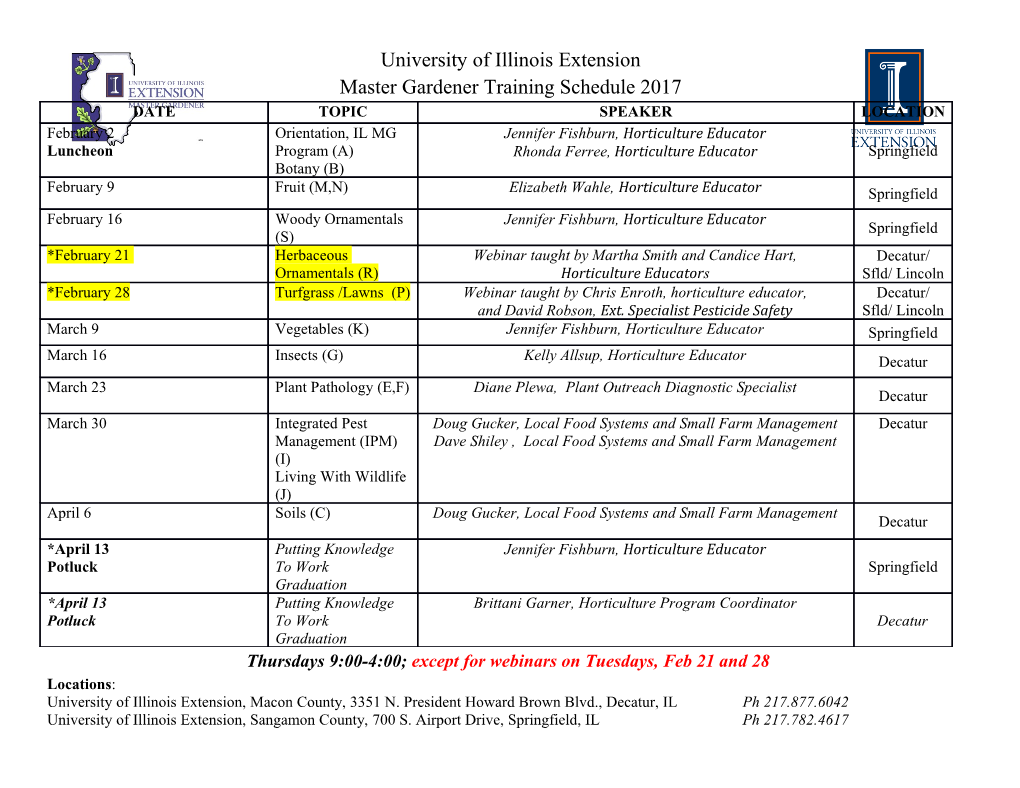
Lecture 14 Simple Linear Regression Ordinary Least Squares (OLS) Consider the following simple linear regression model Yi = α + βXi + "i where, for each unit i, • Yi is the dependent variable (response). • Xi is the independent variable (predictor). • "i is the error between the observed Yi and what the model predicts. This model describes the relation between Xi and Yi using an intercept and a slope parameter. The error "i = Yi − α − βXi is also known as the residual because it measures the amount of variation in Yi not explained by the model. ^ 2 We saw last class that there existsα ^ and β that minimize the sum of "i . Specifically, we wish to findα ^ and β^ such that n X 2 Yi − (^α + βX^ i) i=1 is the minimum. Using calculus, it turns out that P(X − X¯)(Y − Y¯ ) α^ = Y¯ − β^X¯ β^ = i i : P 2 (Xi − X¯) OLS and Transformation If we center the predictor, X~i = Xi − X¯, then X~i has mean zero. Therefore, P X~ (Y − Y¯ ) α^∗ = Y¯ β^∗ = i i : P ~ 2 Xi ∗ By horizontally shifting the value of Xi, note that β = β, but the intercept changed to the overall average of Yi Consider the linear transformation Zi = a + bXi with Z¯ = a + bX¯. Consider the linear model ∗ ∗ Yi = α + β Zi + "i 1 Letα ^ and β^ be the estimates using X as the predictor. Then the intercept and slope using Z as the predictor are P(Z − Z¯)(Y − Y¯ ) β^∗ = i i P 2 (Zi − Z¯) P(a + bX − a − bX¯)(Y − Y¯ ) = i i P(a + bX¯ − a − bX¯)2 P(bX − bX¯)(Y − Y¯ ) = i i P(bX¯ − bX¯)2 b P(X − X¯)(Y − Y¯ ) 1 = i i = β^ b2 P(X¯ − X¯)2 b and α^∗ = Y¯ − β^∗Z¯ β^ aβ^ a = Y¯ − (a + bX¯) = Y¯ − β^X¯ − =α ^ − β^ b b b Zi−a We can get the same results by substituting Xi = b into the linear model. Yi = α + βXi + "i Z − a Y = α + β i + " i b i aβ β Y = α − + Z + " i b b i i ∗ ∗ Yi = α + β Zi + "i Properties of OLS Given the estimatesα ^ and β^, we can define (1) the estimated predicted value Y^i and (2) the estimated residual" ^i. Y^i =α ^ + βX^ i "^i = Yi − Y^i = Yi − α^ − βX^ i The least squared estimates have the following properties. P 1. i "^i = 0 n n n n X X X X "^i = (Yi − α^ − βX^ i) = Yi − nα^ − β^ Xi i=1 i=1 i=1 i=1 = nY¯ − nα^ − nβ^X¯ = n(Y¯ − α^ − β^X¯) = n(Y¯ − (Y¯ − β^X¯) − β^X¯) = 0 2 2. X¯ and Y¯ is always on the fitted line. α^ + β^X¯ = (Y¯ − β^X¯) + β^X¯ = Y¯ ^ sY 3. β = rXY × , where sY and sX are the sample standard deviation of X and Y , and rXY is sX the correlation between X and Y . Note that the sample correlation is given by: P (Xi − X¯)(Yi − Y¯ ) rXY = : (n − 1)sX sY Therefore, P(X − X¯)(Y − Y¯ ) n − 1 s s β^ = i i × × Y × X P 2 (Xi − X¯) n − 1 sY sX Cov(X; Y ) sY sX = 2 × × sX sY sX Cov(X; Y ) s 1 = × Y × sX sY 1 sX sY = rXY × sX 4. β^ is a weighted sum of Yi. P(X − X¯)(Y − Y¯ ) β^ = i i P 2 (Xi − X¯) P(X − X¯)Y P(X − X¯)Y¯ = i i − i P 2 P 2 (Xi − X¯) (Xi − X¯) P(X − X¯)Y Y¯ P(X − X¯) = i i − i P 2 P 2 (Xi − X¯) (Xi − X¯) P(X − X¯)Y = i i − 0 P 2 (Xi − X¯) ¯ X Xi − X = Y P 2 i (Xi − X¯) ¯ X Xi − X = w Y ; w = i i i P 2 (Xi − X¯) 3 5.^α is a weighted sum of Yi. α^ = Y¯ − β^X¯ X 1 X = Y − X¯ w Y n i i i ¯ ¯ X 1 X(Xi − X) = v Y ; v = − i i i P 2 n (Xi − X¯) P 6. Xi"^i = 0. X X X Xi"^i = (Yi − Y^i)Xi = (Yi − α^ − βX^ i)Xi X = (Yi − (Y¯ − β^X¯) − βX^ i)Xi X = ((Yi − Y¯ ) − β^(Xi − X¯))Xi X X = Xi(Yi − Y¯ ) − β^ Xi(Xi − X¯) X 2 X = β^ (Xi − X¯) − β^ Xi(Xi − X¯) X 2 = β^ (Xi − X¯) − Xi(Xi − X¯) ^ X 2 ¯ ¯ 2 2 ¯ = β Xi − 2XiX + X − Xi + XiX) X 2 = β^ X¯ − XiX¯ X = β^X¯ X¯ − Xi = 0 P(X − X¯)(Y − Y¯ ) P X (Y − Y¯ ) Note that β^ = i i = i i P 2 P 2 (Xi − X¯) (Xi − X¯) 7. Partition of total variation. (Yi − Y¯ ) = Yi − Y¯ + Y^i − Y^i = (Y^i − Y¯ ) + (Yi − Y^i) • (Yi − Y¯ ): total deviation of the observed Yi from a null model (β = 0). • (Y^ − Y¯ ): deviation of the modeled value Y^i (β 6= 0) from a null model (β = 0). • (Y^ − Yi): deviation of the modeled value from the observed value. Total Variations (Sum of Squares) P 2 • (Yi − Y¯ ) : total variation: (n − 1) × V (Y ). • P(Y^ − Y¯ )2: variation explained by the model 4 P ^ 2 P 2 • (Y − Yi) : variation not explained by the model; residual sum of squares (SSE), "^i Partition of Total Variation X 2 X 2 X 2 (Yi − Y¯ ) = (Y^i − Y¯ ) + (Yi − Y^i) Proof: 2 X 2 X h i (Yi − Y¯ ) = (Y^i − Y¯ ) + (Yi − Y^i) X 2 X 2 X = (Y^i − Y¯ ) + (Yi − Y^i) + 2 (Y^i − Y¯ )(Yi − Y^i) X 2 X 2 = (Y^i − Y¯ ) + (Yi − Y^i) where, X X h i (Y^i − Y¯ )(Yi − Y^i) = (^α + βX^ i) − (^α + β^X¯) "^i X h i = β^(Xi − X¯) "^i X X = β^ Xi"^i − β^X¯ "^i = 0 + 0 P 2 8. Alternative formula for "^i X 2 X 2 X 2 (Yi − Y^i) = (Yi − Y¯ ) − (Y^i − Y¯ ) X 2 X 2 = (Yi − Y¯ ) − (^α + βX^ i − Y¯ ) X 2 X 2 = (Yi − Y¯ ) − (Y¯ + βXX¯ + βX^ i − Y¯ ) 2 X 2 X h i = (Yi − Y¯ ) − β^(Xi − X¯) X 2 2 X 2 = (Yi − Y¯ ) − β^ (Xi − X¯) or P ¯ ¯ 2 X X (Xi − X)(Yi − Y ) (Y − Y^ )2 = (Y − Y¯ )2 − i i i P 2 (Xi − X¯) 9. Variation Explained by the Model: 5 P 2 An easy way to calculate (Y^i − Y¯ ) is X 2 X 2 X 2 (Y^i − Y¯ ) = (Yi − Y¯ ) − (Yi − Y^i) P ¯ ¯ 2 X (Xi − X)(Yi − Y ) = (Y − Y¯ )2 − i P 2 (Xi − X¯) P 2 (Xi − X¯)(Yi − Y¯ ) = P 2 (Xi − X¯) 10. Coefficient of Determination r2: variation explained by the model P(Y^ − Y¯ )2 r2 = = i P 2 total variation (Yi − Y¯ ) (a) r2 is related the residual variance. X 2 X 2 X 2 (Yi − Y^i) = (Yi − Y¯ ) − (Y^i − Y¯ ) P ¯ 2 hX X i (Yi − Y ) = (Y − Y¯ )2 − (Y^ − Y¯ )2 × i i P 2 (Yi − Y¯ ) 2 X 2 = (1 − r ) × (Yi − Y¯ ) Diving the right-hand side by n − 2 and the left-hand side by n − 1, for large n s2 ≈ (1 − r2)Var(Y ) (b) r2 is the square of the sample correlation between X and Y . P(Y^ − Y¯ )2 r2 = i P 2 (Yi − Y¯ ) P 2 (Xi − X¯)(Yi − Y¯ ) = P 2 P 2 (Yi − Y¯ ) (Xi − X¯) " #2 P(X − X¯)(Y − Y¯ ) = i i = r2 pP 2pP 2 XY (Yi − Y¯ ) (Xi − X¯) Statistical Inference for OLS Estimates Parametersα ^ and β^ can be estimated for any given sample of data. Therefore, we also need to consider their sampling distributions because each sample of (Xi;Yi) pairs will result in different estimates of α and β. 6 Consider the following distribution assumption on the error, iid 2 Yi = α + βXi + "i "i ∼ N (0; σ ) : The above is now a statistical model that describes the distribution of Yi given Xi. Specifically, we assume the observed Yi is error-prone but centered around the linear model for each value of Xi. iid 2 Yi ∼ N(α + βXi; σ ) The error distribution results in the following assumptions. 1. Homoscedasticity: variance of Yi is the same for any Xi. 2 V (Yi) = V (α + βXi + "i) = V ("i) = σ 2. Linearity: the mean of Yi is linear with respect to Xi E(Yi) = E(α + βXi + "i) = α + βXi + E("i) = α + βXi 3. Independence: Yi are independent of each other. Cov(Yi;Yj) = Cov(α + βXi + "i; α + βXj + "j) = Cov("i;"j) = 0 We can now investigate the bias and variance of OLS estimatorsα ^ and β^. We assume that Xi are known constants. • β^ is unbiased. ¯ hX i Xi − X E[β^] = E w Y ; w = i i i P 2 (Xi − X¯) X = wiE[Yi] X = wi(α + βXi) X X = α wi + β wiXi = β 7 Because X 1 X w = (X − X¯) = 0 i P 2 i (Xi − X¯) X 1 X w X = X (X − X¯) i i P 2 i i (Xi − X¯) 1 X ¯ = P P Xi(Xi − X) Xi(Xi − X¯) − X¯(Xi − X¯) 1 X ¯ = P P Xi(Xi − X) Xi(Xi − X¯) − X¯ (Xi − X¯) 1 X ¯ = P Xi(Xi − X) Xi(Xi − X¯) − 0 = 1 • α^ is unbiased. E[^α] = E[Y¯ − β^X¯] = E[Y¯ ] − XE¯ [β^] 1 X 1 X = E Y − βX¯ = E [α + βX ] − βX¯ n i n i 1 = (nα + nβX ) − βX¯ n i = α + βX¯ − βX¯ = α σ2 σ2 • β^ has variance = , where σ2 is the residual variance and s2 is the Pn ¯ 2 2 x i=1(Xi − X1) (n − 1)sx sample variance of x1; : : : ; xn.
Details
-
File Typepdf
-
Upload Time-
-
Content LanguagesEnglish
-
Upload UserAnonymous/Not logged-in
-
File Pages11 Page
-
File Size-