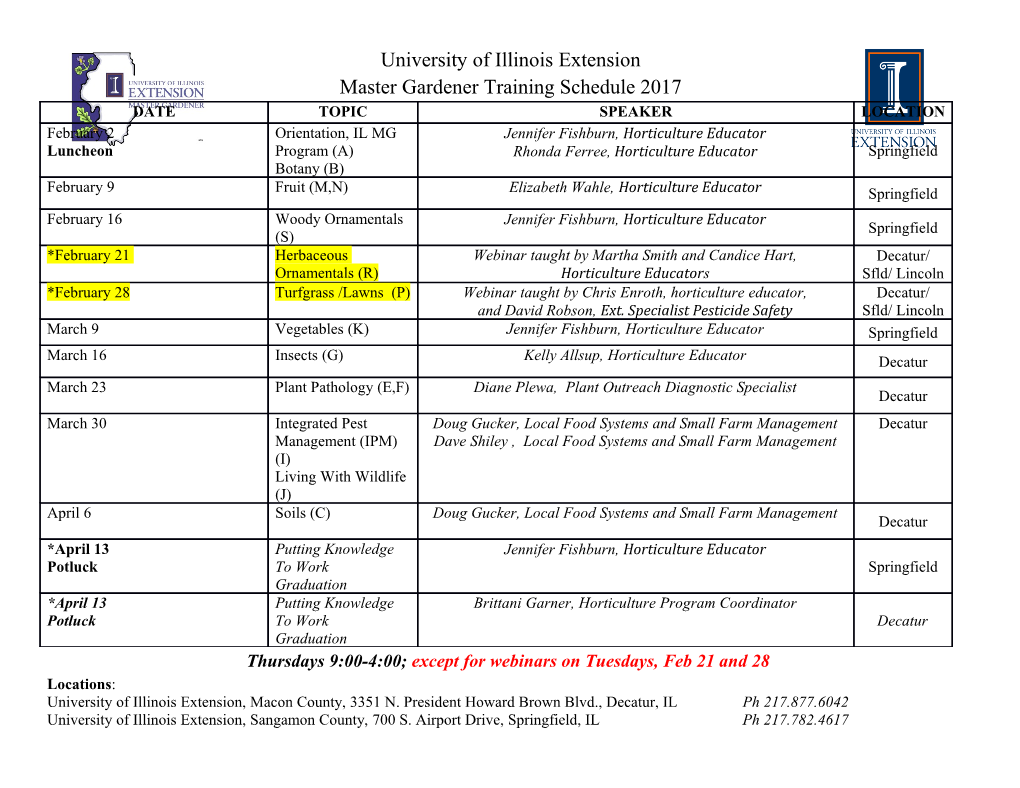
Principal Component Analysis, A Powerful Scoring Technique George C. J. Fernandez, University of Nevada - Reno, Reno NV 89557 ABSTRACT be broadly classified into unsupervised and supervised learning methods. The main Data mining is a collection of analytical difference between supervised and techniques to uncover new trends and unsupervised learning methods is the patterns in massive databases. These data underlying model structure. In supervised mining techniques stress visualization to learning, relationships between input and the thoroughly study the structure of data and to target variables are being established. But, in check the validity of the statistical model fit unsupervised learning, no variable is defined as which leads to proactive decision making. a target or response variable. In fact, for most Principal component analysis (PCA) is one of types of unsupervised learning, the targets are the unsupervised data mining tools used to same as the inputs. All the variables are reduce dimensionality in multivariate data and assumed to be influenced by a few components to develop composite scores. In PCA, in unsupervised learning. Because of this dimensionality of multivariate data is reduced feature, it is possible to study large complex by transforming the correlated variables into models with unsupervised learning than with linearly transformed un-correlated variables. supervised learning. PCA summarizes the variation in a correlated Unsupervised learning methods are used multi-attribute to a set of uncorrelated in many fields including medicine and in components, each of which is a particular microarray studies [1] under a wide variety of linear combination of the original variables. names. Analysis of multivariate data plays a key The extracted uncorrelated components are role in data mining and knowledge discovery. called principal components (PC) and are Multivariate data consists of many different estimated from the eigenvectors of the attributes or variables recorded for each covariance or correlation matrix of the original observation. If there are p variables in a variables. Therefore, the objective of PCA is to database, each variable could be regarded as achieve parsimony and reduce dimensionality constituting a different dimension, in a p- by extracting the smallest number dimensional hyperspace. This multi-dimensional components that account for most of the hyperspace is often difficult to visualize, and variation in the original multivariate data and thus the main objectives of unsupervised to summarize the data with little loss of learning methods are to reduce dimensionality information. A user-friendly SAS application and scoring all observations based on a utilizing the latest capabilities of SAS macro to composite index. Also, summarizing perform PCA is presented here. Previously multivariate attributes by, two or three that can published diabetes screening data containing be displayed graphically with minimal loss of multi-attributes is used to reduce multi information is useful in knowledge discovery. attributes into few dimensions. The most commonly practiced unsupervised methods are latent variable Key Words: Data mining, SAS, Data models (principal component and factor exploration, Bi-Plot, Multivariate data, SAS analyses). In principal component analysis macro, (PCA), dimensionality of multivariate data is reduced by transforming the correlated Introduction variables into linearly transformed uncorrelated Data mining is the process of selecting, variables. In factor analysis, a few uncorrelated exploring, and modeling large amounts of hidden factors that explain the maximum data to uncover new trends and patterns in amount of common variance and are massive databases. These analyses lead to responsible for the observed correlation among proactive decision making by stressing data the multivariate data are extracted. The exploration to thoroughly study the structure relationship between the multi-attributes and of data and to check the validity of statistical the extracted hidden factors are then models that fit. Data mining techniques can investigated. Because it is hard to visualize multi- the dataset are measured using different units dimensional space, principal components (annual income, educational level, numbers of analysis (PCA), a popular multivariate cars owned per family) or if different variables technique, is mainly used to reduce the have different variances. Using the correlation dimensionality of p multi-attributes to two or matrix is equivalent to standardizing the three dimensions. A brief account on non- variables to zero mean and unit standard mathematical description and application of deviation. The statistical theory, methods, and PCA are discussed in this paper. For a the computation aspects of PCA are presented mathematical account of principal component in details elsewhere [2]. analysis, the readers are encouraged to refer While the PCA and Exploratory factor analysis the multivariate statistics book [2] (EFA) analyses are functionally very similar and PCA summarizes the variation in a are used for data reduction and summarization, correlated multi-attribute to a set of they are quite different in terms of the uncorrelated components, each of which is a underlying assumptions. In EFA, the variance of particular linear combination of the original a single variable can be partitioned into variables. The extracted uncorrelated common and unique variances. The common components are called principal components variance is considered shared by other variables (PC) and are estimated from the eigenvectors included in the model and the unique variance of the covariance or correlation matrix of the that includes the error component is unique to original variables. Therefore, the objective of that particular variable. Thus, EFA analyzes only PCA is to achieve parsimony and reduce the common variance of the observed variables dimensionality by extracting the smallest whereas PCA summarizes the total variance and number components that account for most of makes no distinction between common and the variation in the original multivariate data unique variance. and to summarize the data with little loss of The selection of PCA over the EFA is information. dependent upon the objective of the analysis In PCA, uncorrelated PC’s are and the assumptions about the variation in the extracted by linear transformations of the original variables. EFA and PCA are considered original variables so that the first few PC’s similar since the objectives of both analyses are contain most of the variations in the original to reduce the original variables into fewer dataset. These PCs are extracted in components, called factors or principal decreasing order of importance so that the components. However, they are also different first PC accounts for as much of the variation since the extracted components serve different as possible and each successive component purposes. In EFA, a small number of factors are accounts for a little less. Following PCA, extracted to account for the inter-correlations analyst tries to interpret the first few principal among the observed variables and to identify components in terms of the original variables, the latent factors that explain why the variables and thereby have a greater understanding of are correlated with each other. But, the the data. To reproduce the total system objective of PCA is to account for the maximum variability of the original p variables, we need portion of the variance present in the original all p PCs. However, if the first few PCs variables with a minimum number of PC. account for a large proportion of the If the observed variables are measured variability (80-90%), we have achieved our relatively error free, or if it is assumed that the objective of dimension reduction. Because the error and unique variance represent a small first principal component accounts for the co- portion of the total variance in the original set variation shared by all attributes, this may be of the variables, then PCA is appropriate. But if a better estimate than simple or weighted the observed variables are only indicators of the averages of the original variables. Thus, PCA latent factor, or if the error variance represents can be useful when there is a severe high- a significant portion of the total variance, then degree of correlation present in the multi- the appropriate technique is EFA. attributes. Previously published diabetes screening In PCA, the extractions of PC can be data [3] containing multi-attributes from made using either original multivariate data glucose tolerance tests, relative weight (x1: sets or using the covariance or the correlation RELWT), fasting plasma glucose levels (x2: matrix if the original data set is not available. GLUFAST), test plasma glucose levels (x3: In deriving PC, the correlation matrix is GLUTEST), plasma insulin level during test (x4: commonly used when different variables in INSTEST), and steady state plasma glucose levels (x5: SSPG) are used here to information on the nature of multi-attributes demonstrate the features of PCA. A that can be used to decide whether to use user-friendly SAS application developed by standardized or un-standardized data in the the author utilizing the latest capabilities of PCA analysis. The minimum and the maximum SAS macros to perform PCA and EFA analyses values describe the range of variation in each with data exploration is presented here. attribute and help to check for any extreme
Details
-
File Typepdf
-
Upload Time-
-
Content LanguagesEnglish
-
Upload UserAnonymous/Not logged-in
-
File Pages6 Page
-
File Size-