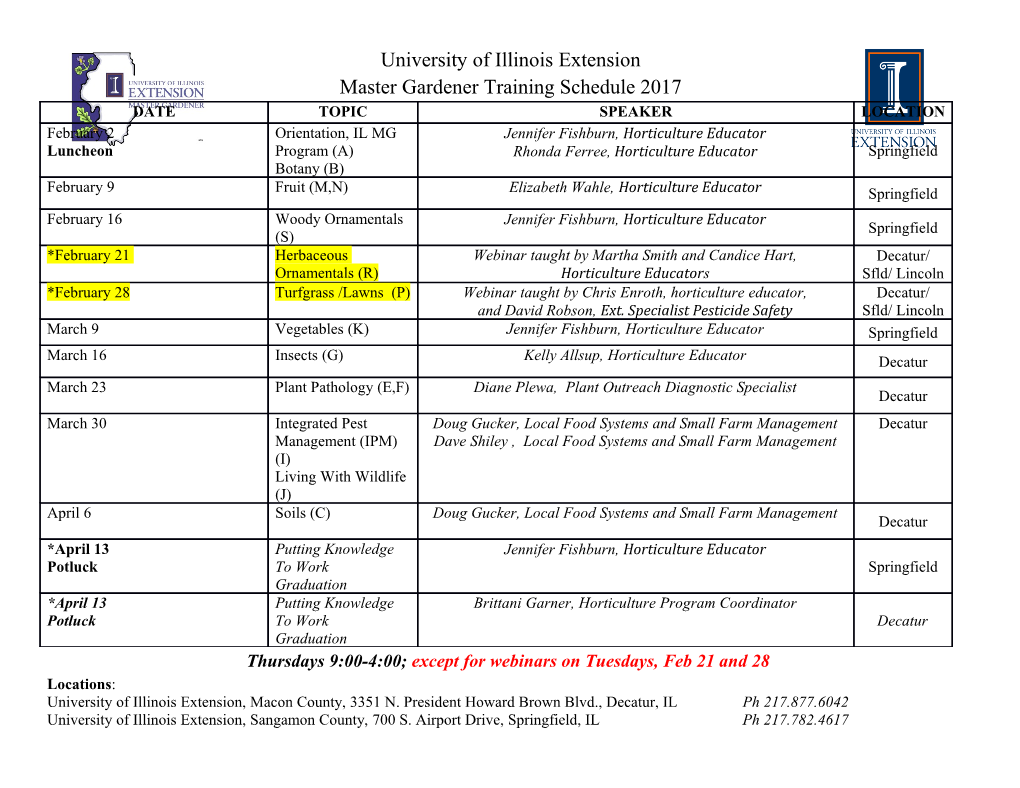
Forecasting with VARs with Long- and Short-Run Restrictions: A Monte-Carlo Study∗ Farshid Vahid† Osmani Teixeira de Carvalho Guill´en School of Economics Banco Central do Brasil Australian National University and Crisp Building 026 Graduate School of Economics — EPGE ACT 0200 Brazil Australia [email protected] Jo˜ao Victor Issler Graduate School of Economics — EPGE Getulio Vargas Foundation Praia de Botafogo 190 s. 1111 Rio de Janeiro, RJ 22253-900 Brazil [email protected] Preliminary version: July, 2004 Abstract Despite the commonly held belief that aggregate data display short-run comove- ment, there has been little discussion about the econometric consequences of this feature of the data. We use exhaustive Monte-Carlo simulations to investigate the importance of restrictions implied by common-cyclical features for estimates and fore- casts based on cointegrated vector autoregressive models. First, we show that the “best” empirical model developed without common cycle restrictions need not nest the “best” model developed with those restrictions. This is due to possible differences in the lag-lengths chosen by model selection criteria for the two alternative models. Second, we show that the costs of ignoring common cyclical features in vector au- toregressive modelling can be high, both in terms of forecast accuracy Third, we find that the Hannan-Quinn and the Scwarz criteria perform well among model selection criteria in simultaneously selecting the lag-length and rank of cointegrated vector au- toregressions. Keywords: Reduced rank models, model selection criteria, forecasting, variance de- composition. JEL Classification: C32, C53. ∗Acknowledgments: PartsosthispaperwerewrittenwhileOsmaniT.Guill´en was visiting Monash University, which hospitality is gratefully acknowledged. Jo˜ao Victor Issler and Osmani T. Guill´en ac- knowledges the support of CNPq-Brazil and PRONEX, and CAPES, respectively. †Corresponding author. 1 1Introduction Although vector autoregressive (VAR) and vector error-correction (VEC) models have become the “working horses” of macroeconometric studies, one of their shortcomings is the excessive number of parameters relative to the average sample size one is usually forced to work with. For example, when dealing with post-war quarterly data, and a VAR with three variables and eight lags, there are seventy five mean parameters to be estimated from about two hundred data points on each variable. One possible way of reducing the parameter space is to impose restrictions usually coming from cointegration, i.e., Engle and Granger(1987), Johansen(1991), and from common cycles, i.e., Engle and Kozicki(1993), Vahid and Engle(1993, 1997) and Hecq, Palm and Urbain(2004). The former are restriction on the long-run behavior of economic data and the latter are restrictions on their short-run behavior. The effects of imposing them in VAR models can be quite different. For example, Engle and Yoo(1987) show that imposing cointegration restrictions helps VAR forecasting accuracy only in long horizons, while Vahid and Issler(2002) show that serial-correlation- common-feature (SCCF) restrictions improves forecasting accuracy at all horizons. A well known result in econometrics is that unbiased restricted models produce better forecasts. Although imposing valid restrictions can indeed improve forecasting accuracy, researchers are usually aware of the fact that there is always the risk of imposing false re- strictions, which calls for conservative behavior: it is presumably better to use a possibly inefficient model instead of risking using an inconsistent model in the search for parsi- mony. Recent Monte-Carlo results in Vahid and Issler challenge this view with respect to VAR models with common cycles. They argue that the cost of ignoring common-cycle re- strictions is more than just the efficiency loss. This happens because the usual practice in applied work of choosing lag length by information criteria will severely underparameterize in this case. While common cycles imply a reduced-rank structure for coefficient matrices it implies no restrictions on the number of lags of a VAR. In this case, standard criteria find too small a lag length for VARs with common-cycle restrictions simply because this is the only possible way available to achieve parsimony. As a results, full-rank models chosen by information criteria are frequently misspecified and for such misspecified models one cannot tell from theory what the consequences of incorporating rank restrictions will be. In this paper we argue that SCCF restrictions should be taken seriously in VAR mod- elling, especially when these models are used for forecasting purposes. Our suggestion is based on a wide-scale Monte-Carlo exercise where data is generated by VARs containing simultaneously short-run and long-run restrictions: SCCF and cointegration restrictions respectively. As will become clear in the next sections, the Monte-Carlo design is far from trivial in this case, due to the fact that these two types of rank restrictions have to hold in every data generating process. In our simulations we cover three important issues on model building, estimation, and forecasting. First, we examine the behavior of standard information criteria in choosing lag length for cointegrated VARs with SCCF restrictions. Second, we provide a comparison of forecasting accuracy of fitted VARs when only cointegration restrictions are imposed and when cointegration and SCCF restrictions are jointly imposed. Finally, as a by- product of the latter exercise, we propose a new estimation algorithm where short- and long-run restrictions interact to estimate jointly the cointegrating and the cofeature spaces respectively. This algorithm follows closely the idea of weak-form reduced-rank structure, 2 suggested by Hecq, Palm and Urbain, where the reduced-rank structure of the lagged coefficient matrices in the vector error-correction model (VECM) is different from that of the adjustment coefficient matrix. In the first pass of our algorithm, only weak-form SCCF restrictions are imposed without imposing a priori any restrictions in cointegrating rank. Based on first pass estimates, the long-run impact matrix is estimated and short- and long-run restrictions interact to yield final VECM estimates. At the end, it is possible to conduct inference on cointegrating rank for the system. The focus of the simulations is the accuracy of multi-step ahead out-of-sample forecasts. We design them so that the results would be relevant for an applied macroeconomist estimating a relatively large number of parameters using a limited number of data points. To that end, we consider a variety of Data Generating Processes (DGPs) and sample sizes, that are similar to the “typical” data sets that applied researchers encounter in practice. The current study extends the work of Vahid and Issler(2002) in two dimensions. First, the unrestricted model being considered here is a cointegrated VAR, i.e., a VECM, whereas in Vahid and Issler the unrestricted model was just a VAR with no cointegration restric- tions. As will become apparent below, selecting a large number data genrating processes (DGPs) for VARs with long- and short-run restrictions is far from trivial, and being able to do it makes the results of our Monte-Carlo exercise more robust. Morevover, consid- ering cointegration restrictions is also more realistic because cointegration is a common occurrence in macroeconomic models and data. Therefore, our current study not only adds to what is currently known about SCCF restrictions but it also adds relevant infor- mation which has a wider application. Second, we propose a new estimation algorithm, where short- and long-run restrictions interact to estimate jointly the cointegrating and the cofeature spaces respectively. because of its performance in small samples, we believe that it has the potential to form the basis of a future estimators for VECM coefficients in the presence of SCCF restrictions. The results of our simulation exercise and those of previous studies on SCCF restric- tions in VAR analysis call for a change in focus in the literature on forecasting with these models. While in the past there has been a considerable effort examining the importance of cointegration restrictions in VAR models — see, among others, Engle and Yoo(1987), Clements and Hendry(1995), and Lin and Tsay(1996) — there has been little work examin- ing the importance of common-cyclical features in VAR analysis. However, the simulation results in Vahid and Issler and the enriched environment considered here show a superior forecasting performance for models incorporating SCCF restrictions, which happens irre- spective of whether cointegration is or is not present in the data and of the forecasting horizon under study. The outline of the paper is as follows. Section 2 states the reduced-rank restrictions that common cyclical fluctuations impose on the parameters of cointegrated VAR models, and presents the model-selection criteria for reduced-rank models; see also the discussion in the Appendix on these issues. Section 3 describes our Monte-Carlo design; see also the discussion in the Appendix on DGP selection. Section 4 presents the simulation results. Section 5 presents the main conclusions of the paper, as well as a suggestion for further research. 3 2 Forecasting VECMs with SCCF restrictions As in most applied macroeconomic research, we assume that the objective is to build a time series model for the growth rate of a vector of n economic variables. We denote the levels of these variables at time t by Yt, their logarithms by yt, and their growth rates (i.e. the first difference of the logarithm of Yt)by∆yt. We make the reasonable assumption that ∆yt is stationary, add the simplifying assumption that ∆yt has mean zero (without any loss of generality), and start with the Wold representation of ∆yt,i.e. ∆yt = C (L) εt, (1) j where C (L)= j∞=0 CjL is a matrix polynomial in the lag operator and C0 = In.From the work of Beveridge and Nelson (1981) and Stock and Watson (1988), it is possible P to decompose the log-level series yt into common trends and cycles (we refer to this as the Beveridge-Nelson-Stock-Watson — or BNSW — decomposition).
Details
-
File Typepdf
-
Upload Time-
-
Content LanguagesEnglish
-
Upload UserAnonymous/Not logged-in
-
File Pages26 Page
-
File Size-