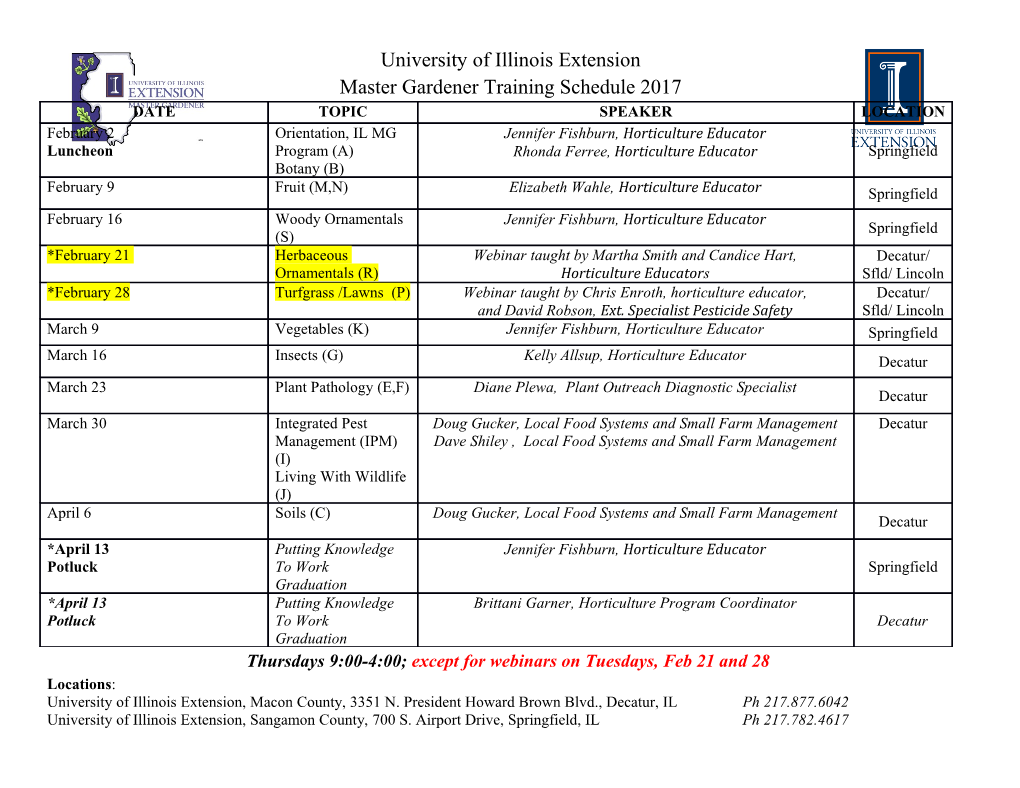
THE UNIVERSITY OF CHICAGO CUE SELECTION AND CATEGORY RESTRUCTURING IN SOUND CHANGE A DISSERTATION SUBMITTED TO THE FACULTY OF THE DIVISION OF THE HUMANITIES IN CANDIDACY FOR THE DEGREE OF DOCTOR OF PHILOSOPHY DEPARTMENT OF LINGUISTICS BY JAMES P. KIRBY CHICAGO, ILLINOIS DECEMBER 2010 Copyright c 2010 by James P. Kirby All rights reserved for rjm CONTENTS ACKNOWLEDGMENTS . vii ABSTRACT . ix VITA ............................................ x LIST OF FIGURES . xii LIST OF TABLES . xv 1 INTRODUCTION AND OVERVIEW . 1 1.1 The role of phonetic variation in sound change . .1 1.2 Sound change in three stages . .4 1.2.1 The multivariate nature of speech . .6 1.3 Cue selection in phonologization . .8 1.3.1 Psychophysical salience and linguistic experience . .8 1.3.2 Cue selection and differential phonologization . 10 1.4 Category restructuring and licensing by cue . 12 1.4.1 Dephonologization and contrast-driven enhancement . 14 1.4.2 Category restructuring and inhibited sound change . 15 1.5 An adaptive model of sound change . 17 1.5.1 The noisy channel . 17 1.5.2 Probabilistic enhancement . 20 1.5.3 Cue restructuring and category restructuring . 22 1.6 Structure of the dissertation . 23 1.6.1 The mixture model of phonetic categories . 24 1.6.2 Phonologization as adaptive subphonemic restructuring . 25 1.6.3 Neutralization as adaptive category restructuring . 26 1.6.4 Implications for the phonetics-phonology interface . 27 2 MODELING SPEECH PRODUCTION AND PERCEPTION . 28 2.1 Desiderata for a representation of speech sound categories . 28 2.1.1 Variability . 28 2.1.2 Multidimensionality . 31 2.2 Classification, categorization, and clustering . 34 2.2.1 Prototypes, exemplars, and density estimation . 37 2.3 Finite mixture models . 45 2.3.1 Mixture models . 46 2.3.2 Gaussian mixture models . 47 2.3.3 Parameter estimation . 49 2.4 Modeling speech production and perception using GMMs . 49 2.4.1 Modeling production: sampling from a density . 49 iv 2.4.2 Modeling perception: the ideal listener . 50 2.4.3 Cue independence and information integration . 52 2.4.4 Cue reliability and cue weight . 56 2.4.5 Classifier accuracy . 59 2.5 Summary . 61 3 AN AGENT-BASED SIMULATION ARCHITECTURE FOR MODELING SOUND CHANGE . 62 3.1 Simulating adaptive enhancement . 62 3.1.1 Computational models of sound change . 63 3.2 An agent-based model . 64 3.2.1 Relations to exemplar theory . 64 3.2.2 Conversing agents . 66 3.3 Summary . 72 4 TRANSPHONOLOGIZATION IN SEOUL KOREAN . 73 4.1 Selection and trading in transphonlogization . 73 4.2 The laryngeal contrast in Seoul Korean . 75 4.2.1 Phonetic cues to the laryngeal contrast in Seoul Korean . 75 4.2.2 Perceptual studies of the Korean laryngeal contrast . 81 4.2.3 Changes in the production and perception of Korean stops . 82 4.2.4 Phonetic bias factors in the production of Korean stops . 86 4.2.5 An adaptive account of sound change in Seoul Korean . 88 4.3 Simulating phonologization in Seoul Korean . 90 4.3.1 Enhancement, no bias . 93 4.3.2 Bias, no enhancement . 94 4.3.3 Bias and enhancement . 95 4.3.4 Summary . 95 4.4 General discussion . 97 4.4.1 Bias factors . 99 4.4.2 Cue relations and speaker control . 100 4.4.3 Phonologization, neutralization, and subphonemic reorganization . 102 4.5 Conclusion . 103 5 PHONETIC CATEGORY RESTRUCTURING . 105 5.1 Unsupervised induction of phonetic categories . 106 5.2 Model-based clustering . 107 5.3 Category restructuring as model selection . 111 5.3.1 Separability in high dimensionality . 117 5.4 The effects of cue availability on category restructuring . 119 5.4.1 Series 1: Optimality . 119 5.4.2 Series 2: Typicality . 123 5.4.3 Discussion . 123 5.5 Covert contrast: the case of Dutch final devoicing . 124 v 5.5.1 The data: Dutch final devoicing . 127 5.5.2 Series 1: Optimality . 128 5.5.3 Series 2: Typicality . 130 5.5.4 Series 3{4: Individual optimality and typicality . 130 5.5.5 Discussion . 133 5.6 General discussion . 136 5.6.1 The role of individual variation . 139 5.6.2 The restructuring problem . 141 5.7 Conclusion . 142 6 SUMMARY AND CONCLUSIONS . 144 6.1 Summary . 144 6.2 Outstanding questions and future directions . 146 6.2.1 Individual variation and population dynamics . 146 6.2.2 Induction of acoustic-phonetic cues . 146 6.2.3 Stage transitions and symbolic representation . 148 6.2.4 Sound change in the laboratory . 149 6.3 Conclusions . 152 REFERENCES . 153 vi ACKNOWLEDGMENTS A great many individuals have contributed in one way or another to the present work; I would like to take the time to thank them here. The members of my dissertation committee, Alan Yu, John Goldsmith, Karen Livescu, Howard Nusbaum, and Jason Riggle, were extremely generous with their advice, perspec- tives, and time; this work is much richer for their input, and I owe a great intellectual debt to them all. Completing this work in a timely fashion would not have been possible without access to a rich pool of empirical speech data collected and reported by other researchers. In particular, I would like to thank Natasha Warner, Allard Jongman, Joan Sereno, and Rach`elKemps for granting me access to their production data on Dutch final obstruent devoicing. My fellow graduate students at UCSD, especially Cindy Kilpatrick, Hannah Rohde, and Dan Brassil, provided friendship, solace, and advice in those first few soul-searching years and beyond. Conversations with my colleagues at the University of Chicago, especially Max Bane, Yaron McNabb, and Morgan Sonderegger, have shaped my thinking about the linguistic enterprise in general and many aspects of the present thesis in particular. In the course of my academic career to date, I have been lucky to have the chance to study with a huge range of talented faculty. At UCSD, my MA advisors Farrell Ackermann, Sharon Rose, and Eric Bakovi´c,along with Andrew Kehler and Chris Barker, encouraged my nascent interests in applying computational techniques to linguistic problems, inspired me to continuous improvement, and graciously supported me even when I decided to switch departments. Jean Mark Gawron and Rob Malouf at SDSU taught me whatever I know about computational linguistics, and tried valiantly to impart much more than I no doubt retained. As Mark predicted, I will now forever remember where I was the first time the Viterbi algorithm was explained to me. As an undergraduate at the University of Wisconsin- Madison, Andrew Sihler, Joe Salmons, and Tom Purnell answered questions, wrote letters, vii and encouraged my initial interest in the language sciences. Without their friendship and support, it is safe to say this document would not exist. Finally, I would like to thank my family, especially my parents Monica and David, for love and encouragement, and to Rachel, my partner in this adventure and surely many more to come. Chicago, November 2010 viii ABSTRACT Changes to the realization of phonetic cues, such as vowel length or voice onset time, can have differential effects on the system of phonological categories. In some cases, variability or bias in phonetic realization may cause a contrast between categories to collapse, while in other cases, the contrast may persist through the phonologization of a redundant cue (Hyman, 1976). The goals of this dissertation are to better understand the subphonemic conditions under which a contrast is likely to survive and when it is likely to collapse, as well as to understand why certain cues are more likely to be phonologized than others. I explore these questions by considering the transmission of speech sounds over a noisy channel (Shannon and Weaver, 1948), hypothesizing that when the precision of a contrast along one acoustic dimension is reduced, other dimensions may be enhanced to compensate (the probabilistic enhancement hypothesis). Whether this results in phonologization or neutralization depends on both the degree to which the contrast is threatened as well as the informativeness of the cues that signal it. In order to explore this hypothesis, phonological categories are modeled as finite mixtures, which provide a natural way to generate, classify, and cluster objects in a multivariate setting. These mixtures are then embedded in an agent-based simulation framework and used to simulate the ongoing process of phonologization of pitch in Seoul Korean (Silva, 2006a,b; Kang and Guion, 2008). The results demonstrate that adaptive enhancement can account for both cue selection as well as the appearance of cue trading in phonologization. Additional data from the incomplete neutralization of final voicing in Dutch (Warner, Jongman, Sereno and Kemps, 2004) are then used to show how variation in phonetic realization can influence the loss or maintenance of phonological categories. Together, these case studies illustrate how variation in production and perception of subphonemic cues can impact the system of phonological contrasts. ix VITA 2000 . B.A., Linguistics and Germanic Linguistics, University of Wisconsin-Madison 2005 . M.A., Linguistics, University of California-San Diego 2007 . M.A., Linguistics, University of Chicago 2010 . Ph.D, Linguistics, University of Chicago PUBLICATIONS Kirby, James P. (to appear a). The role of probabilistic enhancement in phonologization. In A. Yu (ed.), Origins of Sound Change: Approaches to Phonologization. Oxford: Oxford University Press. Kirby, James P. (to appear b). Acquisition of covert contrast: an unsupervised learning approach. In A. Baker, R. Baglini, T. Grinsell, J. Keane, and J. Thomas (eds.), Proceedings from the Annual Meeting of the Chicago Linguistic Society 46,.
Details
-
File Typepdf
-
Upload Time-
-
Content LanguagesEnglish
-
Upload UserAnonymous/Not logged-in
-
File Pages192 Page
-
File Size-