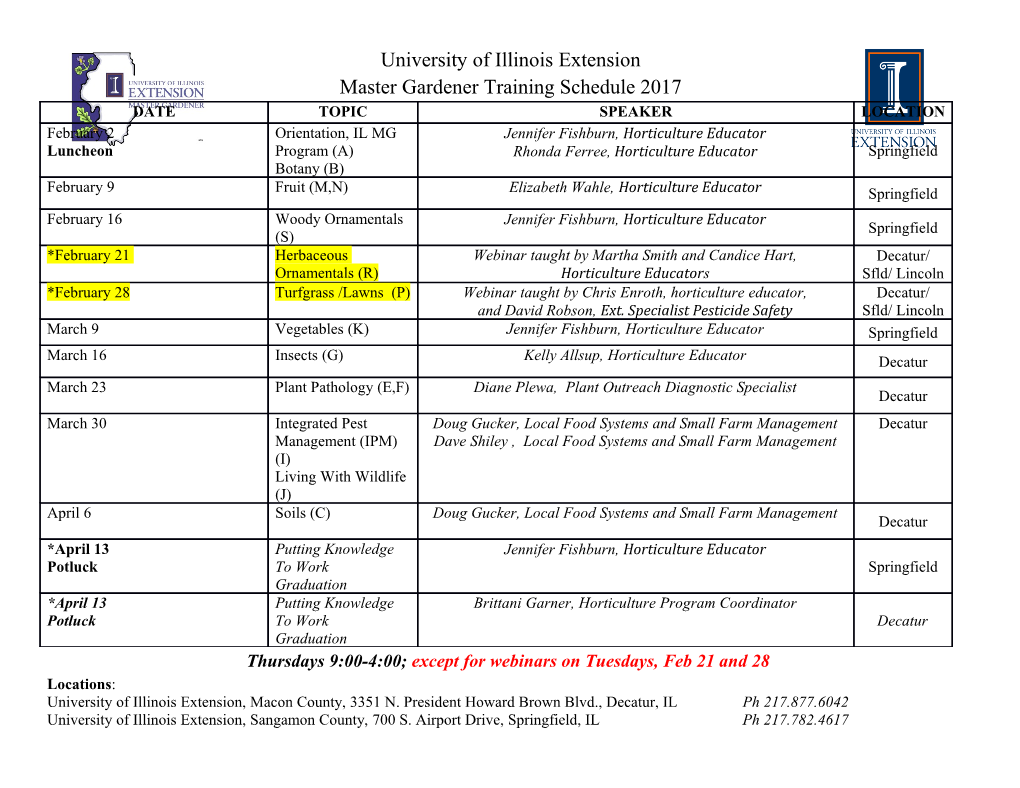
STUDY Requested by the FEMM committee Education and employment of women in science, technology and the digital economy, including AI and its influence on gender equality Policy Department for Citizens’ Rights and Constitutional Affairs Directorate-General for Internal Policies PE 651.042- April 2020 EN Education and employment of women in science, technology and the digital economy, including AI and its influence on gender equality Abstract This study, commissioned by the European Parliament’s Policy Department for Citizens’ Rights and Constitutional Affairs at the request of the FEMM Committee, provides evidence that there is still gender bias and inequality in STEM (Science, Technology, Engineering, Mathematics) fields and the digital sector (e.g., digital technologies, Computer Science, Information Technology, Information and Communication Technology, Artificial Intelligence, cybersecurity). This document, prepared at the request of the FEMM Committee (Policy Department for Citizens’ Rights and Constitutional Affairs, Directorate-General for Internal Policies), is intended to provide an up-to-date literature review on the current status of women’s education and employment in STEM fields and the digital sector. In so doing, the corresponding trajectories are examined, from the primary education level up to the employment level, in an attempt to identify obstacles and bottlenecks that prevent gender parity. Finally, suggestions for future research, initiatives and policies that would improve women’s participation in these areas are made. This document was requested by the European Parliament's Committee on Citizens' Rights and Constitutional Affairs. AUTHORS Prof. Dr. Zacharias C. Zacharia, Research in Science and Technology Education Group, Department of Educational Sciences, University of Cyprus Dr. Tasos Hovardas, Research in Science and Technology Education Group, University of Cyprus Dr. Nikoletta Xenofontos, Research in Science and Technology Education Group, University of Cyprus Ms Ivoni Pavlou, Research in Science and Technology Education Group, University of Cyprus Ms Maria Irakleous, Research in Science and Technology Education Group, University of Cyprus ADMINISTRATOR RESPONSIBLE Martina SCHONARD EDITORIAL ASSISTANT Sandrina MARCUZZO LINGUISTIC VERSIONS EN ABOUT THE EDITOR Policy departments provide in-house and external expertise to support EP committees and other parliamentary bodies in shaping legislation and exercising democratic scrutiny over EU internal policies. To contact the Policy Department or to subscribe for updates, please write to: Policy Department for Citizens’ Rights and Constitutional Affairs European Parliament B-1047 Brussels Email: [email protected] Manuscript completed in April 2020 © European Union, 2020 This document is available on the internet at: http://www.europarl.europa.eu/supporting-analyses DISCLAIMER AND COPYRIGHT The opinions expressed in this document are the sole responsibility of the authors and do not necessarily represent the official position of the European Parliament. Reproduction and translation for non-commercial purposes are authorised, provided the source is acknowledged and the European Parliament is given prior notice and sent a copy. © Cover image used under licence from Shutterstock.com Education and employment of women in science, technology and the digital economy, including AI and its influence on gender equality CONTENTS LIST OF ABBREVIATIONS 6 LIST OF TABLES 7 EXECUTIVE SUMMARY 8 GENERAL INFORMATION 11 1.1. Introduction 11 1.2. Methods 11 SUMMARY OF KEY FINDINGS 13 PRIMARY EDUCATION 15 3.1. Inconsistency between girls’ attitudes and ability and their career beliefs 15 3.2. Opportunities for initiating and sustaining girls’ interest 15 3.3. Barriers presented by social and academic environments 16 SECONDARY EDUCATION 17 4.1. Achievement and attitudes 17 4.2. Time window for choice of subjects and differential preferences of females and males 18 4.3. Initial interest 19 4.4. Social interaction 20 4.4.1. Student-teacher interaction 20 4.4.2. Student-student interaction 20 4.4.3. Student-parent interaction 22 4.4.4. Adverse effects of support provided by teachers and parents 22 4.5. Socio-cultural aspects 22 4.6. Policy reforms focusing on secondary education 23 HIGHER EDUCATION 25 5.1. Choice of subjects 25 5.1.1. Female percentages lag behind male figures across all levels of tertiary education 25 5.1.2. The special case of Computer Science 25 5.1.3. Female preference for doctoral studies 26 5.2. Gender stereotypes and gender bias 26 5.2.1. Females tend to undervalue their own capacities 26 5.2.2. Responsibilisation and contradictory incentive structures for female academics and researchers 28 5.2.3. The gender productivity gap 28 5.3. Social interaction 29 PE 651.042 3 IPOL | Policy Department for Citizens’ Rights and Constitutional Affairs 5.3.1. The dominance and implications of cisgender male heterosexuality in higher education institutes 29 5.3.2. Student-instructor interaction 30 5.3.3. Student-student interaction 31 5.4. Gender and grants in academia 32 EMPLOYMENT 34 6.1. Figures and trends for female employment 34 6.1.1. The European context 34 6.1.2. The special case of Computer Science 34 6.2. Women face disproportionally more obstacles in their careers than men 34 6.3. Women in upper-level positions and gender salary gaps 35 6.4. How far gender discrimination can penetrate: Unintended gender differences in the delivery of social media ads for careers 36 6.5. Differential gender responses to gender bias in employment 37 6.6. Gender diversity propels performance 37 6.7. Institutions established to close the gender gap 38 EXAMPLES OF GENDER BIAS AND INEQUALITIES FROM THE DIGITAL SECTOR: THE CASES OF ARTIFICIAL INTELLIGENCE AND CYBERSECURITY 42 7.1. The case of Artificial Intelligence 42 7.1.1. Gender representation in AI 43 7.1.2. How does gender bias appear in AI and what causes this bias 44 AI gender is stereotyped 44 Underlying gender bias in AI 44 Gendered issues in the AI workplace 45 7.1.3. Overcoming gender bias in AI 45 7.2. The case of cybersecurity 46 7.2.1. Why fewer females select cybersecurity for employment 47 Social/institutional barriers 47 Personal barriers 48 7.2.2. Overcoming the barriers to attracting more females to cybersecurity 48 MAIN DISCUSSION POINTS 51 8.1. Biological, individual, and socio-cultural determinants of the gender gap 51 8.2. No magic wand to fix the gender gap 52 8.2.1. Interventions targeting individual participants may backfire 52 8.2.2. Interventions need to be delivered within real-world contexts 52 8.2.3. Different STEM fields may need to be treated differently 53 4 PE 651.042 Education and employment of women in science, technology and the digital economy, including AI and its influence on gender equality 8.3. Problematize the “leaky pipeline” metaphor 53 8.4. Create enabling environments in education and workplace 54 8.5. Problematize the role of schools 54 8.6. Multi-level approach needed to address the gender gap 55 POLICY RECOMMENDATIONS 56 9.1. Stakeholder interaction at the EU level (macro-level) 56 9.2. Select and analyse cohort data anchored in real-world contexts (macro-level) 56 9.3. Create and sustain enabling education and work environments (meso-level) 57 9.4. Concentrate on positive feedback loops and bottlenecks (meso-level) 57 9.5. Problematize reference material and pedagogical approaches (micro-level) 59 9.6. Problematize peer interactions (micro-level) 60 9.7. Participatory scenario development and assessment for operationalizing stakeholder joint action in combating gender inequality in STEM, ICT, and CS (including AI and cybersecurity) 60 REFERENCES 63 REFERENCE LIST 1 – REFERENCES USED IN THE REVIEW 63 REFERENCE LIST 2 – REFERENCES USED IN THE REVIEW FOR THE CHAPTER TITLED: “EXAMPLES OF GENDER BIAS AND INEQUALITIES FROM THE DIGITAL SECTOR: THE CASES OF ARTIFICIAL INTELLIGENCE AND CYBERSECURITY” 75 REFERENCE LIST 3 – ADDITIONAL REFERENCES USED IN THE CHAPTERS “MAIN DISCUSSION POINTS” AND “POLICY RECOMMENDATIONS” 79 ANNEX 80 PE 651.042 5 IPOL | Policy Department for Citizens’ Rights and Constitutional Affairs LIST OF ABBREVIATIONS AI Artificial Intelligence CS Computer Science ERAC European Research Area and Innovation Committee GDP Gross Domestic Product ICT Information and Communication Technology IT Information Technology SWG Standing Working Group STEM Science, Technology, Engineering, and Mathematics SWOT Strengths, Weaknesses, Opportunities, And Threats 6 PE 651.042 Education and employment of women in science, technology and the digital economy, including AI and its influence on gender equality LIST OF TABLES Table 1: Institutions/initiatives aiming to address the gender gap in STEM in the USA 39 Table 2: Institutions/initiatives aiming to address the gender gap in STEM in Europe 40 Table 3: Positive feedback loops/bottleneck effects for female interest or representation 58 Table 4: Recommendations for future research on gender inequality and discrimination in STEM, ICT, and CS 58 Table 5: Draft scenarios developed for joint stakeholder action to promote gender equality in STEM, ICT, and CS: Focus schools in secondary education 62 PE 651.042 7 IPOL | Policy Department for Citizens’ Rights and Constitutional Affairs EXECUTIVE SUMMARY Gender parity is vital to the prosperity of the EU, because it has been found to affect GDP, levels of employment, and productivity. STEM fields and the digital sector (e.g., digital technologies, CS, IT, ICT, AI, cybersecurity) are among the employment domains where gender bias prevails. Hence, addressing these inequalities is of high importance, especially when considered within the frame of the EU’s principles and values. This document has been prepared at the request of the FEMM Committee (Policy Department for Citizens’ Rights and Constitutional Affairs, Directorate-General for Internal Policies). Major areas of concern for the Committee include the evidence of persisting biases and inequalities in STEM fields and the digital sector, in spite of the fact that these biases and inequalities were identified several decades ago and efforts have been made to address them over the years.
Details
-
File Typepdf
-
Upload Time-
-
Content LanguagesEnglish
-
Upload UserAnonymous/Not logged-in
-
File Pages86 Page
-
File Size-