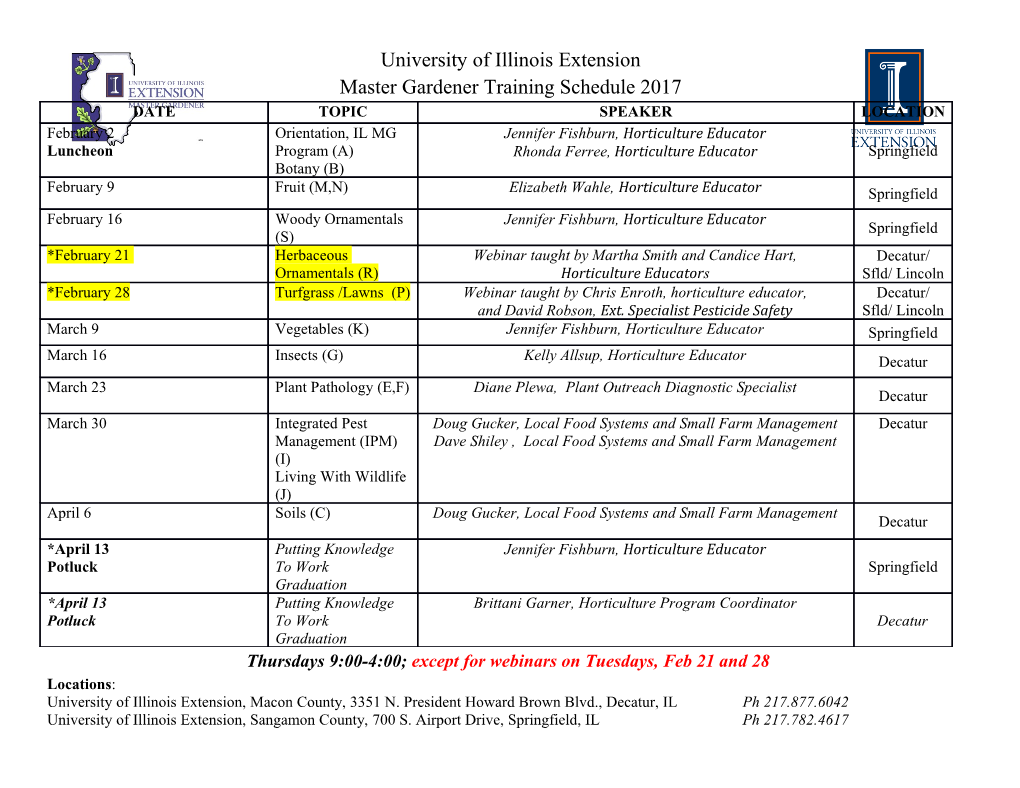
ISBN 978-82-326-2241-2 (electronic ver.) ISBN 978-82-326-2241-2(electronic ISBN 978-82-326-2240-5 (printed ver.) ISBN 978-82-326-2240-5(printed ISSN 1503-8181 ISSN Bilegsaikhan Naidan Bilegsaikhan Doctoral theses at NTNU, 2017:84 NTNU, at theses Doctoral NTNU Norwegian University of Science and Technology Thesis for the Degree of Philosophiae Doctor Faculty of Information Technology and Electrical Engineering Doctoral thesis Department of Computer Science Similarity Search Methods Similarity Search Engineering Efficient Naidan Bilegsaikhan thesesatNTNU,2017:84 Doctoral Bilegsaikhan Naidan Engineering Efficient Similarity Search Methods Thesis for the Degree of Philosophiae Doctor Trondheim, May 2017 Norwegian University of Science and Technology Faculty of Information Technology and Electrical Engineering Department of Computer Science NTNU Norwegian University of Science and Technology Thesis for the Degree of Philosophiae Doctor Faculty of Information Technology and Electrical Engineering Department of Computer Science © Bilegsaikhan Naidan ISBN 978-82-326-2240-5 (printed ver.) ISBN 978-82-326-2241-2 (electronic ver.) ISSN 1503-8181 Doctoral theses at NTNU, 2017:84 Printed by NTNU Grafisk senter Preface This thesis is submitted to the Norwegian University of Science and Technology (NTNU) in partial fulfillment of the requirements for the degree of philosophiae doctor. This doctoral work has been performed at the Department of Computer and Information Science, NTNU, Trondheim, with Associate Professor Magnus Lie Hetland as main supervisor and with co-supervisors Professor Svein Erik Bratsberg and Professor Arne Halaas. The thesis has been part of the research project Information Access Disruptions (iAd) project which is funded by the Norwegian Research Council. The main re- search of iAd project is to develop the next generation of search and the delivery technology in the information access domain. 3 Summary Similarity search has become one of the important parts of many applications in- cluding multimedia retrieval, pattern recognition and machine learning. In this problem, the main task is to retrieve most similar (closest) objects in a large data set to a query efficiently by using a function that gives the distance between two objects. This thesis is presented as a collection of seven papers with a tutorial on the topic and is mainly focused on efficiency issues of similarity search. • Paper I provides a survey on state-of-the-art approximate methods for met- ric and non-metric spaces. • Paper II proposes an approximate indexing method for the non-metric Breg- man divergences. • Paper III shows how to transform static indexing methods into dynamic ones. • Paper IV presents a learning method to prune in metric and non-metric spaces. • Paper V improves the existing approximate technique that can be applied to ball-based metric indexing methods. • Paper VI presents an open source cross-platform similarity search library and a toolkit called the “Non-Metric Space Library” (NMSLIB) for evalua- tion of similarity search methods. • Paper VII evaluates a metric index for approximate string matching. 5 Acknowledgements First and foremost, I would like to thank my supervisor Magnus Lie Hetland. He has always been available to discuss research ideas when I needed and has been supporting me throughout this PhD work and helping me overcome a personal hard time. He also taught me how to conduct experiments and write scientific papers. I would like to thank my supervisor Svein Erik Bratsberg for his support, research ideas and inspiring discussions. I would like also thank Professor Arne Halaas for being co-supervisor. Dr. Øystein Torbjørnsen has been an unofficial advisor to me. Despite being busy with his schedule, he led several fruitful meetings together with my supervisors when I struggled to produce publications. I would like to give a big thank to Leonid Boytsov for his collaboration and co- authoring several papers and co-authoring our research Non-Metric Space Li- brary and maintaining it. I would like to thank all people who contributed to the library. I would like to thank Professor Eric Nyberg for his support of our library. Finally, I would like to thank my family and they have been supporting me all time. 7 Contents Preface 3 Summary 5 Acknowledgements 7 I Introduction and Overview 11 1 Introduction 13 1.1 Motivation ................................. 13 1.2 Research goals ............................... 16 2 Background 17 2.1 Metric space model ............................ 17 2.2 Distance functions ............................. 18 2.3 Similarity queries ............................. 20 2.4 Problem description ........................... 21 2.5 Space partitioning principles ...................... 21 2.6 Pruning principles ............................ 22 2.7 Space decomposition methods ..................... 24 2.8 Approximate methods .......................... 26 2.9 Methods based on clustering principle ................. 30 2.10 Projection methods ............................ 30 2.11 Graph methods .............................. 37 2.12 Other methods ............................... 38 3 Research Summary 39 3.1 Research method ............................. 39 3.2 Included papers .............................. 40 3.2.1 Paper I ............................... 40 3.2.2 Paper II ............................... 41 3.2.3 Paper III .............................. 42 9 10 3.2.4 Paper IV .............................. 43 3.2.5 Paper V .............................. 43 3.2.6 Paper VI .............................. 44 3.2.7 Paper VII .............................. 45 3.3 Publication venue ............................. 45 3.4 Evaluation of contributions ....................... 46 3.5 NSMLIB in an approximate nearest neighbor benchmark ................................. 47 3.6 Future work ................................ 47 3.7 Conclusions ................................ 48 Bibliography 49 II Publications 55 A Paper I: Permutation Search Methods are Efficient, Yet Faster Search is Possible 57 B Paper II: Bregman hyperplane trees for fast approximate nearest neigh- bor search 73 C Paper III: Static-to-dynamic transformation for metric indexing struc- tures (extended version) 89 D Paper IV: Learning to Prune in Metric and Non-Metric Spaces 107 E Paper V: Shrinking data balls in metric indexes 121 F Paper VI: Engineering Efficient and Effective Non-metric Space Library 129 G Paper VII: An empirical evaluation of a metric index for approximate string matching 145 Part I Introduction and Overview 13 Chapter 1 Introduction Outline This thesis is a collection of papers with a basic introduction on similarity search. This chapter presents the motivation and research goals of this work. Chapter 2 describes the theoretical background and a tutorial on similarity search. Chapter 3 summarizes the results and reviews the papers. It also discusses future work and concludes the thesis. The research contribution of this thesis is defined by seven research papers which can be found in Part II. 1.1 Motivation Searching has become one of the crucial parts in our daily life and it allows us to find useful information. Traditional text-based search can not be directly ap- plied to more complex data types (such as multimedia data) which do not have any text information (for instance, caption, description and tags). So-called sim- ilarity search can be applied to those data types. In similarity search, domain objects (such as strings and multimedia data) are modeled in a metric or non- metric space and those objects are retrieved based on their similarity to some given query. For multimedia data, similarity search is often performed on the feature vectors extracted from the real data. Feature vectors are usually represented as high- dimensional data, so due to the so-called curse of dimensionality phenomenon, the efficiency of the indexing structures deteriorates rapidly as the number of di- mensions increases. This may be because there is a trend for the objects to be Some of the text and figures included in this thesis are based on my PhD plan which was partially included in a journal paper without my permission. 14 Introduction almost equidistant from the query objects in a high-dimensional space. Let us consider two use cases of similarity search in two different domains. Multimedia retrieval - In image retrieval, similar images to a query image are re- trieved by using image features such as shape, edge, position and color histogram. So-called the Signature Quadratic Form Distance [5] (SQFD) is shown to be effec- tive in image retrieval. In the method of Beecks [5], an image is mapped into a feature space and then its features are clustered with the standard k-means clus- tering algorithm. Each cluster is represented by a centroid and a cluster weight. Figure 1.1 shows three example images and the visualizations of their correspond- ing features as multiple circles. The centroid, weight and color of each cluster are represented by the center, diameter and color of a circle, respectively. We can see that the first two images are more similar to each other than the third one and that observation is also true for their feature signatures. Figure 1.1: Three example images at top and the corresponding visualizations of their feature signatures at bottom. Machine learning -Ink-nearest neighbor classification, a test instance needs to be assigned into one of the several classes. First, we need to find the k closest training instances of the test instance. Then, the most occurred
Details
-
File Typepdf
-
Upload Time-
-
Content LanguagesEnglish
-
Upload UserAnonymous/Not logged-in
-
File Pages146 Page
-
File Size-