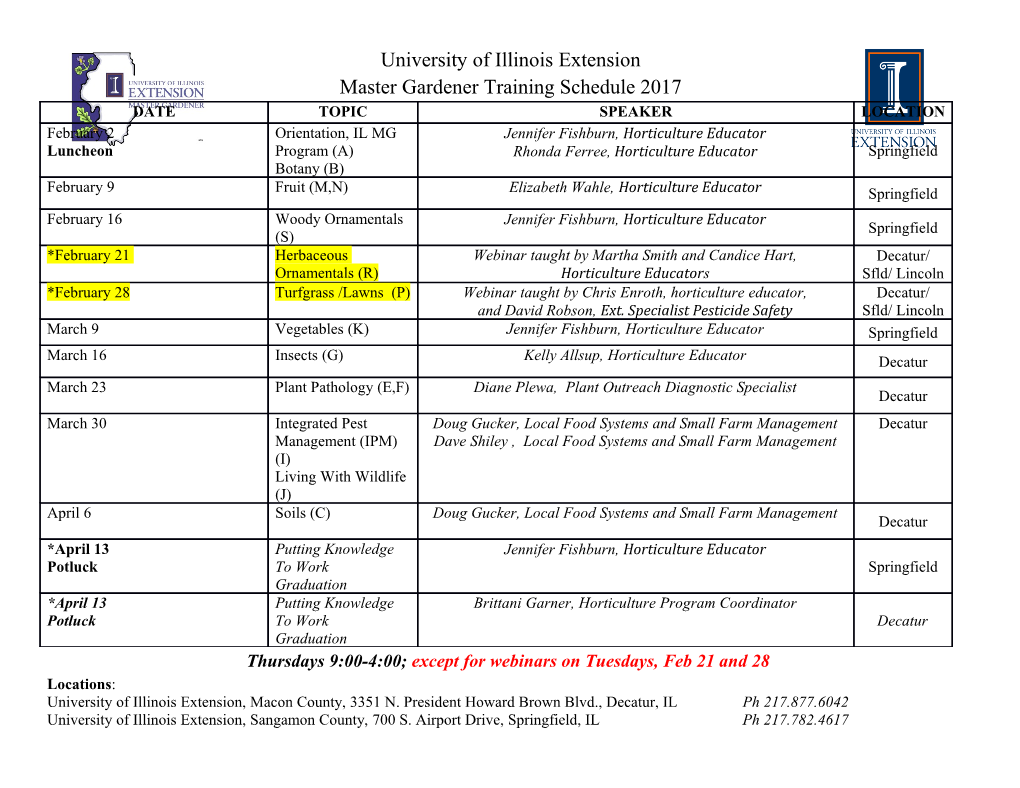
Rethinking Code Generation in Compilers Christian Schulte SCALE KTH Royal Institute of Technology & SICS (Swedish Institute of Computer Science) joint work with: Mats Carlsson SICS Roberto Castañeda Lozano SICS + KTH Frej Drejhammar SICS Gabriel Hjort Blindell KTH funded by: Ericsson, Vetenskapsrådet Compilation source front-end optimizer back-end assembly program (code generator) program Oct Oct 1, 2014 • Front-end: depends on source programming language • changes infrequently SCALE Schulte, • Optimizer: independent optimizations • changes infrequently CodeGeneration Rethinking • Back-end: depends on processor architecture 2 • changes often: new architectures, new features, … Building a Compiler source front-end optimizer back-end assembly program (code generator) program Oct Oct 1, 2014 LLVM Schulte, SCALE Schulte, Infrequent changes: front-end & optimizer • CodeGeneration Rethinking • reuse state-of-the-art: LLVM, for example 3 Building a Compiler source front-end optimizer back-end assembly program (code generator) program Oct Oct 1, 2014 Unison Schulte, SCALE Schulte, Infrequent changes: front-end & optimizer • CodeGeneration Rethinking • reuse state-of-the-art: LLVM, for example • Frequent changes: back-end • use flexible approach: Unison (project this talk is based on) 4 State-of-the-art instruction selection Oct Oct 1, 2014 add r0 r1 r2 x = y + z; mv $a6f0 r0 Schulte, SCALE Schulte, • Code generation organized into stages • instruction selection, CodeGeneration Rethinking 5 State-of-the-art register allocation Oct Oct 1, 2014 x register r0 x = y + z; y memory (spill to stack) … Schulte, SCALE Schulte, • Code generation organized into stages • instruction selection, register allocation, CodeGeneration Rethinking 6 State-of-the-art instruction scheduling Oct Oct 1, 2014 x = y + z; u = v – w; … … u = v – w; x = y + z; Schulte, SCALE Schulte, • Code generation organized into stages • instruction selection, register allocation, instruction scheduling CodeGeneration Rethinking 7 State-of-the-art instruction register instruction selection allocation scheduling Oct Oct 1, 2014 • Code generation organized into stages • stages are interdependent: no optimal order possible Schulte, SCALE Schulte, Rethinking Code Generation CodeGeneration Rethinking 8 State-of-the-art instruction instruction register selection scheduling allocation Oct Oct 1, 2014 • Code generation organized into stages • stages are interdependent: no optimal order possible SCALE Schulte, • Example: instruction scheduling register allocation • increased delay between instructions can increase throughput Rethinking Code Generation CodeGeneration Rethinking registers used over longer time-spans more registers needed 9 State-of-the-art instruction register instruction selection allocation scheduling Oct Oct 1, 2014 • Code generation organized into stages • stages are interdependent: no optimal order possible SCALE Schulte, • Example: instruction scheduling register allocation • put variables into fewer registers Rethinking Code Generation CodeGeneration Rethinking more dependencies among instructions less opportunity for reordering instructions 10 State-of-the-art instruction instruction register selection scheduling allocation Oct Oct 1, 2014 • Code generation organized into stages • stages are interdependent: no optimal order possible SCALE Schulte, • Stages use heuristic algorithms • for hard combinatorial problems (NP hard) Rethinking Code Generation CodeGeneration Rethinking • assumption: optimal solutions not possible anyway • difficult to take advantage of processor features • error-prone when adapting to change 11 State-of-the-art instruction instruction register selection scheduling allocation Oct Oct 1, 2014 • Code generation organized into stages • stages are interdependent: no optimal order possible SCALE Schulte, • Stages use heuristic algorithms • for hard combinatorial problemspreclude (NP hard) optimal code, Rethinking Code Generation CodeGeneration Rethinking • assumption: optimal solutions makenot possible development anyway • difficult to take advantage of processorcomplex features • error-prone when adapting to change 12 Rethinking: Unison Idea • No more staging and heuristic algorithms! • many assumptions are decades old... • Use state-of-the-art technology for solving combinatorial Oct 1, 2014 optimization problems: constraint programming • tremendous progress in last two decades... SCALE Schulte, • Generate and solve single model • captures all code generation tasks in unison Rethinking Code Generation CodeGeneration Rethinking • high-level of abstraction: based on processor description • flexible: ideally, just change processor description • potentially optimal: tradeoff between decisions accurately reflected 13 Unison Approach instruction selection constraint model constraints input instruction constraints program scheduling constraints Oct 1, 2014 register allocation Schulte, SCALE Schulte, processor description • Generate constraint model CodeGeneration Rethinking • based on input program and processor description • constraints for all code generation tasks • generate but not solve: simpler and more expressive 14 Unison Approach instruction selection constraint model constraints off-the-shelf input instruction constraint constraints program scheduling solver constraints Oct 1, 2014 register allocation assembly program Schulte, SCALE Schulte, processor description • Off-the-shelf constraint solver solves constraint model CodeGeneration Rethinking • solution is assembly program • optimization takes inter-dependencies into account 15 Overview • Constraint programming in a nutshell • Approach Oct 1, 2014 • Results Schulte, SCALE Schulte, • Discussion Rethinking Code Generation CodeGeneration Rethinking 16 Oct Oct 1, 2014 Schulte, SCALE Schulte, Rethinking Code Generation CodeGeneration Rethinking CONSTRAINT PROGRAMMING 17 IN A NUTSHELL Constraint Programming • Model and solve combinatorial (optimization) problems • Modeling • variables Oct 1, 2014 • constraints • branching heuristics • (cost function) SCALE Schulte, • Solving • constraint propagation Rethinking Code Generation CodeGeneration Rethinking • heuristic search • Of course simplified… 18 • array of modeling techniques Problem: Send More Money • Find distinct digits for letters such that SEND Oct 1, 2014 + MORE Schulte, SCALE Schulte, = MONEY Rethinking Code Generation CodeGeneration Rethinking 19 Constraint Model • Variables: S,E,N,D,M,O,R,Y {0,…,9} • Constraints: Oct 1, 2014 distinct(S,E,N,D,M,O,R,Y) 1000×S+100×E+10×N+D SCALE Schulte, + 1000×M+100×O+10×R+E = 10000×M+1000×O+100×N+10×E+Y CodeGeneration Rethinking S0 M0 20 Constraints • State relations between variables • legal combinations of values for variables • Examples Oct 1, 2014 • all variables pair wise distinct: distinct(x1, …, xn) • arithmetic constraints: x + 2×y = z • domain-specific: cumulative(t , …, t ) 1 n SCALE Schulte, nooverlap(r1, …, rn) Rethinking Code Generation CodeGeneration Rethinking • Success story: global constraints • modeling: capture recurring problem structures • solving: enable strong reasoning 21 constraint-specific methods Solving: Variables and Values Oct Oct 1, 2014 x{1,2,3,4} y{1,2,3,4} z{1,2,3,4} Schulte, SCALE Schulte, Rethinking Code Generation CodeGeneration Rethinking • Record possible values for variables • solution: single value left • failure: no values left 22 Constraint Propagation distinct(x, y, z) x + y = 3 Oct 1, 2014 x{1,2,3,4} y{1,2,3,4} z{1,2,3,4} Schulte, SCALE Schulte, Rethinking Code Generation CodeGeneration Rethinking • Prune values that are in conflict with constraint 23 Constraint Propagation distinct(x, y, z) x + y = 3 Oct 1, 2014 x{1,2} y{1,2} z{1,2,3,4} Schulte, SCALE Schulte, Rethinking Code Generation CodeGeneration Rethinking • Prune values that are in conflict with constraint 24 Constraint Propagation distinct(x, y, z) x + y = 3 Oct 1, 2014 x{1,2} y{1,2} z{3,4} Schulte, SCALE Schulte, Rethinking Code Generation CodeGeneration Rethinking • Prune values that are in conflict with constraint • propagation is often smart if not perfect! • captured by so-called global constraints 25 Heuristic Search distinct(x, y, z) x + y = 3 x=1 x{1,2} y{1,2} z{3,4} x≠1 distinct(x, y, z) x + y = 3 distinct(x, y, z) x + y = 3 Oct 1, 2014 x{1} y{2} z{3,4} x{2} y{1} z{3,4} Schulte, SCALE Schulte, • Propagation alone not sufficient decompose into simpler sub-problems • CodeGeneration Rethinking • search needed • Create subproblems with additional constraints • enables further propagation • defines search tree 26 • uses problem specific heuristic What Makes It Work? • Essential: avoid search... ...as it always suffers from combinatorial explosion • Constraint propagation drastically reduces search space Oct 1, 2014 • Efficient and powerful methods for propagation available SCALE Schulte, • When using search, use a clever heuristic Rethinking Code Generation CodeGeneration Rethinking • Array of modeling techniques available that reduce search • adding redundant constraints for more propagation, symmetry breaking, ... 27 • Hybrid methods (together with LP, SAT, stochastic, ...) Approach Source Material • Survey on Combinatorial Register Allocation and Instruction Scheduling • Roberto Castañeda Lozano, Christian Schulte. CoRR entry, 2014. • Combinatorial Spill Code Optimization and Ultimate Oct 1, 2014 Coalescing • Roberto Castañeda Lozano, Mats Carlsson, Gabriel Hjort Blindell, Christian Schulte. Languages, Compilers, Tools and Theory for Embedded Systems, 2014. SCALE Schulte, •
Details
-
File Typepdf
-
Upload Time-
-
Content LanguagesEnglish
-
Upload UserAnonymous/Not logged-in
-
File Pages60 Page
-
File Size-