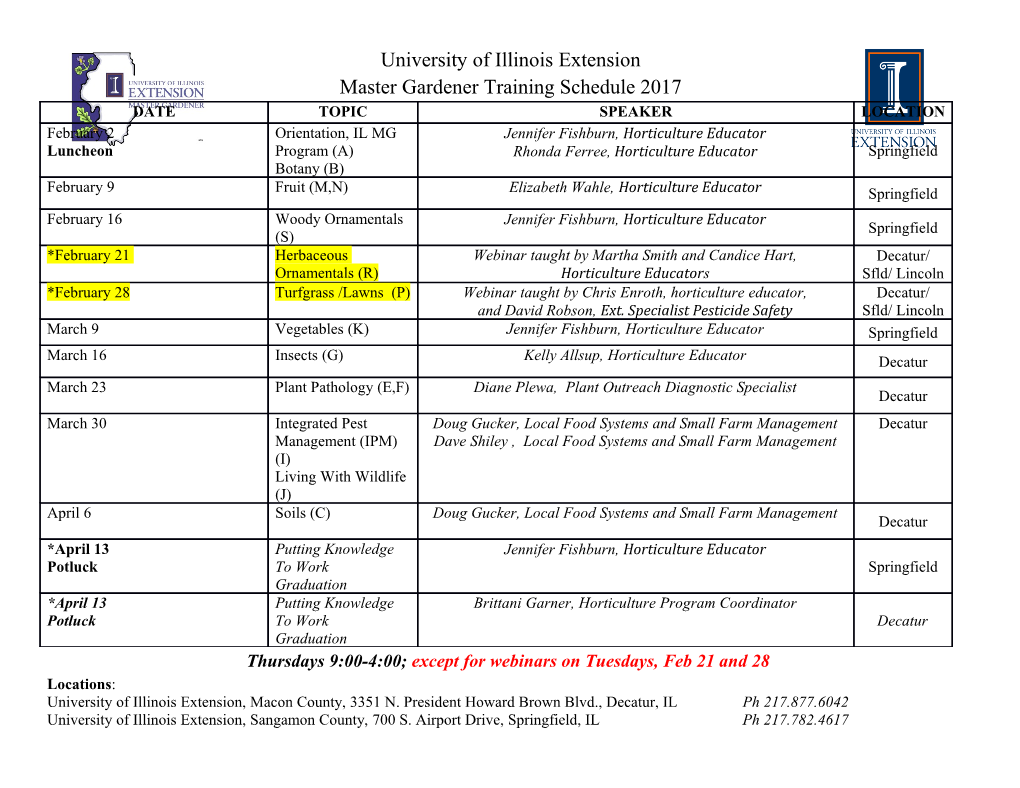
Chemical Prioritization Methods for Nuclear Receptor Modulators at the U.S. EPA Keith A. Houck, Ph.D. National Center for Computational Toxicology (NCCT/ORD/EPA) EDTA International Symposium, Dongguk University November 9, 2018 [email protected] The views expressed in this presentation are those of the author and do not necessarily reflect the views or policies of the U.S. EPA Use of commercial names does not constitute endorsement of those brands U.S. Environmental Protection Agency Regulatory Agencies Make a Broad Range of Decisions on Chemicals… Number of Chemicals Lack of Data /Combinations 70 60 • Number of chemicals and 50 40 combinations of chemicals is 30 extremely large (>20,000 substances 20 <1% Percent of Chemicals of Percent 10 on active TSCA inventory) 0 Acute Cancer • Due to historical regulatory Gentox Dev Tox requirements, most chemicals lack Repro Tox EDSP Tier 1 Modified from Judson et al., EHP 2010 traditional toxicity testing data Ethics/Relevance Economics • Traditional toxicology testing is Concerns $10,000,000 expensive and time consuming $1,000,000 • Traditional animal-based testing has $100,000 Cost issues related to ethics and relevance $10,000 $1,000 Toxicology Moving to Embrace 21st Century Methods 3 High-Throughput Assays Used to Screen Chemicals for Potential Toxicity Hundreds High‐ Thousands Throughput of Chemicals ToxCast/Tox21 Assays • Understanding of what cellular processes/pathways may be perturbed by a chemical 4 • Understanding of what amount of a chemical causes these perturbations Broad Success Derived from High- Throughput Screening Approaches Group Chemicals by Provide Mechanistic Prioritization of Chemicals Similar Bioactivity and Support for Hazard ID for Further Testing Predictive Modeling Chemicals FIFRA SAP, Dec 2014 IARC Monographs Assays/Pathways 5 Focus on Nuclear Receptors and Xenobiotics • Family of ligand-regulated nuclear transcription factors (48 human) • Conserved, modular domains – DNA-binding domain – Ligand-binding domain • Binds lipophilic, small molecules • Endogenous ligands: steroid hormones, fatty acids • Regulates genes for key physiological processes: endocrine system, growth and differentiation, metabolism • Endogenous ligand physicochemical properties consistent with cell permeable qualities • Good focus for selective xenobiotic effects http://proteopedia.org/wiki/index.php/Image:3dzy2.png Ligands for Nuclear Hormone Receptors From the EPA’s Endocrine Disruptor Screening Management Plan: “Examine effects of these chemicals on estrogen, androgen and thyroid hormone-related processes” Sex Steroids ER Vitamin D VDR Estrogens, Progestins, Androgens Unknowns PR OH O OH OH AR O HO O HO OH Glucocorticoids GR Lipids PPARs OH O O HO OH OH O O Mineralocorticoids MR Oxysterols LXRs OH HO O O O O HO Thyroid Hormones TR Bile Acids O FXR I I OH NH O 2 H HO O I OH HO OH H Retinoids RARs Xenobiotics PXR O RXRs Cl CAR OH N N 7 The Estrogen Receptor Model • Public solicitation for diverse high-throughput assays to cover broad range of bioactivity/toxicity endpoints • Many estrogen receptor assays included – Binding – Nuclear localization – Transactivation – Cell proliferation • No single assay perfect for a variety of reasons • Decided to develop computational model utilizing all data Targeted Pathways 18 In Vitro Assays Measure ER-Related Activity R3 A1 Receptor (Direct Molecular Interaction) A2 Intermediate Process A3 Assay ER Receptor ER Receptor Binding 3 Binding R2 R1 Noise Process (Antagonist) A4 (Agonist) R4 ER agonist pathway A5 ER antagonist pathway A6 Dimerization N7 N1 Dimerization Interference pathway A7 Cofactor A8 Cofactor N8 N2 Recruitment Recruitment A9 R5 DNA DNA N9 N3 Binding A10 Binding R6 A12 R9 A11 RNA N4 Transcription A13 A17 Antagonist R7 N10 Transcription Protein A14 A18 Suppression Production N5 A15 ER‐induced R8 N6 Judson et al., Tox Sci. 2015 Proliferation A16 Browne et al., ES&T. 2015 Kleinstreuer et al., EHP 2016 ER Model Performance In vivo Comparison Rank Order (ER Agonist AUC) Judson et al., Tox Sci 2015 Browne et al., Environ. Sci. Technol., 2015 ER Minimal Model Combinations of four assays provide good balanced accuracy R.S. Judson et al. / Regulatory Toxicology and Pharmacology 91 (2017) 39e49 Regulatory Applications: EDSP “The approach incorporates validated high-throughput assays and a computational model and, based on current research, can serve as an alternative for some of the current assays in the Endocrine Disruptor Screening Program (EDSP) Tier 1 battery.” Androgen Receptor Screening • Utilize existing ToxCast/Tox21 assays to develop AR model • Cytotoxic chemicals confounded antagonist cell-based assays • Run additional confirmation assay for antagonists – Higher agonist concentration – Competitive antagonists show right-shift in potency ToxCast/Tox21 Assays Antagonist Mode Evaluation of AR Model Summary Reference Data • Model has high sensitivity Literature Review • Antagonist mode specificity improved by considering Reference Chemical antagonist assay with high Classifications agonist concentration • Weakly active chemicals most Model Performance difficult to detect Evaluation • Broad screening suggested cytotoxic compounds not all excluded Validated Model for Chemical Screening Chem Res Toxicol. 30:946-964, 2017. Agonists versus Antagonist Selective receptor modulators behave conditionally as agonists and/or antagonists 17-Estradiol 4-Hydroxytamoxifen Fulvestrant Brzozowski et al., Nature. 389: 753–8, 1997). Tox21 AR Screening Results ⁓ 8,000 chemicals Hydroxyflutamide Bis(tributyltin)oxide • Only 102 chemicals positive Dipyrithione using strictest criteria Ziram NTP Mix21 AR2 2‐EQP • Expanding criteria allows for ranking of chemicals based on 17alpha‐Ethinylestradiol strength of evidence Bis(1‐piperidinylthioxomethyl)hexasulfide Triphenyltin acetate • Chemicals that are confounded Tributyltin benzoate by cytotoxicity are not eliminated Nilutamide but evidence is weaker Triethyltin bromide • Potency not currently considered Equilin but is another important factor 17alpha‐Estradiol (Acryloyloxy)(tributyl)stannane Triphenyltin fluoride Ethylestrenol Copper dimethyldithiocarbamate Vinclozolin Challenges with assessing NR antagonism in vitro • Measuring loss of signal- confounded by cytotoxicity • To address: – Two different assay platforms – Use bootstrapping techniques to determine effect of cytotoxicity – Two concentrations of agonist R1881 – MARCoNI assay for corepressor/activator recruitment Antagonist Reference Chemical Results LUC vs LUC vs Chemical Designation Assay Hitcalls LUC_counterscreen LUC_viability Procymidone Very Weak Antagonist BLA, LUC, LUCcs Yes Yes Fenarimol Very Weak Antagonist BLA, LUC, LUCcs Yes Yes 4-(1,1,3,3- Tetramethylbutyl)phenol Weak Antagonist LUC Yes Yes o,p'-DDT Weak Antagonist BLA, LUC Yes Yes Antagonist p,p'-DDE Weak Antagonist LUC Yes Yes Propiconazole Weak Antagonist BLA, LUC, LUCcs Yes No Screening Zearalenone Weak Antagonist BLA, LUC, LUCcs No No Methoxychlor Weak Antagonist BLA, LUC, LUC2 No No • LUC: R1881 = 0.5 nM Linuron Moderate/Weak Antagonist BLA, LUC Yes No Vinclozolin Moderate/Weak Antagonist BLA, LUC, LUCcs Yes Yes Flutamide Moderate/Weak Antagonist BLA, LUC, LUCcs Yes Yes Bisphenol A Moderate/Weak Antagonist BLA, LUC, LUCcs Yes Yes • LUC_counterscreen: Prochloraz Moderate/Weak Antagonist BLA, LUC, LUCcs Yes Yes Cyproterone acetate Moderate Antagonist BLA, LUC Yes Yes R1881 = 10 nM Nilutamide Moderate Antagonist BLA, LUC, LUCcs Yes Yes Spironolactone Strong/Moderate Antagonist BLA, LUC No Yes Mifepristone Strong/Moderate Antagonist BLA, LUC, LUCcs No Yes Fenitrothion Strong Antagonist BLA, LUC, LUCcs Yes Yes Hydroxyflutamide Strong Antagonist BLA, LUC, LUCcs Yes Yes Bicalutamide Strong Antagonist BLA, LUC, LUCcs Yes Yes 17-Methyltestosterone Negative Antagonist NA NA NA 4-Androstene-3,17-dione Negative Antagonist NA NA No Atrazine Negative Antagonist NA NA NA Daidzein Negative Antagonist BLA NA NA Deltamethrin Negative Antagonist NA NA NA Methomyl Negative Antagonist LUCcs NA No Simazine Negative Antagonist NA NA NA MARCoNI assay Microarray Assay for Real-time Coregulator-Nuclear receptor Interaction • Cell-free assay measuring co-regulator recruitment to AR-LBD – 154 co-regulators – 3 concentrations (1, 10, 100 uM) – log fold-change of binding compared to DMSO • Tested 318 suspected AR antagonists • Reduced variables (co-regulators) to 28 most affected • Goal: to see if patterns of coregulatory recruitment can distinguish between true antagonists and false antagonists (cytotoxicity/artifacts) Image: pamgene.com High confidence: Cluster 1-2 Lower confidence: Cluster 3 2 1 34 No confidence: Clusters 4-7 5 67 7 Cyproterone 6 5 Bicalutamide DHT 4 Hydroxyflutamide Unique 3 chemicals 2 1 Rank Spearman Dissimilarity/Wards Co-regulator Recruitment Patterns • Mean value of cluster 1 2 3 4 5 6 7 plotted per coregulator • Loss of binding seen 1 in cluster 2&3 versus 1 (red oval) 2 • These represent SRC 3 coactivators that have 4 6 histone acetyl 7 5 transferase activity • Selective receptor modulators; likely would influence biological response Thyroid Hormone Receptor Modulators: Tox21 qHTS Campaign Primary Screen Hit Characterization ASSAYS ASSAYS Rat pituitary GH3 cell line* GH3 expressing endogenous TRα GAL4-TR (human) and TRβ, with TRE regulating GAL4-RXR (human) luciferase expression TR/TR coactivator recruitment Cell viability TR nuclear translocation * Developed by Albertinka Murk, Wageningen University, the Netherlands TR Modulator Hit Characterization: TR Coactivator Assay (Invitrogen):
Details
-
File Typepdf
-
Upload Time-
-
Content LanguagesEnglish
-
Upload UserAnonymous/Not logged-in
-
File Pages43 Page
-
File Size-