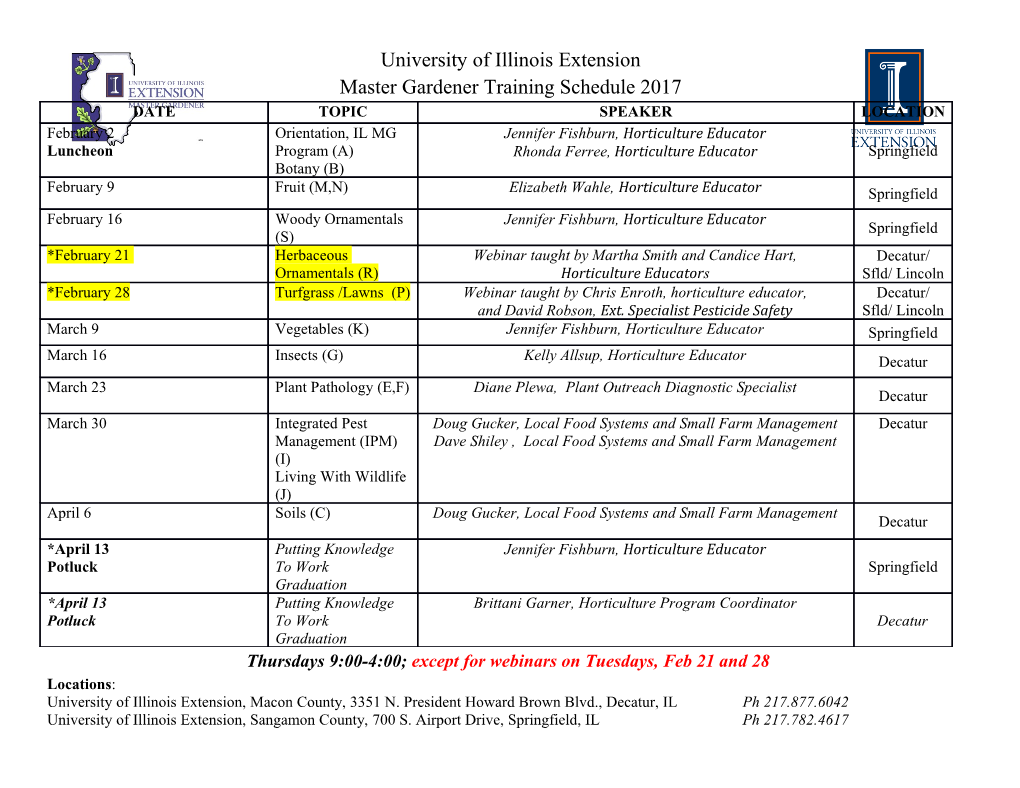
remote sensing Article Eleven Years of Mangrove–Mudflat Dynamics on the Mud Volcano-Induced Prograding Delta in East Java, Indonesia: Integrating UAV and Satellite Imagery Sebrian Mirdeklis Beselly 1,2,3,* , Mick van der Wegen 1,4, Uwe Grueters 5, Johan Reyns 1,4 , Jasper Dijkstra 4 and Dano Roelvink 1,2,4 1 Department of Coastal & Urban Risk & Resilience, Coastal Systems & Engineering and Port Development, IHE Delft Institute for Water Education, Westvest 7, 2611 AX Delft, The Netherlands; [email protected] (M.v.d.W.); [email protected] (J.R.); [email protected] (D.R.) 2 Department of Hydraulic Engineering, Section Coastal Engineering, Delft University of Technology, Stevinweg 1, 2628 CN Delft, The Netherlands 3 Department of Water Resources Engineering, Brawijaya University, Malang 65145, Indonesia 4 Deltares, 2629 HV Delft, The Netherlands; [email protected] 5 Institute of Plant Ecology, Justus Liebig University, 35392 Giessen, Germany; [email protected] * Correspondence: [email protected]; Tel.: +31-152-151-160 Abstract: This article presents a novel approach to explore mangrove dynamics on a prograding delta by integrating unmanned aerial vehicle (UAV) and satellite imagery. The Porong Delta in Indonesia Citation: Beselly, S.M.; van der has a unique geographical setting with rapid delta development and expansion of the mangrove belt. Wegen, M.; Grueters, U.; Reyns, J.; This is due to an unprecedented mud load from the LUSI mud volcanic eruption. The mangrove Dijkstra, J.; Roelvink, D. Eleven Years dynamics analysis combines UAV-based Structure from Motion (SfM) photogrammetry and 11 years of Mangrove–Mudflat Dynamics on (2009–2019) satellite imagery cloud computing analysis by Google Earth Engine (GEE). Our analysis the Mud Volcano-Induced Prograding shows unique, high-spatiotemporal-resolution mangrove extent maps. The SfM photogrammetry Delta in East Java, Indonesia: Integrating UAV and Satellite analysis leads to a 3D representation of the mangrove canopy and an estimate of mangrove biophysi- Imagery. Remote Sens. 2021, 13, 1084. cal properties with accurate height and individual position of the mangroves stand. GEE derived https://doi.org/10.3390/rs13061084 vegetation indices resulted in high (three-monthly) resolution mangrove coverage dynamics over 11 years (2009–2019), yielding a value of more than 98% for the overall, producer and consumer Academic Editors: accuracy. Combining the satellite-derived age maps and the UAV-derived spatial tree structure Farid Dahdouh-Guebas and allowed us to monitor the mangrove dynamics on a rapidly prograding delta along with its structural Viviana Otero attributes. This analysis is of essential value to ecologists, coastal managers, and policymakers. Received: 27 January 2021 Keywords: mangroves; remote sensing; Google Earth Engine; SfM photogrammetry; UAV Accepted: 10 March 2021 Published: 12 March 2021 Publisher’s Note: MDPI stays neutral 1. Introduction with regard to jurisdictional claims in published maps and institutional affil- Mangroves are trees or large shrubs that grow in or adjacent to the intertidal zone and iations. are distributed along (sub-)tropical coasts and estuaries [1,2]. Mangroves are well-known for providing a range of ecosystem services such as timber production, carbon sequestration, soil formation, nutrient cycling, habitat creation for marine and terrestrial species, and protecting coastlines by attenuating waves and limiting erosion [2–4]. However, land use conversion has caused mangrove forests to decline at a rate three to five times larger Copyright: © 2021 by the authors. than the average forest loss. Hence, mangroves’ important ecosystem services will be Licensee MDPI, Basel, Switzerland. diminished [2]. This article is an open access article distributed under the terms and In order to gain a better understanding of the value of important ecological services conditions of the Creative Commons provided by mangroves, further efficient collection of data, e.g., mangrove extent, height, Attribution (CC BY) license (https:// individual position, and species membership are necessary. Earth observation offers a creativecommons.org/licenses/by/ method for the large-scale monitoring and assessment of the environment, especially when 4.0/). it comes to the mangrove forest inventory and spatial extent monitoring that are generally Remote Sens. 2021, 13, 1084. https://doi.org/10.3390/rs13061084 https://www.mdpi.com/journal/remotesensing Remote Sens. 2021, 13, 1084 2 of 28 difficult to carry out on the ground [5]. Mapping mangrove forests is one of the most challenging tasks in remote sensing since the forests are mostly quite large, located in a remote area, and persistent cloud cover in the tropical areas [2,6,7]. Several efforts have been made to monitor the spatial extent of mangrove forests from local to the global scales. Two global-scale mangrove extent maps were released for 2000, i.e., the World Atlas of Mangrove (WAM) [1] and the Global Mangrove Forest Distribution v1 (GMFD) [8]. Other current global products are the Continuous Global Mangrove Forest Cover for the 21st Century (GCMFC-21) that showing annual global mangrove forest cover from 2000 to 2012 [9] and Global Mangrove Watch (GMW) v2.0 [10]. Information on important structural attributes of mangroves such as tree height, age, and diameter at breast height (dbh) is required to characterise the mangrove forest stands. Accurate information of tree height is essential, since there is a high correlation of the height and the dbh, basal area (BA), and above ground biomass (AGB) [5,11]. Mangrove forests with their harsh physical conditions hinder surveyors from carrying out direct measurements in the field. In principle, a number of remote sensing approaches have been developed as supplement or substitute for ground-based inventory [12–15]. A Canopy Height Model (CHM)—that is, a three-dimensional representation of the forest—can be derived by using airborne or terrestrial LiDAR, Synthetic Aperture Radar (SAR), and high-resolution optical imagery [16]. Global satellite datasets such as Shuttle Radar Topography Mission (SRTM) and ICESat/ GLAS [16,17], and ICESat-2/ATLAS launched in 2018 [18] have proven to be useful in deriving canopy height, but they were released in a medium resolution of 30 m × 30 m. TanDEM-X InSAR, a DEM product developed by the German Space Agency (2011–2015) with a ground resolution of 12.5 × 12.5 m, has also been used to estimate canopy height [14]. Recently, Very High Resolution (VHR) CHM derived from Unmanned Aerial Vehicle (UAV)- based Structure from Motion (SfM) Photogrammetry has been deployed [15,16] often at a local scale. The SRTM, ICESat, and TanDEM are the most commonly utilised methods to estimate CHM since they have global coverage, and the data are easily accessible [19]. However, the passive/optical sensors solely obtain surface canopy information, and radar has at least limited penetration capability [19]. LiDAR, on the other hand, is able to penetrate the canopy cover to some extent depending on the acquisition design and lidar system [20]. However, this technique can be associated with high cost which limits the use of this system [16,19]. This study takes the Porong Estuary, Indonesia, as a case study. Porong Estuary provided a unique setting as this area is characterised by a rapidly prograding delta and concomitant mangrove expansion [21–23]. It is because of the mud volcanic eruption in 2006 and subsequent mudflow diversion known as LUSI (acronym of lumpur (mud) and sidoarjo (the regency name)) [23]. Several studies have been conducted to investigate the effects of LUSI’s high sediment load on the Porong River, the Porong Estuary, and surrounding coastal waters [21,24,25] and the development of mangrove on the created wetland in general [26–28]. However, none of them explored the mangrove dynamics and investigated the structural characterisation of the mangroves in the rapidly prograding delta. This study aims to analyse mangrove dynamics on a rapidly prograding delta by a novel integration of UAV SfM Photogrammetry and multiple sources of satellite imagery in cloud computing Google Earth Engine (GEE). The first objective of this study is to retrieve mangrove biophysical properties (height and individual location of trees) employing the off-the-shelf UAV SfM photogrammetry, in combination with ground-truthing based on field data. The second objective is to generate a three-monthly classification of mangrove areas using Landsat 7, Landsat 8, Sentinel 1, and Sentinel 2 in GEE. These mangrove extent maps represent the dry season and wet season, where two maps were created during each of these seasons every three months. The third objective is to estimate the mangrove age and age-height relationship based on the combination of UAV and GEE analysis. Remote Sens. 2021, 13, 1084 3 of 28 2. Materials and Methods 2.1. Study Area The Porong Delta, as presented in Figure1 is located in East Java Province, Indonesia (7.569 S, 112.872 E). It is approximately 37 km southeast of Surabaya, the second-largest city in Indonesia. It has a monsoon climate with a dry (April-September) and wet (October- March) season [29], in which the high precipitation contributes up to 80% of the mean annual precipitation [30]. The Porong River is one of the two major branches of the Brantas watershed. The watershed is regulated with several large dams, barrages, and flood gates. During the dry season, the flow is diverted to Surabaya, and therefore
Details
-
File Typepdf
-
Upload Time-
-
Content LanguagesEnglish
-
Upload UserAnonymous/Not logged-in
-
File Pages28 Page
-
File Size-