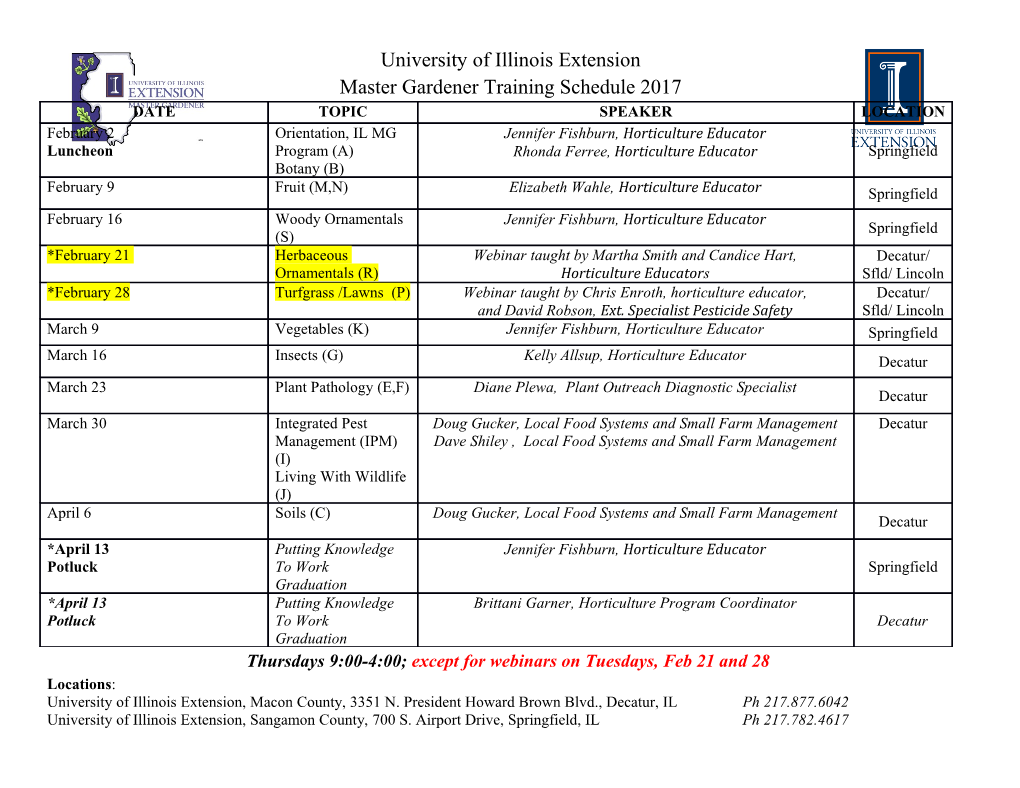
MINLP Solver Technology Stefan Vigerske October 24, 2017 Outline Introduction Fundamental Methods Recap: Mixed-Integer Linear Programming Convex MINLP Nonconvex MINLP Bound Tightening Acceleration { Selected Topics Optimization-based bound tightening Synergies with MIP and NLP Convexity Convexification Primal Heuristics 2/42 Introduction Mixed-Integer Nonlinear Programs (MINLPs) min cTx s.t. gk (x) ≤ 0 8k 2 [m] xi 2 Z 8i 2 I ⊆ [n] xi 2 [`i ; ui ] 8i 2 [n] 1 The functions gk 2 C ([`; u]; R) can be 0 100 200 10 200 300 0 200 5 or 0 −200 −1 1 −1 1 convex nonconvex 3/42 General MINLP = Convex MINLP plus Global Optimization: • Main difficulty: Nonconvex nonlinearities • Main challenges: • Convexification of nonconvex nonlinearities • Reduction of convexification gap (spatial branch-and-bound) • Numerical robustness • Diversity of problem class: MINLP is \The mother of all determinstic optimization problems" (Jon Lee, 2008) Solving MINLPs Convex MINLP: • Main difficulty: Integrality restrictions on variables • Main challenge: Integrating techniques for MIP (branch-and-bound) and NLP (SQP, interior point, Kelley' cutting plane, . ) 4/42 Solving MINLPs Convex MINLP: • Main difficulty: Integrality restrictions on variables • Main challenge: Integrating techniques for MIP (branch-and-bound) and NLP (SQP, interior point, Kelley' cutting plane, . ) General MINLP = Convex MINLP plus Global Optimization: • Main difficulty: Nonconvex nonlinearities • Main challenges: • Convexification of nonconvex nonlinearities • Reduction of convexification gap (spatial branch-and-bound) • Numerical robustness • Diversity of problem class: MINLP is \The mother of all determinstic optimization problems" (Jon Lee, 2008) 4/42 Solvers for Convex MINLP solver citation OA NLP-BB LP/NLP AlphaECP Westerlund and Lundquist [2005], Las- ECP tusilta [2011] AOA Roelofs and Bisschop [2017] (AIMMS) X Bonmin Bonami, Biegler, Conn, Cornu´ejols, XXX Grossmann, Laird, Lee, Lodi, Margot, Sawaya, and W¨achter[2008] DICOPT Kocis and Grossmann [1989] X FilMINT Abhishek, Leyffer, and Linderoth [2010] X Knitro Byrd, Nocedal, and Waltz [2006] X MINLPBB Leyffer [1998] X MINOTAUR Mahajan, Leyffer, and Munson [2009] (X) SBB Bussieck and Drud [2001] X XPRESS-SLP FICO [2008] ECP X . • can often work as heuristic for nonconvex MINLP 5/42 Couenne 2008 Belotti, Lee, Liberti, Margot, and W¨achter[2009] LindoAPI 2009 Lin and Schrage [2009] SCIP 2012 Achterberg [2009], Vigerske and Gleixner [2017], Maher, Fischer, Gally, Gamrath, Gleixner, Gottwald, Hendel, Koch, L¨ubbecke, Miltenberger, M¨uller,Pfetsch, Puchert, Rehfeldt, Schenker, Schwarz, Serrano, Shi- nano, Weninger, Witt, and Witzig [2017] ANTIGONE 2013 Misener and Floudas [2014] Main restriction: algebraic structure of problem must be available (see later) Interval-Arithmetic based: avoid round-off errors, typically NLP only, e.g., COCONUT [Neumaier, 2001], Ibex, . Stochastic search: LocalSolver, OQNLP [Ugray, Lasdon, Plummer, Glover, Kelly, and Mart´ı,2007],... Solvers for General MINLP Deterministic: solver 1st ver. citation αBB 1995 Adjiman, Androulakis, and Floudas [1998a] BARON 1996 Sahinidis [1996], Tawarmalani and Sahinidis [2005] in gPROMS 1996 Smith and Pantelides [1997] 6/42 Interval-Arithmetic based: avoid round-off errors, typically NLP only, e.g., COCONUT [Neumaier, 2001], Ibex, . Stochastic search: LocalSolver, OQNLP [Ugray, Lasdon, Plummer, Glover, Kelly, and Mart´ı,2007],... Solvers for General MINLP Deterministic: solver 1st ver. citation αBB 1995 Adjiman, Androulakis, and Floudas [1998a] BARON 1996 Sahinidis [1996], Tawarmalani and Sahinidis [2005] in gPROMS 1996 Smith and Pantelides [1997] Couenne 2008 Belotti, Lee, Liberti, Margot, and W¨achter[2009] LindoAPI 2009 Lin and Schrage [2009] SCIP 2012 Achterberg [2009], Vigerske and Gleixner [2017], Maher, Fischer, Gally, Gamrath, Gleixner, Gottwald, Hendel, Koch, L¨ubbecke, Miltenberger, M¨uller,Pfetsch, Puchert, Rehfeldt, Schenker, Schwarz, Serrano, Shi- nano, Weninger, Witt, and Witzig [2017] ANTIGONE 2013 Misener and Floudas [2014] Main restriction: algebraic structure of problem must be available (see later) 6/42 Solvers for General MINLP Deterministic: solver 1st ver. citation αBB 1995 Adjiman, Androulakis, and Floudas [1998a] BARON 1996 Sahinidis [1996], Tawarmalani and Sahinidis [2005] in gPROMS 1996 Smith and Pantelides [1997] Couenne 2008 Belotti, Lee, Liberti, Margot, and W¨achter[2009] LindoAPI 2009 Lin and Schrage [2009] SCIP 2012 Achterberg [2009], Vigerske and Gleixner [2017], Maher, Fischer, Gally, Gamrath, Gleixner, Gottwald, Hendel, Koch, L¨ubbecke, Miltenberger, M¨uller,Pfetsch, Puchert, Rehfeldt, Schenker, Schwarz, Serrano, Shi- nano, Weninger, Witt, and Witzig [2017] ANTIGONE 2013 Misener and Floudas [2014] Main restriction: algebraic structure of problem must be available (see later) Interval-Arithmetic based: avoid round-off errors, typically NLP only, e.g., COCONUT [Neumaier, 2001], Ibex, . Stochastic search: LocalSolver, OQNLP [Ugray, Lasdon, Plummer, Glover, Kelly, and Mart´ı,2007],... 6/42 Global MINLP Solver Progress: # Solved Instances and Solving Time 100 virt.best ANTIGONE BARON COUENNE LINDO API SCIP 80 60 40 % solved instances 20 0 800 600 Overall speedup (virtual best solver): 15.12 400 200 0 mean solving time 2011 2012 2013 2014 2015 2016 2017 date ANTIGONE BARON COUENNE LINDO API SCIP • 71 \non-trivial solvable" instances from MINLPLib 08/2011 { 9.3.1 0.3 6.1.1.588 { 11/2012 { 11.5.2 0.4 7.0.1.497 2.1.2 • time limit: 1800 seconds, gap limit: 1e-6 07/2013 1.1 12.3.3 0.4 8.0.1283.385 3.0 09/2014 1.1 14.0.3 0.4 8.0.1694.550 3.1 11/2015 1.1 15.9.22 0.5 9.0.2120.225 3.2 09/2016 1.1 16.8.24 0.5 9.0.2217.293 3.2 08/2017 1.1 17.8.7 0.5 11.0.3742.269 4.0 7/42 Fundamental Methods Fundamental Methods Recap: Mixed-Integer Linear Programming MIP Branch & Cut For mixed-integer linear programs (MIP), that is, min cTx; s.t. Ax ≤ b; xi 2 Z; i 2 I; the dominant method of Branch & Cut combines 3 2 1 & 1 2 3 4 cutting planes branch-and-bound [Gomory, 1958] [Land and Doig, 1960] 8/42 Fundamental Methods Convex MINLP • However: Robustness and Warmstarting-capability of NLP solvers not as good as for LP solvers (simplex alg.) NLP-based Branch & Bound (NLP-BB) ) MIP branch-and-bound MINLP branch-and-bound [Land and Doig, 1960] [Leyffer, 1993] Bounding: Solve convex NLP relaxation obtained by dropping integrality requirements. Branching: Subdivide problem along variables xi , i 2 I, that take fractional value in NLP solution. 9/42 NLP-based Branch & Bound (NLP-BB) ) MIP branch-and-bound MINLP branch-and-bound [Land and Doig, 1960] [Leyffer, 1993] Bounding: Solve convex NLP relaxation obtained by dropping integrality requirements. Branching: Subdivide problem along variables xi , i 2 I, that take fractional value in NLP solution. • However: Robustness and Warmstarting-capability of NLP solvers not as good as for LP solvers (simplex alg.) 9/42 Reduce Convex MINLP to MIP Assume all functions gk (·) of MINLP are convex on [`; u]. Duran and Grossmann [1986]: MINLP and Example: the following MIP have the same optimal min x + y solutions s.t. (x; y) 2 ellipsoid min cTx; x 2 f0; 1; 2; 3g T y 2 [0; 3] s.t. gk (^x) + rgk (^x) (x − x^) ≤ 0; k 2 [m]; x^ 2 R; xi 2 Z; i 2 I; x 2 [`; u]; wherex ^ 2 R are the solutions of the NLP subproblems obtained from MINLP by applying any possible fixing for xI , i.e., T min c x s.t. g(x) ≤ 0; x 2 [`; u]; xI fixed: 10/42 Reduce Convex MINLP to MIP Assume all functions gk (·) of MINLP are convex on [`; u]. Duran and Grossmann [1986]: MINLP and Example: the following MIP have the same optimal min x + y solutions s.t. (x; y) 2 ellipsoid min cTx; x 2 f0; 1; 2; 3g T y 2 [0; 3] s.t. gk (^x) + rgk (^x) (x − x^) ≤ 0; k 2 [m]; x^ 2 R; xi 2 Z; i 2 I; x 2 [`; u]; wherex ^ 2 R are the solutions of the NLP subproblems obtained from MINLP by applying any possible fixing for xI , i.e., T min c x s.t. g(x) ≤ 0; x 2 [`; u]; xI fixed: 10/42 Reduce Convex MINLP to MIP Assume all functions gk (·) of MINLP are convex on [`; u]. Duran and Grossmann [1986]: MINLP and Example: the following MIP have the same optimal min x + y solutions s.t. (x; y) 2 ellipsoid min cTx; x 2 f0; 1; 2; 3g T y 2 [0; 3] s.t. gk (^x) + rgk (^x) (x − x^) ≤ 0; k 2 [m]; x^ 2 R; xi 2 Z; i 2 I; x 2 [`; u]; wherex ^ 2 R are the solutions of the NLP subproblems obtained from MINLP by applying any possible fixing for xI , i.e., T min c x s.t. g(x) ≤ 0; x 2 [`; u]; xI fixed: 10/42 Reduce Convex MINLP to MIP Assume all functions gk (·) of MINLP are convex on [`; u]. Duran and Grossmann [1986]: MINLP and Example: the following MIP have the same optimal min x + y solutions s.t. (x; y) 2 ellipsoid min cTx; x 2 f0; 1; 2; 3g T y 2 [0; 3] s.t. gk (^x) + rgk (^x) (x − x^) ≤ 0; k 2 [m]; x^ 2 R; xi 2 Z; i 2 I; x 2 [`; u]; wherex ^ 2 R are the solutions of the NLP subproblems obtained from MINLP by applying any possible fixing for xI , i.e., T min c x s.t. g(x) ≤ 0; x 2 [`; u]; xI fixed: 10/42 NLP MIP NLP MIP MIP Outer Approximation Method (OA), ECP, EHP Convex MINLP MIP min cTx ≡ min cTx; T s.t. gk (x) ≤ 0 8k 2 [m] s.t. gk (^x) + rgk (^x) (x − x^) ≤ 0; xi 2 Z 8i 2 I ⊆ [n] 8k 2 [m]; x^ 2 R; xi 2 [`i ; ui ] 8i 2 [n] xi 2 Z; 8i 2 I; xi 2 [`i ; ui ]; 8i 2 [n] Outer Approximation (OA) algorithm [Duran and Grossmann, 1986]: • Start with R := ;.
Details
-
File Typepdf
-
Upload Time-
-
Content LanguagesEnglish
-
Upload UserAnonymous/Not logged-in
-
File Pages180 Page
-
File Size-