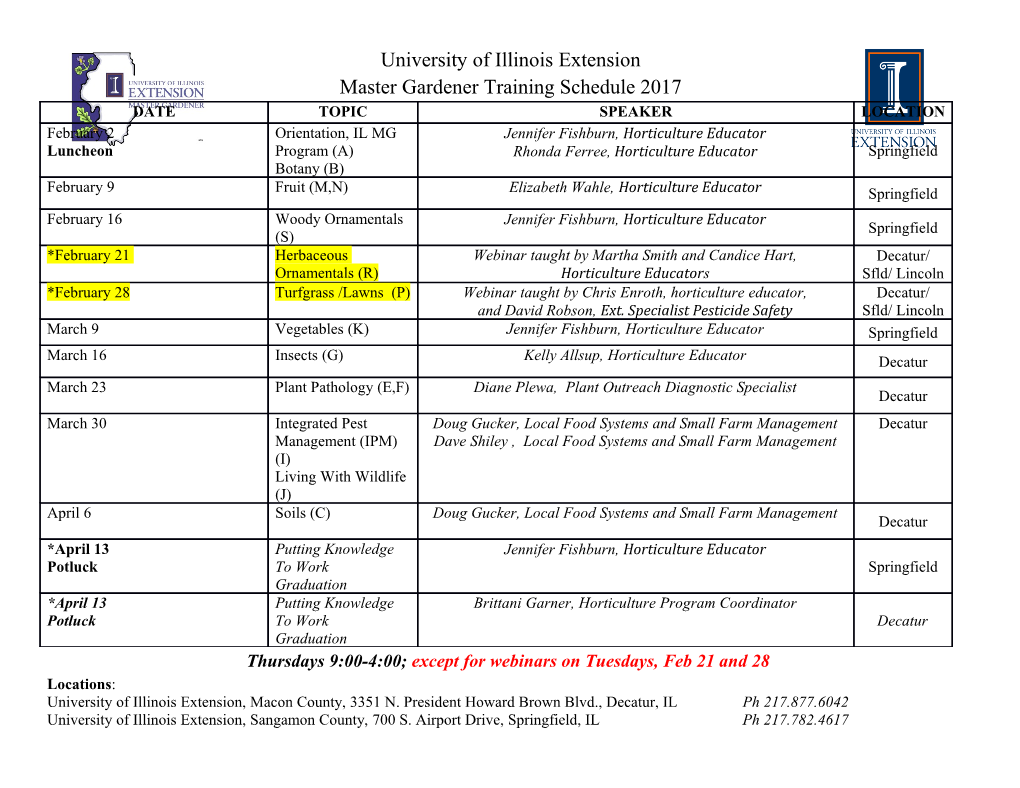
© Statistical Research and Training Center دوره ی ١٣،ﺷﻤﺎره ی ٢، ﭘﺎﯾﯿﺰ و زﻣﺴﺘﺎن ١٣٩۵، ﺻﺺ ١٣١–١۵٣ J. Statist. Res. Iran 13 (2016): 131–153 DOI: 10.18869/acadpub.jsri.13.2.131 The Lomax-Exponential Distribution, Some Properties and Applications Nasrin Hami Golzary, Masoud Ganjiy;∗ and Hossein Bevraniz y University of Mohaghegh Ardabili z University of Tabriz Received: 1/21/2016 Approved: 1/28/2017 Abstract. The exponential distribution is a popular model in applica- tions to real data. We propose a new extension of this distribution, called the Lomax-exponential distribution, which presents greater flexibility to the model. Also there is a simple relation between the Lomax-exponential dis- tribution and the Lomax distribution. Results for moment, limit behavior, hazard function, Shannon entropy and order statistic are provided. To esti- mate the model parameters, the method of maximum likelihood and Bayse estimations are proposed. Two data sets are used to illustrate the applica- bility of the Lomax-exponential distribution. Keywords. T-X family; Lomax distribution; Shannon entropy; simulation study. MSC 2010: 62E15; 60E05. 1 Introduction The exponential and Lomax distributions are two important distributions in statistical practice. They possess several important statistical properties * Corresponding author 132 The Lomax-Exponential Distribution ::: and exhibit great mathematical tractability. History of exponential density function has been obtained in Balakrishnan and Basu (1995). Gupta and Kundu (1999a) introduced generalized exponential density function. Then Gupta and Kundu (1999b) derived some properties of generalized exponen- tial density function. The Lomax distribution, conditionally also called the Pareto Type II distribution, is used in business, economics, actuarial science and etc. It is essentially a Pareto distribution that has been shifted so that its support begins at zero. An extended Lomax distribution was introduced by Lemonte and Cordeiro (2011). Alzaatreh et al. (2013a) proposed the T-X families of distributions. These families of distributions were used to generate a new class of dis- tributions which offer more flexibility in modeling a variety of data sets. Various distributions can be driven by using this method. The gamma- half normal and the gamma-normal distributions were introduced by Alza- atreh and Knight (2013) and Alzaatreh et al. (2014) respectively. Also the gamma-Pareto and Weibull-Pareto distributions were studied by Alzaatreh et al. (2012) and Alzaatreh et al. (2013b) respectively. The gamma-uniform and logistic-uniform distributions were introduced by Torabi and Montaz- eri (2012, 2014). In this paper new distribution, Lomax-exponential will be introduced by using of the method of T-X family. Let F (x) be the cumulative distribution function (c.d.f.) of any random variable X. Also let r(t) and R(t) be the probability density function (p.d.f.) and c.d.f. of a random variable T defined on (0; 1) respectively. The c.d.f. of the T-X family of distributions defined by Alzaatreh et al. (2013a) is given by Z F (x) ( ) 1−F (x) F (x) G(x) = r(t)dt = R : (1) 0 1 − F (x) When X is a continuous random variable, the probability density function of the T-X family is ( ) f(x) F (x) g(x) = r : (2) (1 − F (x))2 1 − F (x) Let a random variable T follows the Lomax distribution with p.d.f. and c.d.f. respectively for t > 0; k; a > 0 as © 2016, SRTC Iran N. Hami Golzar, M. Ganji and H. Bevrani 133 kak r(t) = (3) (t + a)k+1 and a R(t) = 1 − ( )k: (4) t + a Then g(x) in (2) leads to the Lomax-X family with p.d.f. kakf(x)(1 − F (x))k−1 g(x) = : (5) [F (x) + af1 − F (x)g]k+1 The Lomax-exponential distribution (LED for short) is obtained if X follows the exponential distribution with parameter s. The corresponding c.d.f. and p.d.f. of X are respectively defined for k; a; s > 0 and x > 0 as ( ) a k G(x) = 1 − (6) esx + a − 1 and skakesx g(x) = : (7) (esx + a − 1)k+1 When k = a = 1, the LED reduces to the exponential distribution. Then the LED is a generalized of the exponential disreibution. 2 Properties of the Lomax-Exponential Distribu- tion In this section some general properties of the LED will be addressed in- cluding the limiting behavior, Shannon entropy, quantile function and etc. Lemmas 1 and 2 give the relations between the LED, Lomax and Pareto type 1 distributions. Lemma 1. If a random variable X follows the LED with parameters (k; a; s), then the random variable Y = esX − 1 follows the Lomax distribution with J. Statist. Res. Iran 13 (2016): 131–153 134 The Lomax-Exponential Distribution ::: parameters (k; a). Also Y = esX +a−1 follows the Pareto type 1 distribution with parameters (k,a). Proof. The result follows by using the transformation technique. Lemma 2. If a random variable X follows the Lomax distribution with 1 parameters (k; a), then the random variable V = s log(X + 1) follows the LED with parameters (k; a; s). Proof. The result follows by using the transformation technique. In Figure 1, various graphs of g(x) are provided. The p.d.f. of LED may take on a variety of shapes for different values of k, a and s. It can be right-skewed, approximately symmetric or reversed-J shape. The limit of ! ! 1 skak the p.d.f. of LED as x 0 and x are ak+1 and 0, respectively. The Figure 1 confirms these limits. (k = 4, a = 1, s = 2) (k = 4, a = 0.5, s = 2) (k = 9, a = 5, s = 0.25) (k = 4, a = 8, s = 1) (k = 2, a = 2, s = 2) (k = 0.2, a = 10, s = 7) (k = 0.25, a = 7, s = 5) (k = 0.5, a = 7, s = 2) g(x) 0.0 0.5 1.0 1.5 0 1 2 3 4 x Figure 1. The p.d.f. of LED for various value of k, a and s The hazard function associated with the LED is g(x) skesx h(x) = = : (8) 1 − G(x) esx + a − 1 © 2016, SRTC Iran N. Hami Golzar, M. Ganji and H. Bevrani 135 In Figure 2, various graphs of h(x) are provided for various values of k, a and s. It is clear that when a > 1, the hazard function is increasing, when a < 1, the hazard function is decreasing and when a = 1, the hazard function is fixed (= sk). The limits of the hazard function of the LED as x ! 0 and ! 1 sk x + are a and sk, respectively. (k = 1.5, a = 1, s = 2) (k = 1.5, a = 0.5, s = 2) (k = 1, a = 5, s = 2) (k = 4, a = 8, s = 1) (k = 1.5, a = 2, s = 2) (k = 0.5, a = 0.25, s = 1) h(x) 0 2 4 6 8 0 1 2 3 4 x Figure 2. The hazard function of LED for various value of k, a and s Theorem 1. The LED has a unique mode at x = x0. When a > k + 1, the 1 a−1 mode is x0 = s log k and when a < k + 1 the mode is x0 = 0. Proof. The mode of the LED can be obtained by taking the derivative of g(x) with respect to x, (g0) and by setting it to zero. It is easy to see g0 > 0 1 a−1 0 1 a−1 for 0 < x < s log k and g < 0 for x > s log k . 2.1 Quantile Function The quantile function of the LED, Q(λ), 0 < λ < 1 is obtained by solving G(Q(λ)) = λ. It implies that J. Statist. Res. Iran 13 (2016): 131–153 136 The Lomax-Exponential Distribution ::: ( ) 1 a Q(λ) = log p − a + 1 : s k 1 − λ The median of the LED is obtained as 0 1 1 1 a Median = Q( ) = log @ q − a + 1A : (9) 2 s k 1 2 2.2 Moment The moment generating function for the LED is given by Z Z 1 1 (t+s)x tX tx k e MX (t) = E(e ) = e g(x)dx = ska sx k+1 dx: 0 0 (e + a − 1) By defining y = esx + a − 1 Z 1 t − s k (y a + 1) MX (t) = ka k+1 dy: a y Using Taylor expansion t − t t − s 2 − 2 t t t t −1 s ( s 1)y (1 a) (y − a + 1) s = y s + y s (1 − a) + + ··· s 2 t t t −1 ' y s + y s (1 − a): s Then Z 1 t (y − a + 1) s M (t) = kak dy X yk+1 Za 1 t t t −1 y s + y s (1 − a) ' k s ka k+1 dy ( a y ) t t −1 a s (1 − a)ta s = k + ; (10) − t − t k s s(k + 1 s ) © 2016, SRTC Iran N. Hami Golzar, M. Ganji and H. Bevrani 137 provided t < ks. The derivative of (10) with respect to t is given by ! t t t @ k a s (k − ) log a + a s M (t) = s @t X s (k − t )2 " s # t −1 t −1 t t −1 k (1 − a)f(sa s + ta s log a)(k + 1 − ) + ta s g + s : − t 2 s (k + 1 s ) The first non-central moment follows by setting t=0, k log a + 1 k(1 − a) E (X) = + : (11) LED sk as(k + 1) By defining k = a = 1 in (11), the first non-central moment of the exponential distribution is obtained as 1 E (X) = : LED s Simultaneously, the equation (11) works well in Subsection 2.7.
Details
-
File Typepdf
-
Upload Time-
-
Content LanguagesEnglish
-
Upload UserAnonymous/Not logged-in
-
File Pages23 Page
-
File Size-