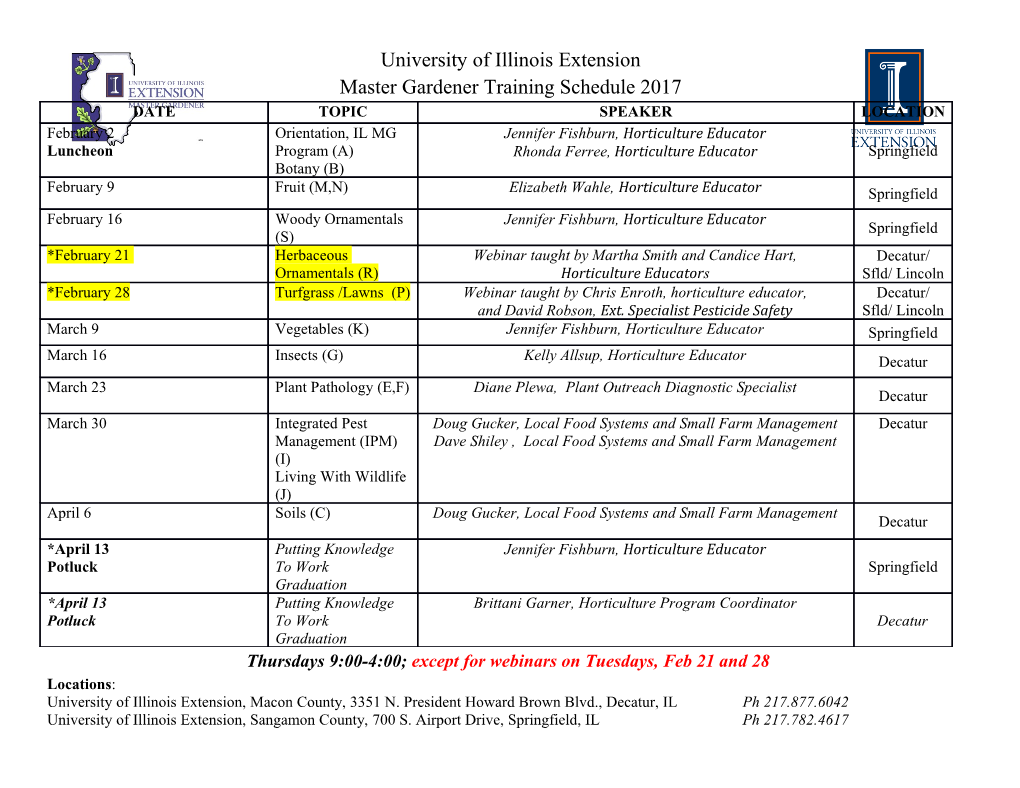
COMPARATIVE QUANTITATIVE GENETICS OF PROTEIN STRUCTURES: A COMPOSITE APPROACH TO PROTEIN STRUCTURE EVOLUTION by Jose Sergio Hleap Submitted in partial fulfillment of the requirements for the degree of Doctor of Philosophy at Dalhousie University Halifax, Nova Scotia December 2015 c Copyright by Jose Sergio Hleap, 2015 To my parents for all the support and the drive they instilled on me To my girlfriend for all the love and support during this process To my supervisor for the guidance provided And last but not least, To whomever had the patience to read this document ii Table of Contents Abstract ...................................... vi List of Abbreviations Used .......................... vii Acknowledgements ............................... x Chapter 1 Introduction .......................... 1 Chapter 2 Morphometrics of protein structures: Geometric mor- phometric approach to protein structure evaluation .. 4 2.1GMmethodsinproteinstructures.................... 8 2.1.1 Abstractingaproteinstructureasashape........... 8 2.1.2 GPSvsProteinstructurealignment............... 9 2.1.3 FormDifferenceinproteinstructures.............. 14 2.2 Applicability of GM-like methods .................... 20 2.2.1 Statistical analysis of the α-Amylase evolutionary variation . 21 2.2.2 StatisticalanalysisoftheNPC1proteinsimulation...... 29 Chapter 3 Defining structural and evolutionary modules in pro- teins: A community detection approach to explore sub- domain architecture ...................... 34 3.1 Landmark definition ........................... 37 3.2 Contact definition ............................. 37 3.2.1 In2Dsimulationdatasets.................... 37 3.2.2 Inproteinstructures....................... 37 3.3Graphconstruction............................ 38 3.3.1 Graphabstraction........................ 39 3.3.2 Community structure or clustering optimization ........ 39 3.4 Statistical significance test of clusters: Controlling the false positives . 40 3.4.1 Refinementofthemembershipvector.............. 41 3.5 Statistical power test of clusters: Acknowledging the false negatives probability ................................. 42 iii 3.6 Bootstrapping: Measuring the accuracy of sample estimates ..... 43 3.7Simulations................................ 47 3.7.1 Multivariatenormalsimulation................. 47 3.7.2 Structuredsimulation....................... 51 3.7.3 Proteinshapesimulation..................... 55 3.8Explorationofotherrealdatasets.................... 56 3.9 Concluding remarks ............................ 62 3.9.1 Putative meaning of the sub-domain architecture ....... 63 Chapter 4 The semantics of the modular architecture of protein structures ............................ 65 4.1 The emergence of modularity: natural selection and the self-organizing natureofproteins............................. 66 4.2 Domains as modules ........................... 69 4.2.1 Proteins as networks: Identifying domains by graph theory . 73 4.3 Sub-domain architecture ......................... 76 4.4 Exploring the hierarchy of protein structure architecture: Perspectives 79 Chapter 5 The response to selection in protein structures: A com- parative quantitative genetics approach ......... 82 5.1 Lynch’s comparative quantitative genetic model: Applications in pro- teinstructures............................... 85 5.1.1 Computational infeasibility of the full comparative approach . 87 5.1.2 Dealing with computational constraints: An approximation to theG-matrix........................... 91 5.1.3 Beyond the OTUs: partitioning the variance within taxonomic units ................................ 94 5.2 Overcoming over-parametrization: Approaching the G-matrix by means oftheP-matrix.............................. 96 5.2.1 The meaning of the pooled within-structure covariance matrix 98 5.3 Response to selection: The case of α-Amylase............. 99 5.3.1 Estimating dynamic and genetic variance-covariance matrices in the α-Amylasedataset.....................101 5.3.2 Concluding remarks ........................ 108 Chapter 6 Conclusion ............................110 Appendix A PDB codes and plot equivalences .............113 iv Appendix B PCoA using Cα as landmark ................116 Appendix C Feasibility of different phylogenetic mixed model imple- mentations ............................118 Appendix D BioMed Central license agreement .............124 Appendix E PLOS license ..........................128 Appendix F BENTHAM science Self-archiving policies and copyright agreement ............................130 F.1Self-archivingpolicies...........................130 F.2 Copyright agreement as per electronic mail: Grant of permission . 131 Bibliography ...................................133 v Abstract Structural biology has been long concerned about the emergence of protein structures and the convergence to particular folds. It can be said that protein structures are the realization of genetic information given thermodynamical and biological constraints. Given these properties, let’s refer to a structure as a phenotype. As such, protein structures can be analysed as shapes within a geometric morphometrics framework, and as a phenotype in a quantitative genetics framework. Here, I present a robust way to analyse protein structures statistically in either evolutionary or molecular dynamics sampling. I show how General Procrustes Analysis (GPA) can be applied to aligned molecular dynamics snapshots, and provide evidence that the scaling com- ponent of GPA is not applicable to protein structures. I also show how analysing protein structures as shapes can give insights into dynamic and evolutionary patterns. Analysing proteins as shapes also gives the possibility to apply known techniques to assess modularity. Traditional techniques have dimensionality limitations. I show how to overcome these limitations and propose a robust way to analyse protein struc- ture modularity. I show how a protein can be partitioned into biologically meaningful clusters, which can be used for description, protein prediction, or analysis of protein dynamics and evolution. The meaning of such modules is discussed further, and a hierarchical model for protein structure modularity is proposed. Also, methods to explore different kinds of modules at different kinds of hierarchy are explored. Finally, given that protein structures are phenotypes, the potential response to selection can be assessed by means of comparative quantitative genetics. I show that traditional comparative approaches have a heavy computational burden, therefore making the analysis infeasible. Nevertheless, similar approaches are developed to efficiently and accurately generate the estimations when the phenotypic variance is partitioned based on repeated measures, using a pooled-within covariance estimation. vi List of Abbreviations Used GM: Geometric Morphometrics GPS: Generalized Procrustes Superimposition PCA: Principal Component Analysis CVA: Canonical Variates Analysis PCoA: Principal Coordinate Analysis cMDS: Classical Multidimensional Scaling PLS: Partial Least Square Regression FM: Form Matrix FDM: Form Difference Matrix MATT: Multiple Alignment with Translations and Twists HOMSTRAD: HOMologous STRucture Alignment Database SABmark: Sequence Alignment Benchmark SCOP: Structural Classification of Proteins RMSD: Root Mean Square Deviation I: Most influential point FD: Form Difference/Form deformation PPA: Porcine Pancreatic Amylase PSI-BLAST: Position-Specific Iterative Basic Local Alignment Search Tool PDB: Protein Data Bank vii PFAM: Protein Families database GH13: Glycoside Hydrolase Family 13 ET: Evolutionary Trace HPA: Human Pancreatic Amylase TMA: Tenebrio molitor α-Amylase AHA: Pseudoalteromonas haloplanktis α-Amylase Arg: Arginine; also abbreviated as ARG or R Lys: Lysine; Also abbreviated as LYS or L Asn: Asparagine; Also abbreviated as ASN or N EC: Enzyme Commission ML: Maximum Likelihood GTR: General Time Reversible HGT: Horizontal Gene Transfer NPC1: Nieman-Pick Type C-1 MD: Molecular Dynamics PVP: Proportion of Variable with enough Power PCS principal components space LD: Linear Discriminants AFU: Autonomic Folding Units CATH: Class, Architecture, Topology, Homology database LGT: Lateral Gene Transfer viii RIN: Residue Interaction Networks PSN: Protein Structure Networks PK: Pyruvate Kinase RCN: Residue contact Networks AN: All Neighbours NSN: Non-Sequential Neighbours FA: Factor Analytic SEM: Structural Equations Modelling GLM: Generalized Linear Models PMM: Phylogenetic Mixed Models REML: Restricted Maximum Likelihood MCMC: Markov Chain Monte Carlo CPC: Common Principal Component RS: Random Skewers BGLMM: Bayesian Generalized Linear Mixed Models ix Acknowledgements I would like to especially thank my supervisor Christian Blouin, for teaching me more than expected, and for making my PhD experience as enjoyable as possible, for the lunch talks and for introducing me to Hive, Go and GURPS!. I would also like to thank Professors Norbert Zeh and Robert Beiko as well as the members of Dr. Beiko’s Lab in Dalhousie University for some helpful suggestions throughout the thesis, as well as the laughs, beers, camps, and all the extracurricular activities, to all of you thank you. To Wilson Chan, Alex Safatli, Kyle Nguyen, Simiao Lu, Tyler Brunet, and Jack Ryan for the collaboration in some parts of the thesis, but mainly for the Pepsi’s o’clocks, the 60’s Mondays and musical weeks, the laughs, and to made working more entertaining. To Liz Mackay for bearing with my writing and help me editing the manuscripts, and to her and her family
Details
-
File Typepdf
-
Upload Time-
-
Content LanguagesEnglish
-
Upload UserAnonymous/Not logged-in
-
File Pages174 Page
-
File Size-