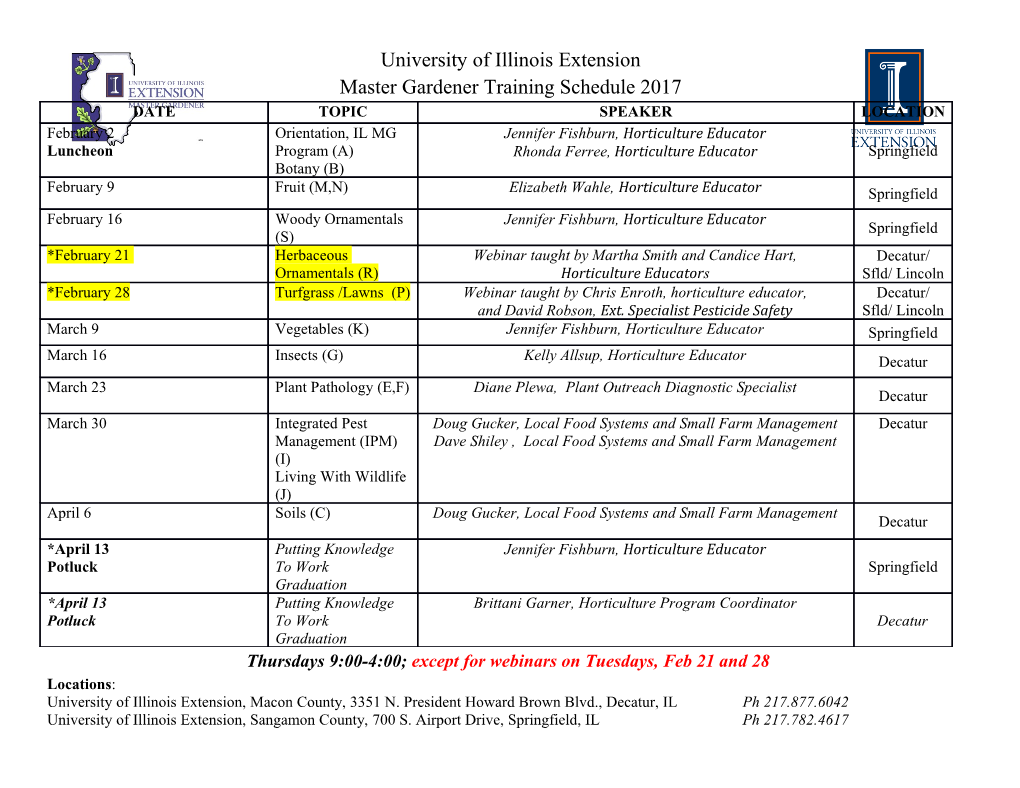
INTEGRATION OF ECONOMIC ACTIVITY BETWEEN THE EURO AREA AND CROATIA Tanja Broz The institute of Economics Zagreb Department for macroeconomic analysis and policy Trg J. F. Kennedya 7 10000 Zagreb Croatia [email protected] Abstract This paper analyses the integration of Croatian and the euro area’s industrial production indices in order to see whether Croatia and the euro area form an optimum currency area. More precisely, it is of interest to determine would future Croatian adoption of euro be of benefit to Croatia (of course, once Croatia joins the EU). First, unit root tests are employed, then cointegration analysis and finally Granger causality test. Results indicate that there is no long run relationship between Croatian and the euro area’s industrial production indices. However, Granger causality test shows that in the short run the euro area’s industrial production causes Croatian. But, this is not enough to conclude that Croatia and the euro area form the optimum currency area. Key words : optimum currency areas, integration of economic activity, Croatia JEL classification : E42, F15, F33 157 1. Introduction In a situation when Croatia started with the negotiation process for joining the EU and when it is very likely that Croatia will join the EU in the next decade at latest, it is justifiable to expect that Croatia will introduce euro, since new members do not have the possibility of the “opt out” clause. For that reason it is necessary to examine whether the introduction of euro will be beneficial for Croatia or will it impose unwanted costs. Discussion about common currency areas usually rests on the theory of optimum currency areas, which was created by Mundell (1961) and supplemented by many other authors such as McKinnon (1963), Kenen (1969), Tower and Willett (1976), Tavlas (1993), Bayumi and Eichengreen (1996) and Frankel and Rose (1998). An optimum currency area is an area for which the costs of abandoning the exchange rate are outweighed by the benefits of adopting a single currency. In addition, the OCA theory can be viewed as a tool for finding an answer to the question on how to choose the optimum exchange rate regime. It should be mentioned, however, that there is no widely accepted algorithm or index to indicate unambiguously should a country join a currency area or not. In fact, there is no standard theory of optimum currency areas, but rather several approaches that have been inspired by Mundell’s (1961) seminal paper. The main goal of this paper is to determine would the common monetary policy with the euro area suit to Croatia. More precisely, it will be determined whether Croatia and the euro area are integrated using industrial production indices. The results should help the economic policy makes with the decisions about changing the exchange rate regime. The rest of the paper is organised as follows. In Chapter 2 some descriptive properties of the data are explored. In Chapter 3 stationarity of time series involved in the analysis is examined. Chapter 4 determines whether those series are cointegrated. If there is a cointegrating relationship between the series, which means that variables have a long run relationship, then the error correction term is used in Granger causality test, which is analysed in Chapter 5. If there is not cointegrating relationship between variables, then Granger causality test is conducted without error correction term. Granger causality test indicates short term adjustment of the selected variables. Last Chapter concludes. 158 2. Data The degree of economic integration is assessed by the similarities of monthly indices for industrial production for the euro area and Croatia. Series go from January 1994 until May 2005. Even though there are available data for the earlier period, it is not desirable to use those data for Croatia, because of recession period associated with the change of the economic system. Stabilisation program was introduced in the beginning of October 1993, so results conducted with sample from 1994 should be more reliable. Industrial production indices represent total industry defined by NACE. Sources for the data on industrial production indices are Central Bureau of Statistics for Croatian data and Eurostat for the euro area’s data. Both time series are seasonally adjusted. Even though industrial production does not account for the total economic activity, there are some advantages in using industrial production instead of time series data for GDP. Since Croatia exists since the beginning of the ‘90s (and GDP started being calculated officially only in 1997), there is not enough data for conducting econometric analysis using quarterly GDP data. Industrial production is reported monthly, which gives more data and more degrees of freedom in conducting the analysis. Also, in transition economies industrial production is probably more accurately measured than many other indicators of economic activity (Korhonen, 2001). Despite the fact that industrial production indices do not reflect total economic activity dynamics 1, until now it was constantly confirmed that the industrial production cycles concur with GDP cycles in Croatia and euro area. This can be seen from Figures 1 and 2, which show quarterly seasonally adjusted industrial production indices and quarterly seasonally adjusted GDP indices from the first quarter 1997 until the second quarter 2004. So, industrial production is used as a proxy for real economic activity in the euro area and Croatia. 1 In the last couple of years, industry sector participate in total gross added value of Croatian economy with about 28 percent ( Čeni ć, 2005) and in euro area with about 21 percent (Eurostat). 159 Figure 1 Quarterly seasonally adjusted industrial p Croatia, 2000 = 100. 130,00 120,00 110,00 100,00 90,00 80,00 70,00 60,00 roduction and GDP indices for Source: Croatian1tr9 Central4 Bureau of Statistics 4tr94 Figure 2 Quarterly seasonally adjusted industrial p 3tr95 euro area, 2000 = 100. 2tr96 1tr97 110,00 105,00 4tr97 100,00 3tr98 95,00 2tr99 Industrial production 90,00 1tr00 GDP 85,00 4tr00 80,00 3tr01 75,00 2tr02 70,00 1tr03 roduction and GDP indices for4t rthe03 Source: Eurostat1t r94 3tr04 4tr94 3tr95 2tr96 1tr97 4tr97 3tr98 2tr99 1tr00 Industrial production 4tr00 GDP 3tr01 2tr02 1tr03 160 4tr03 3tr04 3. Unit root tests Not until a long ago economists implicitly presumed that macroeconomic data were stationary, which for expositional purposes can be thought of as “nicely behaved”, or at least stationary around a deterministic time trend (Kennedy, 1996). But, as econometric research studies showed, most macroeconomic data are, in fact, nonstationary. This means that statistics such as t and Durbin-Watson statistics and measures such as R 2 did not retain their traditional characteristics. 2 If a researcher would run a regression with such a data, she or he would produce spurious results (Kennedy, 1996). 3 So, in order to have valid estimates, it has become very important before starting with the analysis to test for nonstationarity in time series data. 4 Even though industrial production samples have monthly data, this does not help to increase the power of the unit root test, because in unit root tests it is not the frequency of the data that counts but longer time period. Hence, in order to increase the power of the tests, we used several unit root tests. Also, after we made Augmented Dickey-Fuller test (ADF), Phillips-Peron test (PP) and Dickey-Fuller-Generalised Least Squares test (DF-GSL), we compared the results with other studies which tested industrial production indices for unit roots. Unit root test results for Croatian industrial production index we compared with Čeni ć (2005) and unit root test results for euro area’s industrial production index we compared with Korhonen (2001). Even though time span differs, the main results are the same. Figure 3 shows industrial production indices for Croatia and the euro area in levels. Brief look to the figure indicates that those series are increasing with time, which could mean that they are nonstationary. If they are integrated in order one, this should mean that their first differences should be stationary. 2 If two stationary variables are generated as independent random series, when one of those variables is regressed on the other, the t-ratio on the slope coefficient would be expected not to be significantly different form zero and R2 would be expected to be very low (Brooks, 2002). 3 If two unrelated series contain a trend and are thus nonstationary, as the sample grows in size, the trend will dominate, causing the R 2 between two series to come near unity. This happens because the total sum of squares becomes infinite, causing R 2 (which is calculated with formula 1 – explained sum of squares/total sum of squares) to come close to one. t statistics also blows up, because of high R 2. So, this reflects the problem of spurious regression results – unrelated integrated (nonstatinary) series appear to be related, if one uses conventional methods in analysis. 4 One of the first who pointed out this matter were Granger and Newbold (1974). 161 Figure 3 Indices of industrial production for euro 130 120 110 100 90 80 70 area and Croatia – levels Source: Croatian Central Bureau of Statistics and E 60 Series are seasonally adjusted. 1994m01 1994m06 1994m11 1995m04 indices’ order of integration, which is important1995m09 f 1996m02 Unit root tests are in this paper used in order to than we can proceed with tests for cointegration. 1996m07 1996m12 1997m05 1997m10 1998m03 results in first differences. The methodology is as 1998m08 Table 1 shows results for ADF, DF-GLS and PP tests 1999m01 values (this means that 1999m06 hypothesis is rejected at conventional test levels. 1999m11 2000m04 than critical values, the unit root hypothesis cann 2000m09 urostat 2001m02 Tables report 2001m07 is being rejected.
Details
-
File Typepdf
-
Upload Time-
-
Content LanguagesEnglish
-
Upload UserAnonymous/Not logged-in
-
File Pages21 Page
-
File Size-