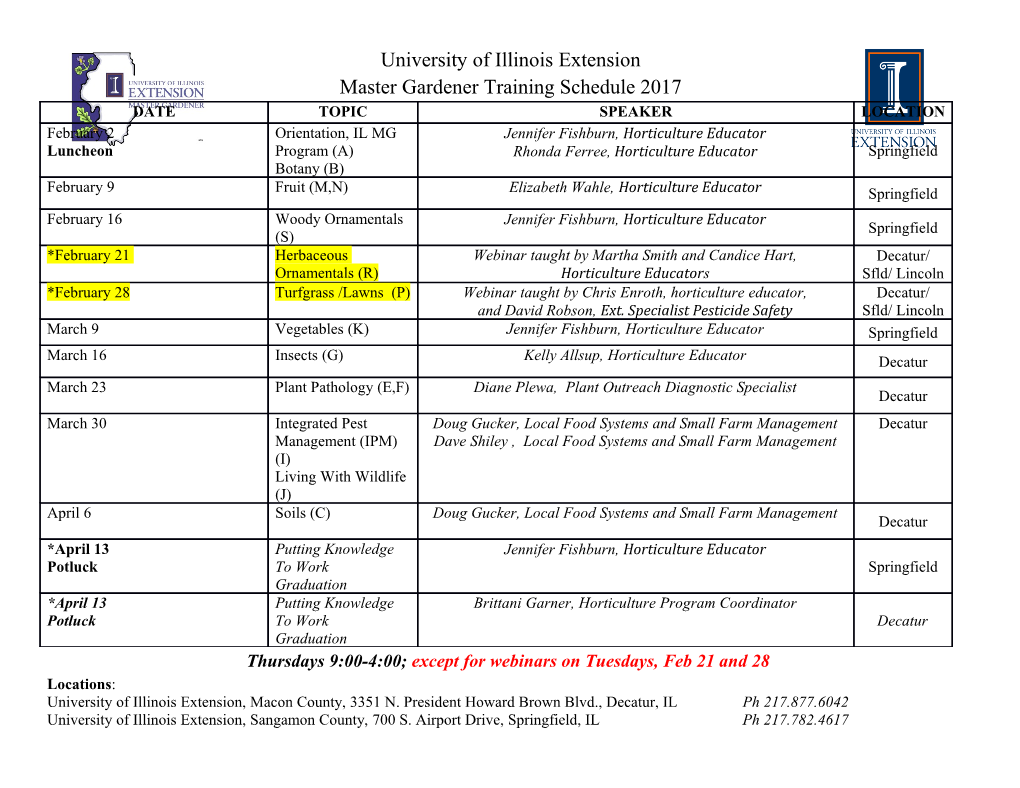
bioRxiv preprint doi: https://doi.org/10.1101/2021.08.21.457196; this version posted August 21, 2021. The copyright holder for this preprint (which was not certified by peer review) is the author/funder, who has granted bioRxiv a license to display the preprint in perpetuity. It is made available under aCC-BY-NC-ND 4.0 International license. 1 AlphaFold2 transmembrane protein structure prediction shines 2 3 Tamás Hegedűs1,2,*, Markus Geisler3, Gergely Lukács4, Bianka Farkas1,5 4 5 6 7 8 1 Department of Biophysics and Radiation Biology, Semmelweis University, Budapest, HU 9 2 TKI, Eötvös Loránd Research Network, Budapest, HU 10 3 Department of Biology, University of Fribourg, Fribourg, Switzerland 11 4 Department of Physiology and Biochemistry, McGill University, Montréal, Quebec, Canada 12 5 Faculty of Information Technology and Bionics, Pázmány Péter Catholic University, Budapest, HU 13 14 15 16 17 *Correspondence: Tamás Hegedűs 18 email: [email protected] 19 20 21 22 1 bioRxiv preprint doi: https://doi.org/10.1101/2021.08.21.457196; this version posted August 21, 2021. The copyright holder for this preprint (which was not certified by peer review) is the author/funder, who has granted bioRxiv a license to display the preprint in perpetuity. It is made available under aCC-BY-NC-ND 4.0 International license. 23 Abstract 24 Transmembrane (TM) proteins are major drug targets, indicated by the high percentage of prescription 25 drugs acting on them. For a rational drug design and an understanding of mutational effects on protein 26 function, structural data at atomic resolution are required. However, hydrophobic TM proteins often resist 27 experimental structure determination and in spite of the increasing number of cryo-EM structures, the 28 available TM folds are still limited in the Protein Data Bank. Recently, the DeepMind’s AlphaFold2 29 machine learning method greatly expanded the structural coverage of sequences, with high accuracy. 30 Since the employed algorithm did not take specific properties of TM proteins into account, the validity 31 of the generated TM structures should be assessed. Therefore, we investigated the quality of structures 32 at genome scales, at the level of ABC protein superfamily folds, and also in specific individual cases. We 33 tested template-free structure prediction also with a new TM fold, dimer modeling, and stability in 34 molecular dynamics simulations. Our results strongly suggest that AlphaFold2 performs astoundingly 35 well in the case of TM proteins and that its neural network is not overfitted. We conclude that a careful 36 application of its structural models will advance TM protein associated studies at an unexpected level. 2 bioRxiv preprint doi: https://doi.org/10.1101/2021.08.21.457196; this version posted August 21, 2021. The copyright holder for this preprint (which was not certified by peer review) is the author/funder, who has granted bioRxiv a license to display the preprint in perpetuity. It is made available under aCC-BY-NC-ND 4.0 International license. 37 Introduction 38 39 Although enormous resources were devoted to predict proteins’ structure for many decades, predicting a 40 protein structure from its sequence remained a challenging task1. There was a change in the last Critical 41 Assessment of Protein Structure Prediction (CASP) competition2 when two neural network based 42 approaches, RoseTTAFold3 and AlphaFold24 (AF2), were excelled. Importantly, DeepMind generated 43 AF2-predicted structures for the human5 and 20 other proteomes and they were deposited to EBI 44 (https://alphafold.ebi.ac.uk). Moreover, to ease the running of predictions for researchers, DeepMind6 45 and community Google Collaboration notebooks7 have been generated, albeit applying some 46 simplifications. AlphaFold2 was trained using multiple sequence alignments (MSA) and experimental 47 protein structures deposited before April 2018. Five different models were trained (e.g. with different 48 random seeds, with or without structural templates) to promote an increased diversity in structure 49 predictions6. The input for prediction is the sequence of a single protein chain, used for MSA generation 50 and structural template search. The quality of the resulted structural models is characterized by the mean 51 of per residue pLDDT (predicted Local Distance Difference Test) score (which takes values between 0 52 and 100, the higher value is better) and the structures are ranked accordingly4. The pLDDT confidence 53 measure predicts the accuracy of the Cα Local Distance Difference Test (lDDT-Cα) for the corresponding 54 prediction. Although this means that the high accuracy and reliability of AF2 observed in CASP14 can 55 be transferred to predicting the structure of any protein sequences (or whole proteomes)4,5, this has not 56 been validated yet and scientists do not have a clear indication how well AF2-predicted structures can be 57 trusted. Even more, there is a special skepticism in the field of transmembrane proteins, which are 58 challenging to investigate using either experimental or computational methods, especially because 59 AlphaFold2 was not tuned for TM proteins. It is also not known, whether the structural model with the 60 highest pLDDT score always corresponds to the native structure. In order to tackle these issues, we 61 investigated whether AF2-predicted human α-helical TM protein structures exhibit correctly located TM 3 bioRxiv preprint doi: https://doi.org/10.1101/2021.08.21.457196; this version posted August 21, 2021. The copyright holder for this preprint (which was not certified by peer review) is the author/funder, who has granted bioRxiv a license to display the preprint in perpetuity. It is made available under aCC-BY-NC-ND 4.0 International license. 62 regions. To demonstrate at a higher resolution that the predicted TM folds are native, we compared 63 predicted structures of the ATP Binding Cassette (ABC) superfamily from the AF2-predicted 21 64 proteomes to existing experimental ABC folds. ABC proteins play a role in important cellular processes 65 in all types of organisms and most of them transport substrates through the cell membrane in an ATP 66 dependent manner8–10. ABCC7/CFTR is a special member, which is an ATP-gated chloride channel and 67 includes a long intrinsically disordered regulatory R domain11,12. The functional form of ABC proteins is 68 built from two highly conservative nucleotide binding domains (NBDs) and two transmembrane domains 69 (TMDs) which can be encoded in one or separate peptide chains. The low conservation of their TMDs 70 are related to diverse functions and their currently known TM folds are also structurally divergent and 71 can be classified into eight groups (Pgp-, ABCG2-, MalFG-, BtuC-, EcfT-, LptFG-, MacB-, and MlaE- 72 like folds)13,14. Our results demonstrate that AlphaFold2 provides reliable protein structures also for 73 transmembrane proteins and can solve many issues associated with transmembrane protein structures. 74 75 Results 76 77 TM helices membrane topology assignments in AlphaFold2 structures 78 First, we split the human AF2 structures to soluble and transmembrane sets using the HTP (Human 79 Transmembrane Proteome) database15, calculated the mean pLDDT score for each protein, and plotted 80 their distribution (Fig. 1A and Fig. S1). Mean pLDDT values were also calculated separately for the TM 81 and non-TM regions of transmembrane proteins. Intriguingly, soluble proteins exhibited a broader 82 distribution and a significant area at lower pLDDT values compared to TM proteins. This was 83 unexpected, since the majority of the AlphaFold2 learning set inherently included more soluble protein 84 templates and the algorithm was not tuned for transmembrane proteins. However, correlation between 85 low pLDDT values and disordered segments was observed5, thus our observation strongly suggested that 86 more soluble proteins possess disordered regions than TM proteins. Interestingly, a very large portion of 4 bioRxiv preprint doi: https://doi.org/10.1101/2021.08.21.457196; this version posted August 21, 2021. The copyright holder for this preprint (which was not certified by peer review) is the author/funder, who has granted bioRxiv a license to display the preprint in perpetuity. It is made available under aCC-BY-NC-ND 4.0 International license. 87 TM regions (53%) were predicted with high pLDDT scores (>90) (Fig. 1A) suggesting that AF2 captured 88 the rules governing protein structures within the hydrophobic region. 89 Next, we compared the spatial localization of TM helices in AF structures corresponds with rational 90 and physiological helix orientation in a lipid bilayer slab by using the Constrained Consensus Topology 91 prediction (CCTOP) software16, which includes information from both experimental and computational 92 sources. We separated the start and end positions of predicted TM helices to two residue sets according 93 to their localization relative to the opposite sides of the bilayer. The distance between the center of 94 geometry of the two sets were calculated and its distribution is plotted (Fig. 1B). The majority of the 95 membrane thickness values were in the range between 20 and 30 Å that is in the range of the hydrophobic 96 region thickness. In order to support this finding with experimental data, the hydrophobic thickness of 97 experimentally determined human transmembrane protein structures was retrieved from the PDBTM 98 database17. The AF2 and experimental distribution largely overlapped (Fig. 1B). These observations 99 suggested that hydrophobic thickness values below 15Å and above 35Å may indicate an erroneous AF2 100 structure (12%, 725 out of 5952, Table S1). We also investigated the distribution of pLDDT scores versus 101 hydrophobic thickness (Fig. 1C). This plot indicated that AF2 structures with non-physiological thickness 102 values can process very high pLDDT scores, consequently, these scores alone may be insufficient to 103 select correct TM structures in blind predictions. 104 An inaccurate TM topology prediction of CCTOP may provide an outlier hydrophobic thickness in 105 the case of a correct AF2-predicted structure.
Details
-
File Typepdf
-
Upload Time-
-
Content LanguagesEnglish
-
Upload UserAnonymous/Not logged-in
-
File Pages24 Page
-
File Size-