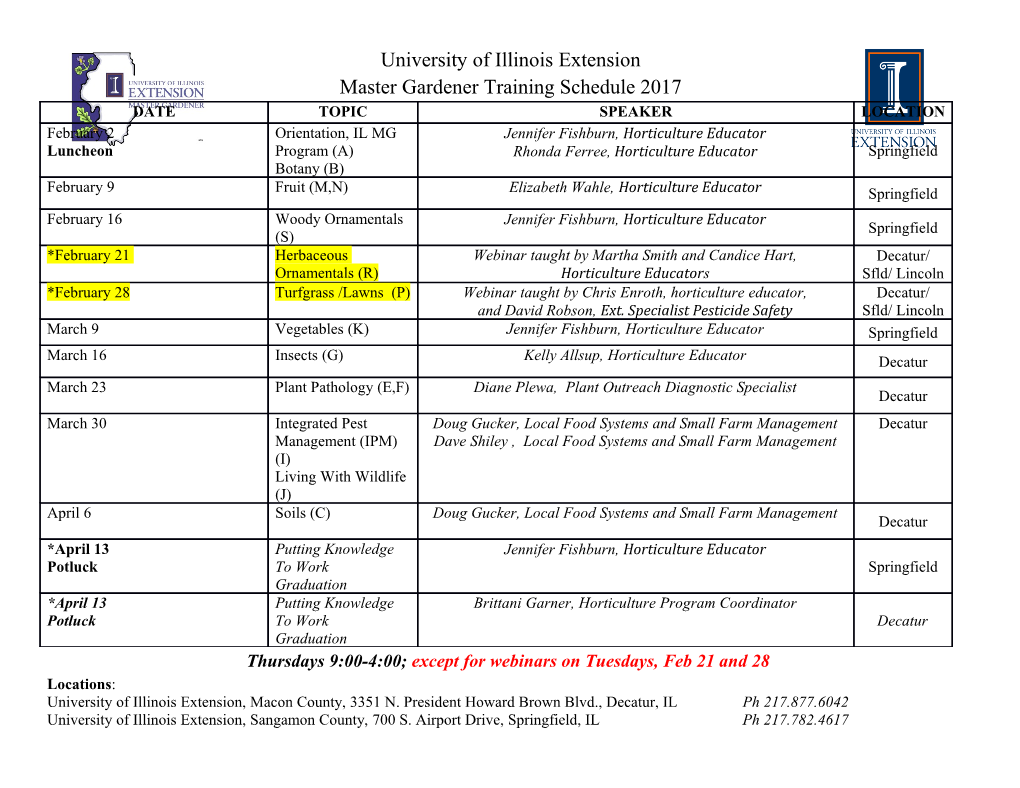
Wright State University CORE Scholar Browse all Theses and Dissertations Theses and Dissertations 2010 Understanding User-Generated Content on Social Media Bala Meenakshi Nagarajan Wright State University Follow this and additional works at: https://corescholar.libraries.wright.edu/etd_all Part of the Computer Engineering Commons, and the Computer Sciences Commons Repository Citation Nagarajan, Bala Meenakshi, "Understanding User-Generated Content on Social Media" (2010). Browse all Theses and Dissertations. 1008. https://corescholar.libraries.wright.edu/etd_all/1008 This Dissertation is brought to you for free and open access by the Theses and Dissertations at CORE Scholar. It has been accepted for inclusion in Browse all Theses and Dissertations by an authorized administrator of CORE Scholar. For more information, please contact [email protected]. Understanding User-Generated Content on Social Media Dissertation submitted in partial fulfillment of the requirements for the degree of Doctor of Philosophy By BALA MEENAKSHI NAGARAJAN (Signature of Student) 2010 Wright State University COPYRIGHT BY BALA MEENAKSHI NAGARAJAN 2010 WRIGHT STATE UNIVERSITY SCHOOL OF GRADUATE STUDIES August 18, 2010 I HEREBY RECOMMEND THAT THE DISSERTATION PREPARED UNDER MY SUPER- VISION BY Meenakshi Nagarajan ENTITLED Understanding User-generated Content on Social Media BE ACCEPTED IN PARTIAL FULFILLMENT OF THE REQUIREMENTS FOR THE DEGREE OF Doctor of Philosophy. Amit P. Sheth, Ph.D. Dissertation Director Arthur Ardeshir Goshtasby, Ph.D. Director, Computer Science and Engineering Ph.D. Program Andrew T. Hsu, Ph.D. Dean, School of Graduate Studies Committee on Final Examination John M. Flach, Ph.D. Daniel Gruhl, Ph.D. Michael L. Raymer, Ph.D. Shaojun Wang, Ph.D. Kevin Haas, M.S. ABSTRACT Nagarajan, Bala Meenakshi, Ph.D., Department of Computer Science and Engineering, Wright State University, 2010. Understanding User-generated Content on Social Media. Over the last few years, there has been a growing public and enterprise fascination with ‘social media’ and its role in modern society. At the heart of this fascination is the ability for users to participate, collaborate, consume, create and share content via a variety of platforms such as blogs, micro-blogs, email, instant messaging services, social network services, collaborative wikis, social bookmarking sites, and multimedia sharing sites. This dissertation is devoted to understanding informal user-generated textual content on social media platforms and using the results of the analysis to build Social Intelligence Applications. The body of research presented in this thesis focuses on understanding what a piece of user- generated content is about via two sub-goals of Named Entity Recognition and Key Phrase Ex- traction on informal text. In light of the poor context and informal nature of content on social media platforms, we investigate the role of contextual information from documents, domain mod- els and the social medium to supplement and improve the reliability and performance of existing text mining algorithms for Named Entity Recognition and Key Phrase Extraction. In all cases we find that using multiple contextual cues together lends to reliable inter-dependent decisions, better than using the cues in isolation and that such improvements are robust across domains and content of varying characteristics, from micro-blogs like Twitter, social networking forums such as those on MySpace and Facebook, and blogs on the Web. Finally, we showcase two deployed Social Intelligence applications that build over the results of Named Entity Recognition and Key Phrase Extraction algorithms to provide near real-time information about the pulse of an online populace. Specifically, we describe what it takes to build applications that wish to exploit the ‘wisdom of the crowds’ – highlighting challenges in data collection, processing informal English text, metadata extraction and presentation of the resulting information. iii Contents 1 1. Introduction 1 1.1 Dissertation Focus . 2 1.2 Dissertation Statements and Contributions . 5 1.2.1 Broader Impact . 6 1.3 Dissertation Organization . 8 2 2. Aboutness of Text and The Role of Context 9 2.1 Characterizing Aboutness . 9 2.2 The Role of Context . 13 2.2.1 The Formality of Language . 16 2.3 Communication on Social Media Platforms . 18 2.4 Aboutness Understanding in Informal Text . 20 3 3. Named Entity Recognition in Informal Text 25 3.1 Preliminaries . 26 3.1.1 NER is Challenging and Expensive . 27 3.1.2 Entity Types . 27 3.1.3 Techniques and Approaches . 28 3.1.4 Feature Space for NER . 30 3.1.5 Evaluation Metrics . 31 3.2 Thesis Focus - Cultural NER in Informal Text . 34 3.2.1 Entity Type - Cultural Named Entities . 35 3.2.2 The ‘Spot and Disambiguate’ Paradigm . 36 3.2.3 Two Approaches to Cultural NER . 37 3.2.4 Feature Space for Cultural NER . 39 3.3 Cultural NER – Multiple Senses across Domains . 41 3.3.1 A Feature Based Approach to Cultural NER . 41 3.3.2 Problem Definition . 42 3.3.3 Improving NER - Contributions . 43 3.3.4 Feature Extraction . 45 3.3.4.1 Problem Setup . 45 iv 3.3.4.2 Approach Overview . 47 3.3.5 Algorithmic Implementations . 48 3.3.5.1 Sense Label Propagation Algorithm . 48 3.3.6 Clustering Document Evidences . 57 3.3.7 The ‘complexity of extraction’ score . 60 3.3.8 Experimental Evaluations . 61 3.3.8.1 Efficacy of the Algorithms . 62 3.3.8.2 NER Improvements . 64 3.3.9 Related Work . 71 3.3.9.1 Characterizing Extraction Difficulty . 71 3.3.9.2 Estimating Extraction Difficulty . 73 3.3.9.3 Cultural Entity Identification and WSD . 75 3.3.10 Discussion . 76 3.4 Cultural NER – Multiple Senses in the Same Domain . 79 3.4.1 Use of Domain Knowledge for Cultural NER . 81 3.4.1.1 Our Approach and Contributions . 83 3.4.2 Related Work . 84 3.4.3 Restricted Entity Extraction . 86 3.4.3.1 Ground Truth Data Set . 86 3.4.3.2 Impact of Domain Restrictions . 88 3.4.4 Real World Constraints . 89 3.4.5 NLP Assist . 93 3.4.5.1 Feature Space for NER . 94 3.4.6 Data and Experiments . 97 3.4.6.1 Usefulness of Feature Combinations . 98 3.4.7 Improving Spotter Accuracy Using NLP Analysis . 100 3.4.8 Discussion . 101 3.5 Summary of NER Contributions . 103 3.5.1 Applications of NER in Social Media Content . 103 4 4. Summarizing User-generated Content 104 4.1 Key Phrase Extraction - ‘Aboutness’ of Content . 104 4.1.1 Thesis Focus - Summarizing Social Perceptions . 106 4.1.2 Key Phrase Extraction - Approach Overview . 110 4.1.3 Key Phrase Extraction - Selection . 111 4.1.4 Key Phrase Extraction - Elimination of Off-topic Phrases . 120 4.1.5 Experiments and Evaluation . 127 4.1.5.1 Evaluating Extracted Key Phrases for Browsing Real-time Data on the Web . 127 4.1.5.2 Evaluating Extracted Key Phrases for Targeted Content Delivery 129 4.1.6 Related Work, Applications of Key Phrase Extraction . 132 v 5 5. Applications of Understanding User-generated Content 135 5.1 Mining Online Music Popularity . 137 5.1.1 Vision and Motivation . 137 5.1.2 Top N Lists . 138 5.1.3 Proxies for Popularity . 139 5.1.4 Thesis Contributions . 140 5.1.5 Corpus Details . 141 5.1.6 System Design . 144 5.1.7 Crawling and Ingesting User Comments . 145 5.1.8 Annotation Components . 147 5.1.8.1 Music related / Artist-Track Annotator . 149 5.1.8.2 Sentiment Annotator . 150 5.1.8.3 Spam Annotator . 155 5.1.9 Generation of the Hypercube . 158 5.1.9.1 Projecting to a list . 159 5.1.10 Experiments - Testing and Validation . 160 5.1.10.1 Generating our Top-N list . 160 5.1.10.2 The Word on the Street . 162 5.1.11 Results . 164 5.1.12 Lessons Learned, Broader Impact . 166 5.2 Social Signals from Experiential Data on Twitter . 169 5.2.1 Thesis Contributions - Twitris . 170 5.2.2 Twitris System Overview . 173 5.2.2.1 Gathering Topically Relevant Data . 174 5.2.2.2 Processing Citizen Observations . 177 5.2.2.3 User Interface and Visualization . 178 5.2.3 Broader Impact . 180 6 6. Conclusions and Future Directions 182 6.1 Future Directions . 183 6.1.1 Computational Social Science . 184 6.1.2 Poster, Content and Network Interactions and a Social System . 185 Bibliography 187 vi List of Figures 1.1 Examples of user-generated content from different social media platforms . 4 2.1 Formality scores of text from various genre . 18 2.2 Snapshot of MusicBrainz, a knowledge base of facts in the music domain and examples of in-line annotations of artist names in user-generated content. 21 2.2 Thesis Contributions - Aboutness understanding tasks and use of varied types of contextual cues . 24 3.1 Showing excerpt of a blog discussing two senses of the entity ‘Transformers’ . 41 3.2 Showing steps in the 2-step framework for obtaining the Extraction Complexity of entity e in distribution D. 47 3.3 Constructing the Spreading Activation Network . 52 3.4 Extracted Language Model for the entity ‘The Dark Knight’ . 56 3.5 Entities, their known senses from Wikipedia, and their computed extraction com- plexities . 64 3.7 Features used in judging NER improvements . ..
Details
-
File Typepdf
-
Upload Time-
-
Content LanguagesEnglish
-
Upload UserAnonymous/Not logged-in
-
File Pages210 Page
-
File Size-