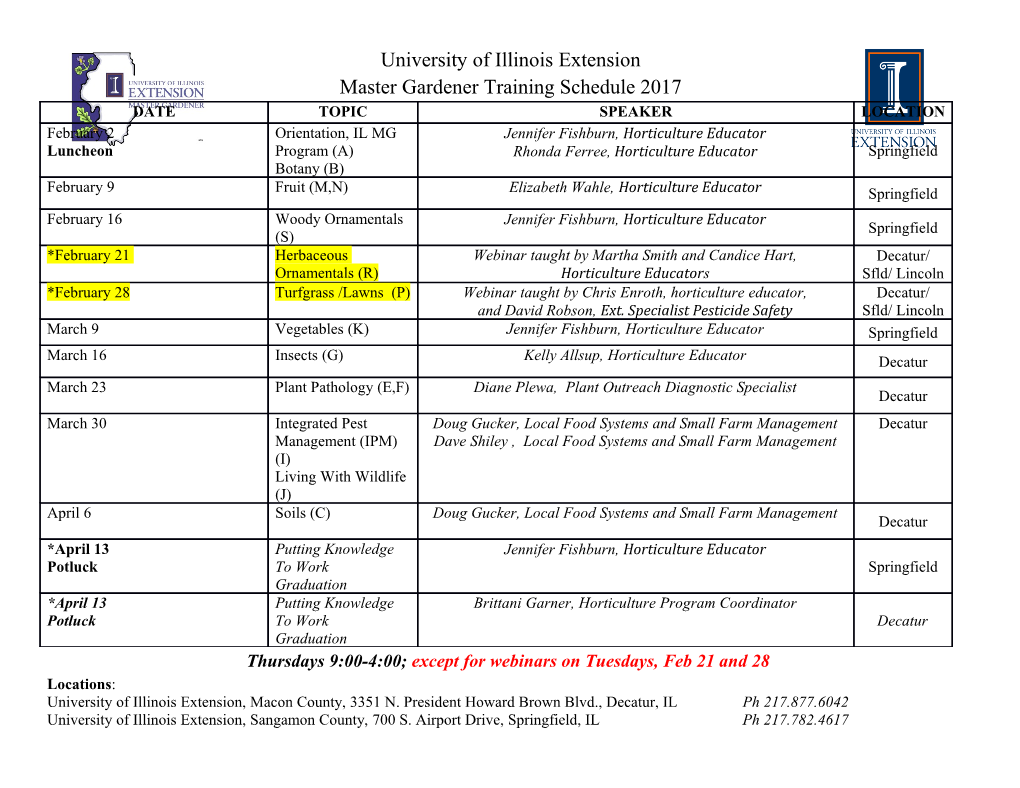
Confidence Interval Some concepts: Interval estimate, coverage probability, confidence coefficient, confidence interval (CI) Definition: an interval estimate for a real-valued parameter θ based on a sample X ≡ (X1,… , Xn) is a pair of functions L(X ) and U(X ) so that L(X ) ≤ U(X ) for all X , that is 퐿푋, 푈푋. Note: • The above is a two-sided confidence interval, one can also define one-sided intervals: (-∞, U(X )] or [L(X), ∞). Definition: the coverage probability of an interval estimator is 푃휃 ∈ 퐿푋, 푈푋 = 푃퐿푋 ≤ 휃, 푈푋 ≥ 휃 Note: • This is the probability that the random interval 퐿푋, 푈푋 covers the true θ. • One problem about the coverage probability is that it can vary depend on what θ is. Definition: For an interval estimator 퐿푋, 푈푋 of a parameter θ, the confidence coefficient ≡ 푖푛푓푃휃 ∈ 퐿푋, 푈푋. Note: • The term confidence interval refers to the interval estimate along with its confidence coefficient. 1 There are two general approaches to derive the confidence interval: (1) the privotal quantitymethod, and (2) invert the test, a introduced next. 1. General approach for deriving CI’s : The Pivotal Quantity Method Definition: A pivotal quantity is a function of the sample and the parameter of interest. Furthermore, its distribution is entirely known. Example. Point estimator and confidence interval for µ when the population is normal and the population variance is known. - Let X 1 , X 2 ,, X n be a random sample for a normal population with mean and variance 2 . That is, iid . X N(, 2 ),i 1,...,n . i ~ - For now, we assume that 2 is known. (1). We start by looking at the point estimator of : 2 X ~ N(, ) n (2). Then we found the pivotal quantity Z: X Z ~ N(0,1) n Now we shall start the derivation for the symmetrical CI’s for µ from the PDF of the pivotal quantity Z 2 100(1-)% CI for , 0<<1 (e.g. =0.05 ⇒ 95% C.I.) P(Z 2 Z Z 2 ) 1 X P(Z 2 Z 2 ) 1 n P(Z 2 X Z 2 ) 1 n n P(X Z 2 X Z 2 ) 1 n n P(X Z 2 X Z 2 ) 1 n n P(X Z 2 X Z 2 ) 1 n n (3) ∴ the 100(1-α)% C.I. for μ is [X Z 2 , X Z 2 ] n n *Note, some special values for 훂 and the corresponding 퐙훂/ퟐvalues are: 3 1. The 95% CI, where 훂 = ퟎ. ퟎퟓ and the corresponding 퐙훂 = 퐙ퟎ.ퟎퟐퟓ = ퟏ. ퟗퟔ ퟐ 2. The 90% CI, where 훂 = ퟎ. ퟏ and the corresponding 퐙훂 = 퐙ퟎ.ퟎퟓ = ퟏ. ퟔퟒퟓ ퟐ 3. The 99% CI, where 훂 = ퟎ. ퟎퟏ and the corresponding 퐙훂 = 퐙ퟎ.ퟎퟎퟓ = ퟐ. ퟓퟕퟓ ퟐ ∴Recall the 100(1-α)% symmetric C.I. for μ is [X Z 2 , X Z 2 ] n n *Please note that this CI is symmetric around 푿 Lsy 2 Z The length of this CI is: 2 n (4) Now we derive a non-symmetrical CI: 4 P(Z Z Z ) 1 2 3 3 100(1-α)% C.I. for μ ⇒ [X Z , X Z ] 2 1 3 n 3 n Compare the lengths of the C.I.’s, one can prove theoretically that: L (Z Z ) L 2 Z 2 sy 3 3 n 2 n You can try a few numerical values for α, and see for yourself. For example, 훂 = ퟎ. ퟎퟓ Theorem: Let f(y) be a unimodal pdf. If the interval satisfies (i) 푓(푦) 푑푦 =1− 훼 ∫ (ii) f(a) = f(b) > 0 ∗ ∗ (iii) a ≤ 푦 ≤ b, where 푦 is a mode of f(y), then [a, b] is the shortest lengthed interval satisfying (i). Note: • y in the above theorem denotes the pivotal statistic upon which the CI is based • f(y) need not be symmetric: (graph) ∗ • However, when f(y) is symmetric, and 푦 = 0, then a = -b. This is the case for the N(0, 1) density and the t density. 5 Example. Large Sample Confidence interval for a population mean (*any population) and a population proportion p <Theorem> Central Limit Theorem 푋 − 휇 → 푍 = ⎯⎯ 푁(0,1) 휎/√푛 When n is large enough, we have 푋 − 휇 푍 = ~̇ 푁(0,1) 휎/√푛 That means Z follows approximately the normal (0,1) distribution. Application #1. Inference on when the population distribution is unknown but the sample size is large X Z ~ N (0,1) / n By Slutsky’s Theorem We can also obtain another pivotal quantity when σ is unknown by plugging the sample standard deviation S as follows: X Z ~ N (0,1) S/ n We subsequently obtain the 100(1 )% C.I. using the second P.Q. S for : X Z /2 n Application #2. Inference on one population proportion p when the population is Bernoulli(p) *** i... i d Let Xi ~ Bernoulli ( p ), i 1, , n , please find the 100(1-α)% CI for p. n X i Point estimator : pˆ X i1 (ex. n 1000 , pˆ 0.6 ) n Our goal: derive a 100(1-α)% C.I. for p Thus for the Bernoulli population, we have: 휇 = 퐸(푋) = 푝 휎 = 푉푎푟(푋) = 푝(1 − 푝) Thus by the CLT we have: 6 X p Z ~ N (0,1) p(1 p ) n Furthermore, we have for this situation: 푋 = 푝̂ Therefore we obtain the following pivotal quantity Z for p: pˆ p Z ~ N (0,1) p(1 p ) n By Slustky’s theorem, we can replace the population proportion in the denominator with the sample proportion and obtain another pivotal quantity for p: 푝̂ − 푝 푍∗ = ~̇ 푁(0,1) 푝̂(1 − 푝̂) 푛 # Thus the 100(1 )% (approximate, or large sample) C.I. for p based on the second pivotal quantity 푍∗ is: * Pz(/ 2 Z z / 2 ) 1 pˆ p Pz( z ) 1 / 2pˆ(1 pˆ ) / 2 n pˆ(1p ˆ ) pˆ (1 pˆ ) Ppz(ˆ ppzˆ ) 1 / 2n / 2 n pˆ(1 pˆ ) pˆ (1 p ˆ ) Ppz(ˆ ppz ˆ ) 1 / 2n / 2 n => The 100(1 )% large sample C.I. for p is pˆ(1 pˆ )p ˆ (1 pˆ ) [pZˆ , pZˆ ] . /2n /2 n # CLT => n large usually means n 30 # special case for the inference on p based on a Bernoulli population. The sample size n is large means n Let X X i , large sample means: i1 npˆ X 5 (*Here X= total # of ‘S’), and n(1 pˆ ) nX 5 (*Here n-X= total # of ‘F’) 7 Example: normal population, 2 unknown 2 1. Point estimation : X~ N (, ) n X 2. Z ~ N (0,1) n 3. Theorem. Sampling from normal population a. Z~ N (0,1) n1 S 2 b. W ~ 2 2 n1 c. Z and W are independent. Z X Definition. T ~ t W( n 1) S n n1 ------ Derivation of CI, normal population, 2 is unknown ------ 2 X~ N (, ) is not a pivotal quantity. n 2 X ~ N (0, ) is not a pivotal quantity. n X Z ~ N (0,1) is not a pivotal quantity. / n Remove !!! X Therefore T ~ t is a pivotal quantity. S/ n n1 Now we will use this pivotal quantity to derive the 100(1- α)% confidence interval for μ. We start by plotting the pdf of the t-distribution with n-1 degrees of freedom as follows: 8 The above pdf plot corresponds to the following probability statement: Pt(n1, /2 Tt n 1, /2 ) 1 X => Pt( t ) 1 n1, /2S/ n n 1, /2 SS => Pt( X t ) 1 n1, /2n n 1, /2 n SS => PXt( Xt ) 1 n1, /2n n 1, /2 n SS => PXt( Xt ) 1 n1, /2n n 1, /2 n SS => PXt( Xt ) 1 n1, /2n n 1, /2 n => Thus the 100(1 )% C.I. for when 2 is unknown is SS [Xt , Xt ] . (*Please note that n1, /2n n 1, /2 n tn1, /2 Z /2 ) 9 Example. Inference on 2 population means, when both populations are normal. We have 2 independent samples, the population variances 2 2 2 are unknown but equal (1 2 ) pooled- variance t-test. iid Data: X,, X ~(, N 2 ) 1n1 1 1 iid 2 Y,, Y ~(, N ) 1n2 2 2 Goal: Compare 1 and 2 1) Point estimator: σ σ 1 1 μ−μ =X −Y~N μ −μ, + =N μ −μ, + σ n n n n 2) Pivotal quantity: (X Y )( 1 2 ) 1 1 Z n1 n 2 (X Y )( 1 2 ) T ~ tn n 2 W (n 1) S2 ( n 1) S 2 1 1 1 2 1 1 2 2 S 2 2 p n1 n 2 2 n 1 n 2 n1 n 2 2 . 2 2 2 (n1 1) S 1 ( n 2 1) S 2 where S p is the pooled variance. n1 n 2 2 This is the PQ of the inference on the parameter of interest (1 2 ) 10 3) Confidence Interval for (1 2 ) 1 Pt ( Tt ) n n2, n n 2, 1 22 1 2 2 (X Y )( 1 2 ) 1 Pt ( t ) n n2, n n 2, 1 221 1 1 2 2 S p n1 n 2 1 1 1 1 1(P t Sp ()() X Y 1 2 t S p ) n n2, n n 2, 1 22n1 n 2 1 2 2 n 1 n 2 1 1 1 1 1PXYt ( Sp 1 2 XYt S p ) n n2, n n 2, 1 22 n1 n 2 1 2 2 n1 n 2 This is the 100(1 )% C.I for (1 2 ) 11 2.
Details
-
File Typepdf
-
Upload Time-
-
Content LanguagesEnglish
-
Upload UserAnonymous/Not logged-in
-
File Pages13 Page
-
File Size-