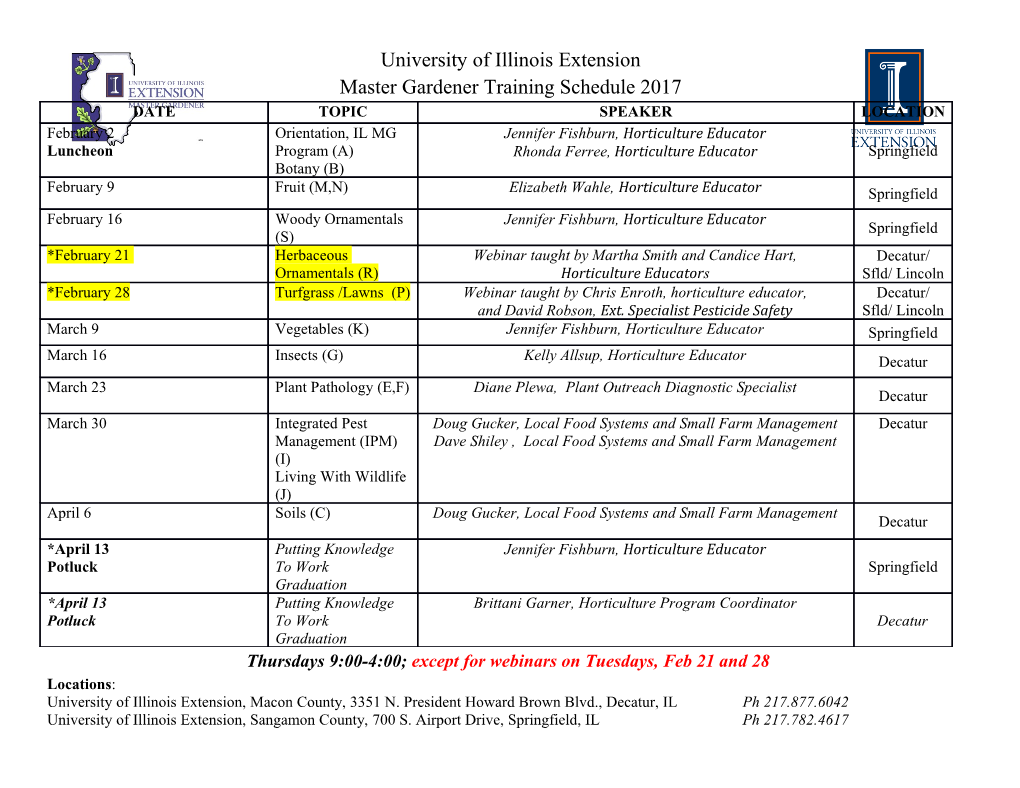
Int J Disaster Risk Sci (2015) 6:207–215 www.ijdrs.com DOI 10.1007/s13753-015-0059-0 www.springer.com/13753 ARTICLE Crop Insurance Premium Ratemaking Based on Survey Data: A Case Study from Dingxing County, China 1,2,3 2,3 1,4,5 1,4 Xingming Zhang • Weixia Yin • Jun Wang • Tao Ye • 6 1,2,3 Jintao Zhao • Jing’ai Wang Published online: 14 August 2015 Ó The Author(s) 2015. This article is published with open access at Springerlink.com Abstract Premium ratemaking is an important issue to regions that lack sufficient long-term yield data, especially guarantee insurance balance of payments. Most ratemaking in developing countries with smallholder agriculture. methods require large samples of long-term loss data or farm-level yield data, which are often unavailable in Keywords Agricultural risk Á Characteristic developing countries. This study develops a crop insurance agricultural yield Á Crop insurance Á North ratemaking method with survey data. The method involves China Á Premium ratemaking a questionnaire survey on characteristic yield information (average yield, high yield, and low yield) of farming households’ cropland. After compensating for random error, the probability distributions of farm-level yields are 1 Introduction simulated with characteristic yields based on the linear additive model. The premium rate is calculated based on Crop insurance plays an important role in providing Monte Carlo yield simulation results. This method was farmers with protection against catastrophic yield shortfalls applied to Dingxing County, North China to arrive at the (Charpentier 2008). The level of risk involved usually is insurance loss cost ratio and calculate the necessary pre- quantitatively reflected in the size of the crop insurance mium rate. The method proposed in this study could serve premium. Individual-yield crop insurance is the most as a feasible technique for crop insurance ratemaking in widely used and has usually been priced on the basis of long-term loss data recorded by insurance companies or long-term individual yield records. With long-term loss & Jing’ai Wang data, the loss cost ratio (LCR), or pure premium rate, is [email protected] calculated through simple division calculation. The nec- 1 essary data include the long-term operational history of State Key Laboratory of Earth Surface Processes and agricultural insurance and constant or similar production Resource Ecology, Beijing Normal University, Beijing 100875, China across time (Josephson et al. 2000). The premium rate also can be determined through integration of the probability 2 School of Geography, Beijing Normal University, Beijing 100875, China distribution simulated with yield records (Ozaki et al. 2008). Regional yield data, for example, are used to price 3 Key Laboratory of Regional Geography, Beijing Normal University, Beijing 100875, China crop insurance schemes, where indemnity and premium are based on the regional yield (Miranda 1991; Skees et al. 4 Academy of Disaster Reduction and Emergency Management, Beijing Normal University, Beijing 100875, 1997). But it is extremely difficult to obtain long series of China yield records at the farm level to estimate the distribution 5 People’s Insurance Company of China, Beijing 100022, of yields of individual smallholder farms with a sufficient China degree of confidence. The situation is even worse in 6 School of Management, Langfang Teachers College, developing countries with smallholder agriculture. There Langfang 065000, China are very few cases where high-quality farm yield series are 123 208 Zhang et al. Crop Insurance Premium Ratemaking Based on Survey Data available to support yield simulation based rating. Thus yjt in the following array (Koundouri and Kourogenis more appropriate data and ratemaking methods are 2011): 2 3 urgently needed. t ¼ 1 t ¼ 2 ... The existing literature suggests several methods using 6 7 6 j ¼ 1 y11 y12 ...7 available regional yields to price farm-level insurance. Botts 6 7 6 j ¼ 2 y21 y22 ...7 6 7 and Boles (1958) assumed normal distribution of farm yields 6 ::: 7 ð1Þ 6 7 around the county average yield with a certain standard 6 ::: 7 deviation. The method was challenged because of its normal 4 :::...5 distribution assumption (Nelson 1990) and constant devia- j ¼ NyN1 yN2 tion (Skees and Reed 1986). Also, the relationship between regional yield and individual farm yields is very complicated According to the indemnity calculation formula in the (Wang and Zhang 2003). There is some research on insurance contract (PICC 2011), the county annual LCR at year t is derived as follows: ratemaking with yield data of only a few years (Nelson P ÂÃ 1990). However, short-term series data do not fully charac- N max l À y ; 0 j¼1 P j jt terize crop yield risks (Coble et al. 2010). For example, LCRt ¼ N 8yjt C Â lj ð2Þ l farmers are required to report 4–10 years of individual yield j¼1 j records when buying actual production history (APH) C is the crop insurance coverage level. lj is the average products. However, the risk management agency (RMA) of yield of specific acre j. The average LCR in T year is the US Department of Agriculture (USDA) uses the reported calculated as a simple average of the annual loss rates. yield records to estimate the expected yield, instead of the P P T N yield distribution of individual farms (Schnapp et al. 2000). t¼1 j¼1 max½lj À yjt; 0 EðLCRÞ¼ P Regional yields and short-term series of farm-level yields do N T Â j¼1 lj not provide farm-level and long-term series information at P P ð3Þ N T max½l À y ; 0 the same time. So they cannot provide adequate input for j¼1 t¼1 P j jt ¼ N 8 yjt C Â lj ratemaking. Characteristic values carry more information T Â j¼1 lj than the same number of reported records by individual If the yield probability distributions of acres are known, farms. For example, the extremes, the upper and lower hin- across time (temporal properties), the expected LCR (pure ges (quartiles), and the median of a set of data are conven- premium rate) can be expressed as: tionally used in the configuration of a box plot to convey the R CÂyj characteristics of a distribution of data values (Mcgill et al. EðIÞ E½ ðl À yjtÞfjðÞyit dðyitÞ EðLCRÞ¼ ¼ 0 j ð4Þ 1978; Williamson et al. 1989). EðlÞ E½l This article presents a survey data based approach to price crop insurance in counties of China. Different from the APH EðLCRÞ is the expected LCR. EðIÞ is the expected that requires farmers to provide 4–10 years of actual yield data, indemnity. fj is the yield probability distribution of acre j. the method proposed here focuses on characteristic yield C Â yj is the yield coverage. The farmer whose yield is information—average yield, high yield, and low yield—of higher than C Â yj will not be paid by the insurance smallholder farms. As the memory of yields of specific past company. years becomes vague in the present, characteristic yield infor- In reality, long-term series of all farm-level yield mation is more reliable than reported yields for specific years, if records in regions (array 1) are difficult to obtain. So it both are recalled by farmers. Instead of using the reported data would be more applicable to calculate the premium rate directly, special treatment is employed to compensate for ran- with Eq. 4, based on the estimation of the farm-level yield dom error and ensure data validity. Section 2 presents the basic probability distribution. Here we try to do this based on the principle for premium ratemaking, Sect. 3 outlines the survey data obtained through a survey with a sample size of 486 that provided the data, Sect. 4 presents the steps of data pro- households. The survey data provide the information to cessing, and Sect. 5 outlines conclusions from the study. calculate the net rate. 2 Basic Principle for Premium Ratemaking 3 Farm-Level Yield Data from the Survey Let’s assume that the random variable of interest is yjt, Based on Eqs. 1–4 of Sect. 2, the key question for the production of a specific acre j at year t. Let N be the ratemaking is how to estimate the probability distributions total number of acres. We arrange the random variables of farm-level yields. Estimating this distribution with long- 123 Int J Disaster Risk Sci 209 term regional yield data (Botts and Boles 1958) has the county is 714 km2. The county has 16 towns with a total disadvantage of ignoring the variation of farm-level dis- area of 684 km2; an urban district; a provincial industrial tribution, although it incorporates considerations for tem- park; and 274 villages. Because agriculture is found only in poral variations. Using short-term series farm-level yield the villages, the study area does not cover the urban district records prohibits reliable characterization of the temporal and the provincial industrial park (Table 1). The total aspects of the distribution. Therefore this study proposes wheat planting area is about 33,300 ha, with an annual the method of estimating the distribution with a farming production of more than 200,000 tons. Agricultural income household survey of the long-term yield characteristics. is the main source of income for local farmers. The farming This section presents the design and execution of the 2011 income for the surveyed households ranged between RMB survey in the case study area of Dingxing County, Hebei 3,000 and 7,000 yuan, or about USD 480–1,100 (calculated Province, on the North China Plain. at the 2011 wheat price of 2.2 yuan per kg).
Details
-
File Typepdf
-
Upload Time-
-
Content LanguagesEnglish
-
Upload UserAnonymous/Not logged-in
-
File Pages9 Page
-
File Size-