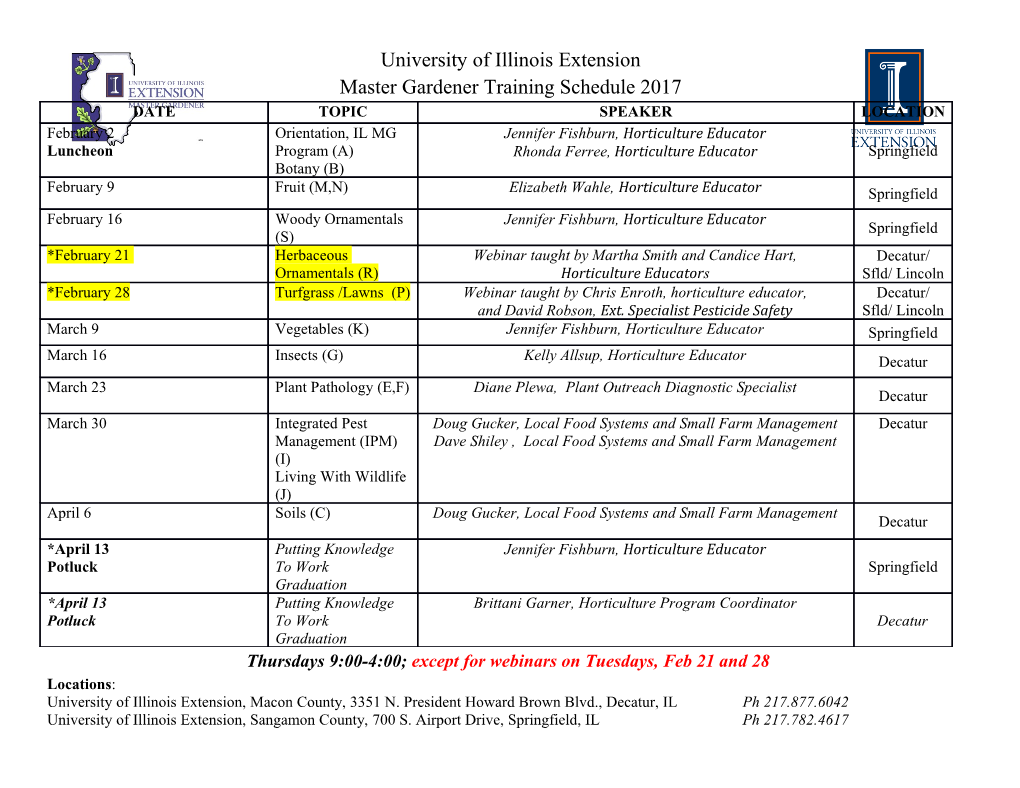
AN IMPROVED NORMALIZATION METHODOLOGY FOR DEVELOPING MEAN HUMAN RESPONSE CURVES Kevin Moorhouse National Highway Traffic Safety Administration United States Paper Number 13-0192 response curves should prove useful in more accurately characterizing the target human response ABSTRACT to aid in the design of more biofidelic dummies. Mean human response curves and associated INTRODUCTION biomechanical response targets are commonly developed from Post-Mortem Human Subject Biomechanical response corridors developed from (PMHS) test data to guide the design of human subject test data are commonly used to guide anthropomorphic test devices (ATDs) by providing the design of anthropomorphic test devices (ATDs) “target” biomechanical responses to impact. Since by providing “target” biomechanical responses to differences in anthropometry and physical impact. The biomechanical responses typically characteristics within a group of PMHS can result in consist of physical measures such as force, widely varying response data, the first step in acceleration, or deflection, and could be in the time- developing target biomechanical responses is domain or another domain (e.g., force-deflection). typically to normalize the responses to a certain The ability of an ATD to match these target “standard” anthropometry representing the ATD to responses defines its biofidelity. be designed or evaluated. The normalization procedure should adjust the response data to account The target responses are most often developed by for the variation in anthropometry and physical subjecting a group of Post-Mortem Human Subjects characteristics, and thus should collapse the group of (PMHS) to an impact or crash scenario, measuring curves closer to a single response so that a mean the resulting responses, and then representing each response can be more accurately established that group of responses such that it characterizes the represents the human response of the “standard” response of the selected population and can be used anthropometry selected. Several methods for to evaluate the biofidelity of the corresponding ATD normalizing PMHS test data can be found in the response. One way to accomplish this is to literature, but there is no consensus as to which is the encompass the entire group of response curves for a most effective. In this study, the two most common given measurement using straight-line segment existing normalization techniques, as well as some corridors, and then a biofidelic dummy response is newly developed methodologies, were evaluated by expected to lie entirely within that corridor applying them to both a side impact PMHS sled test (ISO/TR9790, 1999; Lobdell et al., 1973). Another data set, and a lateral and oblique pendulum side methodology is to reduce the group of responses into impact PMHS data set. The efficacy of the a single mean response curve, which itself represents normalization techniques were assessed for each the ATD design target (Cavanaugh et al., 1986; group of common signals by calculating the average Maltese et al., 2002; Morgan et al., 1986). In percent coefficient of variation (%CV) for time- addition to the mean response curve, standard history curves, and an analogous measure for force- deviation curves can be created around the mean to provide both a visual measure of the variation in the deflection curves (%CVellipse). Both of these measures provide a quantifiable assessment of the group of PMHS, as well as a quantitative measure of similitude of the group of curves (i.e., the that variation for assessing the biofidelity of an ATD. normalization technique resulting in the lowest Generally the standard deviation curves envelop the mean curve more tightly than the straight-line average %CV value or %CVellipse value most effectively collapses the curves). The normalization segment corridors, and there is no requirement that a technique found to consistently perform the best is a biofidelic ATD response must lie completely within newly developed extension of impulse momentum- the standard deviation curves. Representing the based normalization in which the stiffness ratio was target response using the mean response and standard determined from effective stiffness values calculated deviation curves is more appealing than straight-line from the test data, rather than using characteristic segment corridors because it provides a quantifiable lengths. Utilization of an improved normalization framework for assessing the biofidelity of an ATD methodology in the development of mean human Moorhouse 1 while maintaining the shape and characteristics of the Pelvis Force vs Time actual human response to impact. 9000 8000 Since there are a variety of ATDs, each representing 7000 a certain “standard” anthropometry or set of physical characteristics (i.e., 50th percentile male, 5th 6000 percentile female, etc.), it is important that the PMHS 5000 responses used to develop the design targets represent 4000 the same respective population. However, in reality (N) Force 3000 there is often large variation in the physical characteristics within a group of human subjects 2000 (e.g., size, shape, inertial properties, etc.) which 1000 results in widely varying response data. 0 Normalization is a procedure for mathematically -10 0 10 20 30 40 50 60 adjusting the response data to account for the Ti me (ms) variation in physical characteristics, and is often the first step in developing target biomechanical Figure 1. Mean curve resulting from two out-of-phase responses. Successful normalization should collapse responses the group of curves closer to a single response so that a mean response can be more accurately established that represents that of the selected population. developed based on a dimensional analysis approach to geometric scaling in which three scaling ratios Anthropometric variation between subjects such as containing the fundamental dimensions of mass, differences in height and weight can obviously affect length, and time must be defined in order to derive the magnitude of the response data, but differences in scaling ratios for other engineering variables of factors such as body mass distribution (i.e., fat-to- interest. Two of these ratios were defined to assume muscle ratio) can not only affect the magnitude but constant density and modulus of elasticity among also affect the phase or timing of the response data, subjects; the third ratio is the total body mass ratio: which is especially critical to the creation of a mean ρ E M response curve. Figure 1 shows an example of two 1 = 50th 1 = 50th λ = 50th (1) ρ curves (blue and red) which are out of phase but sub Esub M sub similar in shape and magnitude. The resulting mean curve (black) is bimodal, much lower in magnitude, where ρ is density, E is the modulus of elasticity, and has a shape nothing like either of the individual curves. Ideally, normalization would be able to M is total body mass, λ is the total body mass th account for the variation between the subjects which scaling ratio, and the subscripts “50 ” and “sub” caused this out-of-phase response, thus resulting in a represent the “standard” subject and test subject, more representative mean response curve. respectively. Note that since the most common th “standard” subject is the 50 percentile male it will th Although several methods for normalizing human hereafter be referred to with the subscript “50 ”, and subject test data can be found in the literature, the M 50th can be easily obtained from anthropometric two most commonly implemented procedures are tables. mass-based normalization as described by Eppinger (1984), and impulse momentum-based normalization Normalizing factors for engineering variables of as described for single mass systems (e.g., sled & interest (L = length or deflection, F = force, drop tests) by Mertz (1984) and for two-mass A = acceleration, T = time, and V = velocity) can then systems (e.g., pendulum tests) by Viano (1989). be derived from the three ratios in Equation (1) in conjunction with fundamental laws of physics, as Mass-based normalization shown in Equations (2) – (6): (Eppinger et al, 1984) M 1 The mass-based procedure normalizes human subject ρ = ⇒ L = λ 3 L (2) response data based solely on a mass ratio involving L3 50th sub the subject’s total body mass and the total body mass of the “standard” subject to which the responses are to be normalized. The underlying theory was Moorhouse 2 F 2 and then models the impacts as a simple spring-mass E = ⇒ F = λ 3 F (3) system: L2 50th sub m k λ = 50th λ = 50th m k (7) −1 m k F = MA ⇒ A = λ 3 A (4) sub sub 50th sub For the mass ratio, instead of a simple ratio of total L 1 body mass, an effective mass of the impacted body A = ⇒ T = λ 3T (5) T 2 50th sub region is estimated from the response data using an impulse momentum analysis as shown in Equation L (8): = = T V ⇒ V50th Vsub (6) T Fdt T = = ∫0 Fdt meff v0 ⇒ meff (8) ∫0 v The strengths of mass-based normalization are that it 0 is easy to implement, the procedure is independent of test condition (i.e., the same procedure is used for where meff is the effective mass, F is the force sled tests, drop tests, and pendulum tests), and the adjustment made to the response data is directly during impact, vo is the change in velocity during linked to the easy-to-obtain anthropometry measure the impact, and T is the duration of impact. By of total body mass. The fact that the response data is incorporating the response data in the normalization adjusted based solely on total body mass may make it procedure, some of the other causes for variation easy to implement, but it could also be considered a besides anthropometry (discussed earlier) can be weakness because measures of anthropometry alone somewhat accounted for. Unlike the total body mass are often poor predictors of response data.
Details
-
File Typepdf
-
Upload Time-
-
Content LanguagesEnglish
-
Upload UserAnonymous/Not logged-in
-
File Pages11 Page
-
File Size-