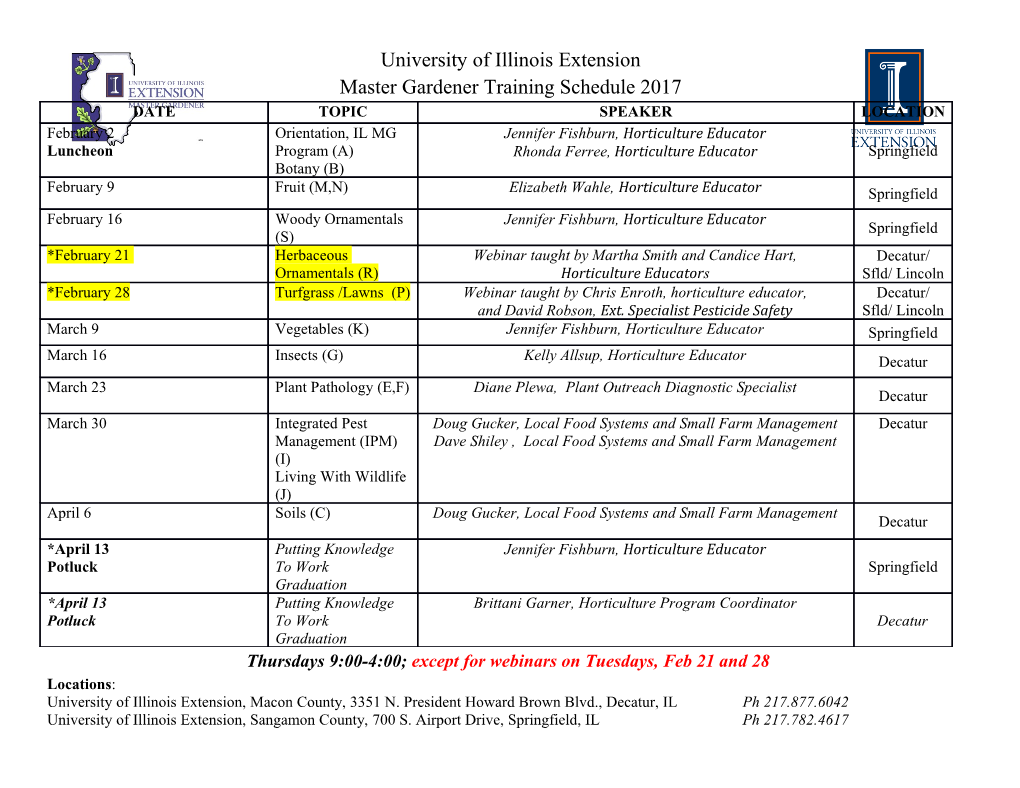
A QUANTITATIVE STUDY ON BEV AND PHEV ADOPTION IN THE NETHERLANDS Brenda Janssen Student number: 358527 MSc Business Information Management 2015/2016 June 16th 2016 Coach: Micha Kahlen, MSc Co-reader: Dr. Ksenia Koroleva Brenda Janssen – June 16th 2016 The author declares that the content presented in this master thesis is original and that no sources other than those mentioned in the text and its references have been used in creating the master thesis. The copyright of this master thesis rests with the author. The author is responsible for its contents. Rotterdam School of Management, Erasmus University, is only responsible for the educational coaching and cannot be held liable for the content. 1 Brenda Janssen – June 16th 2016 Acknowledgements First and foremost, I would like to thank my coach Micha Kahlen for his advice, guidance and specially his quick and honest feedback throughout the thesis process. Secondly, I would like to thank my co-reader Ksenia Koroleva for her time and feedback on, among others, the statistical challenges I had to tackle. Also, I would like to thank my Stedin coaches Baerte de Brey and Henk Fidder for their time, their feedback, for sharing their knowledge and their challenging questions which kept me focused. Furthermore, I would like to thank Harm-Jan Idema for his time, ideas and insights in the ever changing world of electric vehicles. I am grateful to my parents for their unconditional support during the past years. Finally, special thanks go to Sander who has supported me throughout the thesis trajectory and was always willing to be my sparring partner and motivator. Brenda Davidse-Janssen June 16th 2016 2 Brenda Janssen – June 16th 2016 Abstract Purpose: Over the past two years the amount of registered plug-in hybrid (PHEV) and battery electric vehicles (BEV) in The Netherlands has more than doubled, inter alia, due to subsidies and fiscal benefits provided by the Dutch government. However, it is still rather uncertain which other factors, apart from financial incentives, drive (PH)EV adoption. Besides, what role does the degree of urbanization play? DSOs need an estimate on how the corresponding demand for electricity will develop, in order to prepare for, for example grid infrastructure. On the other hand, governments need insights in the measures that should be taken in order to reach the aim of an emission free Dutch fleet by 2050. Furthermore, cities that face severe air pollution need insights in how to decrease pollution as effectively as possible. Thus, this study will answer the following research question: What demographical, municipal and context factors influence the adoption of (Plug-in Hybrid) Electric Vehicles for very highly urbanized to non-urban municipalities? Methods: Adoption likelihood for plug-in hybrid and battery electric vehicles is analyzed based on demographic, municipal, context and urban drivers. The multiple regression model is based on factors such as age, standardized spendable household income, the number of persons per household and the number of cars per household, the ability to request a charging pole, the number of public charging poles and the degree of urbanization. Whereas data on demographic, context and urban drivers has been obtained from the Centraal Bureau voor de Statistiek, the municipal data has been made available through APPM. The purchase likelihood, measured by the number of BEVs and PHEVs out of the total number of passenger cars per municipality, is estimated for the short-term. Finally, this estimation will be applied to the municipality of Rotterdam in order to forecast both BEV and PHEV adoption until 2020. Findings: For BEV adoption, standardized spendable household income is the most important factor (β=0.310), followed by the relative presence of 40 to 50 year olds (β=0.124). The number of public charging poles is an important influencing factor as well (β=0.339), nevertheless its relation is somewhat ambiguous as it represents an endogeneity problem. Standardized spendable household income is an even more important factor for the purchase likelihood of plug-in hybrid vehicles (β=0.3459), followed by the number of cars per household (β=0.328), income from an own enterprise (β=0.196), and the relative presence of 50 to 60 year olds (β=-0.195). The difference in the importance of household income is likely caused by the relatively higher purchase price of PHEVs. While the degree of urbanization is not found significant for BEV adoption, it appears to have a positive association with PHEV adoption (β=0.275). Furthermore, for highly urban municipalities, the number of cars per household is an important factor for both BEV and PHEV adoption. The number of persons per household is found to be positively related to BEV adoption for moderately and little urban municipalities. Implications: Local governments can predict based on the above described findings which neighborhoods will most likely see the highest BEV and PHEV adoption rates. This could give insights into the level of air quality or could clarify which inhabitants and neighborhoods might require additional incentives to stimulate (PH)EV purchase likelihood. For DSOs these findings can assist in the prioritization of investments related to infrastructure upgrades: neighborhoods with relatively higher incomes are more likely to adopt a BEV or PHEV and thus might demand a higher number of charging poles. Car manufacturers can use the 3 Brenda Janssen – June 16th 2016 findings of this study to target the right consumer segment and look ahead for other segments that might be interested in cheaper or more advanced versions. Finally, this study gives insights in how (PH)EV adoption will develop, ceteris paribus, and what additional incentives or technological improvements might be needed in order to achieve acceptable adoption rates. In terms of theoretical implications, future research should continue to remain BEV and PHEV adoption separated and continue to take the degree of urbanization into account. Limitations: The focus of this study is on demographic, municipal, context and urban factors influencing the purchase likelihood of BEVs and PHEVs. Consequently, the scope of this research is limited and cannot aim to cover the full range of predictive factors. Future research could incorporate the effects of the changing fiscal and financial benefits, qualitative factors such as range anxiety or charging behavior and fast charging infrastructure. Finally, the forecasts for the municipality of Rotterdam have been based on assumptions due to the absence of growth figures. Keywords: Battery Electric Vehicle, Charging Infrastructure, Context Drivers, Degree of Urbanization, Demographic Drivers, Distribution System Operator, Forecast 2020, Non-financial Municipal Incentives, Plug-in Hybrid Vehicle 4 Brenda Janssen – June 16th 2016 Table of content Acknowledgements ....................................................................................................................................... 2 Abstract ......................................................................................................................................................... 3 Introduction ................................................................................................................................................... 7 Hypothesis Development ............................................................................................................................ 10 2.1 Demographic drivers ......................................................................................................................... 10 2.2 Municipal drivers ............................................................................................................................... 11 2.3 Context drivers .................................................................................................................................. 12 2.4 Degree of urbanization ...................................................................................................................... 13 Research Design .......................................................................................................................................... 15 3.1 Methodology ..................................................................................................................................... 15 3.2 Sample & data ................................................................................................................................... 16 3.2.1 Sample ........................................................................................................................................ 16 3.2.2 Dependent variable: percentage battery electric and plug-in hybrid vehicles .......................... 16 3.2.3 Independent variables: demographic, municipal, context and urban drivers ........................... 18 Results ......................................................................................................................................................... 21 4.1 Descriptives ....................................................................................................................................... 21 4.2 Estimating BEV adoption ................................................................................................................... 22 4.2.1 Estimating demographic drivers on BEV adoption ..................................................................... 22 4.2.2 Municipal
Details
-
File Typepdf
-
Upload Time-
-
Content LanguagesEnglish
-
Upload UserAnonymous/Not logged-in
-
File Pages75 Page
-
File Size-