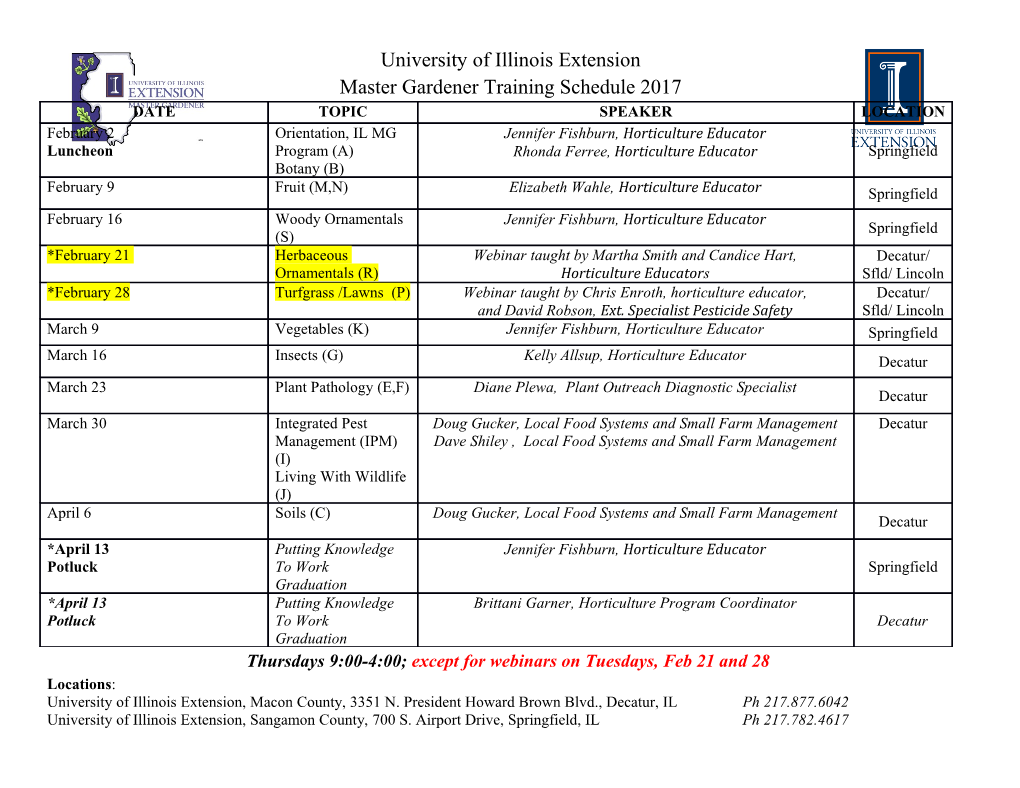
The language of causation Ari Beller*, Erin Bennett* & Tobias Gerstenberg fabeller, erindb, [email protected] Department of Psychology, Stanford University 450 Jane Stanford Way, Stanford, CA, USA, 94305 * equal contribution Abstract these accounts so far have in common is that they construe causal relationships in terms of some notion of probabilistic, People use varied language to express their causal understand- ing of the world. But how does that language map onto peo- counterfactual, or logical dependence. ple’s underlying representations, and how do people choose Wolff (2007) developed a different framework for think- between competing ways to best describe what happened? In this paper we develop a model that integrates computational ing about causal expressions such as “cause”, “enable”, “de- tools for causal judgment and pragmatic inference to address spite”, and “prevent” that is based on Talmy’s (1988) theory these questions. The model has three components: a causal of force dynamics (see also Wolff, Barbey, & Hausknecht, inference component which computes counterfactual simula- tions that capture whether and how a candidate cause made 2010). According to the force dynamics model, causal ex- a difference to the outcome, a literal semantics that maps pressions map onto configurations of force vectors (Figure 1). the outcome of these counterfactual simulations onto different For example, the causal construction “A caused P to reach E” causal expressions (such as “caused”, “enabled”, “affected”, or “made no difference”), and a pragmatics component that maps onto a configuration in which P’s initial force didn’t considers how informative each causal expression would be point toward the endstate E, and A’s force combined with for figuring out what happened. We test our model in an ex- P’s such that the resulting force R led P to reach the end- periment that asks participants to select which expression best describes what happened in video clips depicting physical in- state. In contrast, the construction “A enabled P to reach E” teractions. implies that P’s force vector already pointed toward the end- Keywords: causality; language; counterfactuals; pragmatics; state, and A’s force combined with P’s such that it reached the intuitive physics. endstate. The force dynamics model accurately predicts par- ticipants’ modal selection of which expression best captures Introduction what happened in a variety of different video clips (Wolff, The words we use to describe what happened matter. Hearing 2007). However, the force dynamics model also has some that “Tom killed Bill” elicits a different mental model of what limitations. For example, it doesn’t yield quantitative pre- happened than hearing that “Tom caused Bill to die” does dictions about how well a particular expression captures what (Freitas, DeScioli, Nemirow, Massenkoff, & Pinker, 2017; happened, but instead relies on a qualitative mapping between Niemi, Hartshorne, Gerstenberg, Stanley, & Young, 2020; situations and causal expressions. Rather than a distribution Thomson, 1976). The question of how we express our knowl- over response options, it predicts a single response. Also, edge of what happened in words, and how we infer what hap- as we will see below, when people are provided with other pened based on the words we hear, has a long and deep tradi- alternative expressions (such as “affected”, or “made no dif- tion in philosophy, linguistics, and cognitive science (Pinker, ference”) they don’t choose “enabled” for the predicted force 2007). configuration. In cognitive science, most work on causal cognition has fo- In this paper, we propose a new model of the meaning and cused on building models that capture the notions of “cause” use of causal expressions. Our model builds on the counter- and “prevent” (Waldmann, 2017). However, some attempts factual simulation model (CSM) of causal judgment devel- have also been made to uncover the differences between oped by Gerstenberg, Goodman, Lagnado, and Tenenbaum causal verbs such as “cause” and “enable”. For example, (2015, 2020). Gerstenberg et al. tested their model on dy- Cheng and Novick (1991) argue that these verbs map onto namic physical interactions similar to the diagrams shown at different patterns of co-variation. Sloman, Barbey, and Ho- the top of Figure 2. In their experiment, participants judged taling (2009), in contrast, propose that their meanings are CAUSE ENABLE PREVENT DESPITE best understood as mapping onto qualitatively different causal A models. Whereas “A causes B” means that there is a causal R E P A R E P E A R P E link from A to B, “A enables B” means that A is necessary for B to happen, and that there exists an alternative cause to P R A B. Goldvarg and Johnson-Laird (2001) develop an account based on mental model theory in which “cause” and “al- Figure 1: Analysis of different causal expressions in terms of low” map onto different possible cause-effect pairs (see also configurations of forces. P = patient force, A = agent force, Khemlani, Wasylyshyn, Briggs, & Bello, 2018). What all R = resulting force, E = endstate. 3134 ©2020 The Author(s). This work is licensed under a Creative Commons Attribution 4.0 International License (CC BY). to what extent balls A and B were responsible for ball E’s Table 1: Model derivation for clips 1–4 shown in Figure 2. going through (or missing) the gate. The CSM predicts that a) Aspect Values people’s causal judgments are sensitive to different aspects of Clip 1 2 3 4 causation that capture the extent to which the cause made a Whether 1.00 1.00 0.00 0.00 difference to how and whether the outcome happened. These How 1.00 0.00 1.00 0.00 aspects of causation are defined in terms of counterfactual Sufficient 1.00 1.00 0.00 0.00 contrasts operating over an intuitive understanding of the b) Semantic Values physical domain. People use their intuitive understanding of Clip 1 2 3 4 physics to mentally simulate how things could have turned out differently (cf. Gerstenberg & Tenenbaum, 2017; Ull- No Difference 0.00 0.00 0.80 1.00 Affected 1.00 0.00 1.00 0.00 man, Spelke, Battaglia, & Tenenbaum, 2017), and the result Enabled 1.00 1.00 0.00 0.00 of these counterfactual simulations informs their causal judg- Caused 1.00 0.00 0.00 0.00 ments (Gerstenberg, Peterson, Goodman, Lagnado, & Tenen- baum, 2017). c) Literal Listener Distributions The model we develop here predicts people’s use of Clip 1 2 3 4 the causal expressions “caused”, “enabled”, “affected”, and No Difference 0.00 0.00 0.44 0.56 Affected 0.50 0.00 0.50 0.00 “made no difference”. The model has three components: a Enabled 0.50 0.50 0.00 0.00 causal inference component that computes the different as- Caused 1.00 0.00 0.00 0.00 pects of causation according to the CSM, a semantics compo- nent that defines a logical mapping from aspects of causation d) Speaker Distributions to causal expressions, and a pragmatics component that takes Clip 1 2 3 4 into account how informative each expression would be about No Difference 0.00 0.00 0.47 1.00 what happened (Goodman & Frank, 2016). Affected 0.25 0.00 0.53 0.00 Enabled 0.25 1.00 0.00 0.00 The paper is organized as follows. We first describe our Caused 0.50 0.00 0.00 0.00 model and its three components by illustrating how it applies to some example cases. We then present an experiment that ball B would have gone through the gate if ball A had been re- tests the model, as well as alternative models, on a challeng- moved. The model captures this uncertainty by running noisy ing set of video clips. We conclude by discussing the model’s counterfactual simulations. In simulating the counterfactual, limitations that suggest important avenues for future research. B’s movements are perturbed from the point at which the col- Model lision with ball A would have happened onward. By record- ing the proportion of cases in which the outcome would have We discuss the three components of our model in turn: causal been different from what actually happened in these noisy inference, model semantics, and pragmatics. samples, the model computes the probability that A was a Causal inference whether-cause of e. Whether-causation captures the extent to which the presence of the candidate cause was necessary The causal inference component of our model builds on for the outcome to come about. the CSM (Gerstenberg et al., 2015, 2020), which postulates how-causation For how-causation H , the model computes that causal judgments are sensitive to different aspects of 0 causation including how-causation, whether-causation, and whether the fine-grained counterfactual outcome De in sce- sufficient-causation. We briefly describe each aspect here us- nario S would have been different from what actually hap- ing clips participants viewed in our experiment (see Figure 2). pened De, if the candidate cause A had been changed. Table 1a shows the aspect values for clips 1–4. H (A ! De) = P(De0 6= DejS;change(A)) whether-causation To test for whether-causation W , the model computes the probability that the counterfactual out- This test captures whether A made a difference to how the come e0 in scenario S would have been different from what outcome came about (cf. Lewis, 2000; Woodward, 2011). actually happened e, if the candidate cause A had been re- The outcome event De is construed at a finer level of gran- moved. ularity, with information about the time and space at which the event happened. The change() operation is implemented W (A ! e) = P(e0 6= ejS;remove(A)) as a small perturbation to ball A’s initial position.
Details
-
File Typepdf
-
Upload Time-
-
Content LanguagesEnglish
-
Upload UserAnonymous/Not logged-in
-
File Pages7 Page
-
File Size-