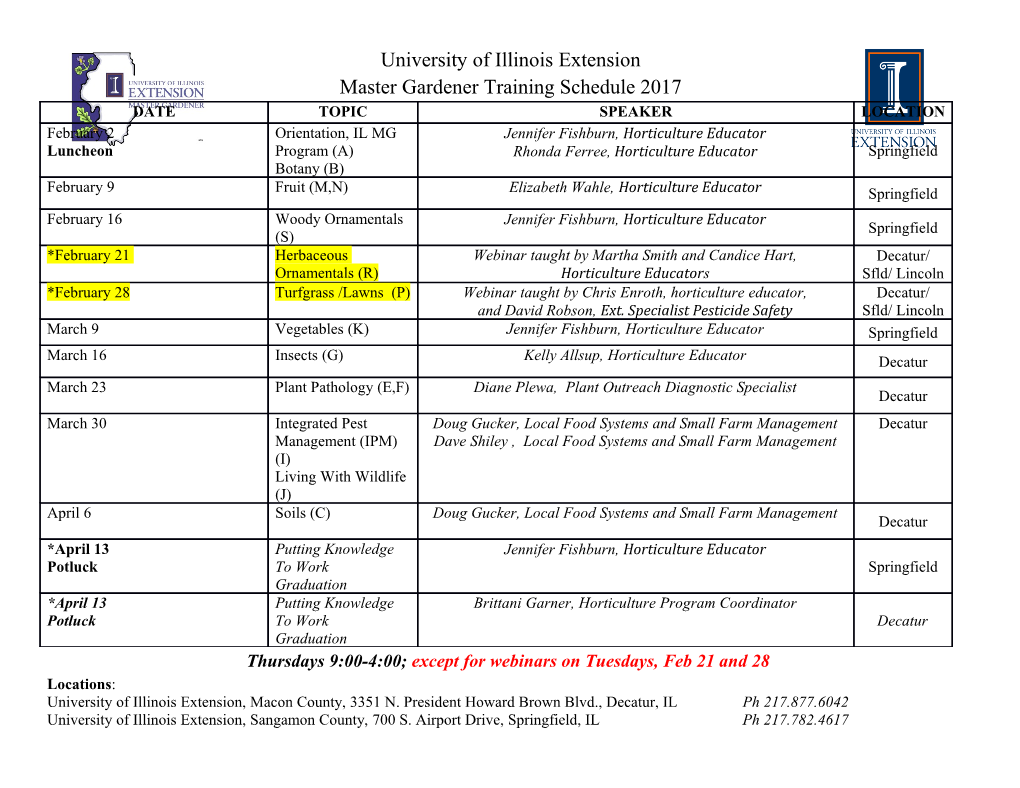
Neural Machine Translation for Extremely Low-Resource African Languages: A Case Study on Bambara Allahsera Auguste Tapo1;∗, Bakary Coulibaly2, Sébastien Diarra2, Christopher Homan1, Julia Kreutzer3, Sarah Luger4, Arthur Nagashima1, Marcos Zampieri1, Michael Leventhal2 1Rochester Institute of Technology 2Centre National Collaboratif de l’Education en Robotique et en Intelligence Artificielle (RobotsMali) 3Google Research, 4Orange Silicon Valley ∗[email protected] Abstract population have access to “encyclopedic knowl- edge” in their primary language, according to a Low-resource languages present unique chal- 2014 study by Facebook.2 MT technologies could lenges to (neural) machine translation. We dis- help bridge this gap, and there is enormous interest cuss the case of Bambara, a Mande language in such applications, ironically enough, from speak- for which training data is scarce and requires ers of the languages on which MT has thus far had significant amounts of pre-processing. More than the linguistic situation of Bambara itself, the least success. There is also great potential for the socio-cultural context within which Bam- humanitarian response applications (Öktem et al., bara speakers live poses challenges for auto- 2020). mated processing of this language. In this pa- Fueled by data, advances in hardware technol- per, we present the first parallel data set for ogy, and deep neural models, machine translation machine translation of Bambara into and from (NMT) has advanced rapidly over the last ten years. English and French and the first benchmark re- Researchers are beginning to investigate the ef- sults on machine translation to and from Bam- bara. We discuss challenges in working with fectiveness of (NMT) low-resource languages, as low-resource languages and propose strategies in recent WMT 2019 and WMT 2020 tasks (Bar- to cope with data scarcity in low-resource ma- rault et al., 2019), and in underresourced African chine translation (MT). languages. Most prominently, the Masakhane (8 et al., 2020) community3, a grassroots initiative, 1 Introduction has developed open-source NMT models for over 30 African languages on the base of the JW300 Underresourced languages, from a natural lan- corpus (Agic´ and Vulic´, 2019), a parallel corpus of guage processing (NLP) perspective, are those religious texts. lacking the resources (large volumes of parallel Since African languages cover a wide spectrum bitexts) needed to support state-of-the-art perfor- of linguistic phenomena and language families mance on NLP problems like machine translation, (Heine and Nurse, 2000), individual development automated speech recognition, or named entity of translations and resources for selected languages recognition. Yet the vast majority of the world’s or language families are vital to drive the over- languages—representing billions of native speak- all progress. Just within the last year, a number ers worldwide—are underresourced. And the lack of dedicated studies have significantly improved of available training data in such languages usually the state of African NMT: van Biljon et al.(2020) reflects a broader paucity of electronic information analyzed the depth of Transformers specifically resources accessible to their speakers. for low-resource translation of South-African lan- For instance, there are over six million guages, based on prior studies by Martinus and Wikipedia articles in English but fewer than sixty Abbott(2019) on the Autshumato corpus (Groe- thousand in Swahili and fewer than seven hundred newald and du Plooy, 2010). Dossou and Emezue in Bambara, the vehicular and most widely-spoken (2020) developed an MT model and compiled re- native language of Mali that is the subject of this sources for translations between Fon and French, paper.1 Consequently, only 53% of the worlds 2fbnewsroomus.files.wordpress.com/ 1meta.wikimedia.org/wiki/List_of_ 2015/02/state-of-connectivity1.pdf Wikipedias. 3masakhane.io 23 Proceedings of the 3rd Workshop on Technologies for MT of Low Resource Languages, pages 23–32 Decmber 04, 2020. c 2020 Association for Computational Linguistics Akinfaderin(2020) modeled translations between from social media or text messages, and these are English and Hausa, Orife(2020) for four languages a written in a melange of French, Bambara and of the Edoid language family, and Ahia and Ogueji Arabic. Consequently, corpora based on common (2020) investigated supervised vs. unsupervised Bambara usage must account for the code switch- NMT for Nigerian Pidgin. ing found in these mixtures. In this paper, we present the first parallel data set Most of these characteristics are shared with re- for machine translation of Bambara into and from lated languages, e.g., a subset of the Mande family English and French and the first benchmark results of languages, where many languages are mutually on machine translation to and from Bambara. We intelligible. Thus, our hope is that our approach discuss challenges in working with low-resource will be transferable to the other twelve official local languages and propose strategies to cope with data languages of Mali, or to other African languages scarcity in low-resource MT. We discuss the socio- with a comparable socio-cultural and linguistic em- cultural context of Bambara translation and its im- bedding, for example Wolof (non-Mande), which plications for model and data development. Finally, is comparable in terms of number of speakers, bor- we analyze our best-performing neural models with rowings from Arabic and French influence, and a small-scale human evaluation study and give rec- oral traditions. ommendations for future development. We find The next section will provide more details on that the translation quality on our in-domain data digital resources and describe the process of ex- set is acceptable, which gives hope for other lan- ploring and collecting data and choosing parallel guages that have previously fallen under the radar corpora for the training of the NMT model. of MT development. We released our models and data upon publica- 3 Data Collection tion4. Our evaluation setup may serve as bench- 3.1 Bambara Corpora mark for an extremely challenging translation task. We discovered that there has been no prior devel- 2 The Bambara Language opment of automatic translation of Bambara, de- spite a relatively large volume of research on the Bambara is the first language of five million peo- language (Culy, 1985; Aplonova and Tyers, 2017; ple and the second language of approximately ten Aplonova, 2018). As a pilot study for assessing million more. Most of its speakers are members of the potential for automatic translation of Bambara, Bambara ethnic groups, who live throughout the Leventhal et al.(2020) crowdsourced a small set African continent. Approximately 30–40 million of written or oral translations from French to Bam- people speak some language in the Mande family bara. Additional work was carried out exploring of languages, to which Bambara belongs (Lewis novel crowdsourcing strategies for data collection et al., 2014). in Mali Luger et al.(2020). Bambara is a tonal language with a rich mor- The Corpus Bambara de Référence (Vydrin phology. Over the years, several competing writing et al., 2011) is the largest collection of electronic systems have developed, however, as an histori- texts in Bambara. It includes scanned and text- cally predominately oral language, a majority of based electronic formats. A number of parallel Bambara speakers have never been taught to read texts based on this data exist. For example, Vydrin or write the standard form of the language. Many (2018) analyzed Bambara’s separable adjectives are incapable of reading or writing the language at using this data. all. The standardization of words and the coinage To survey the known available sources of parallel of new ones are still works in progress; this poses texts with Bambara, we consulted with a number challenges to automated text processing. of authorities on Bambara, including the Academie During Muslim expansion and French coloniza- Malienne des Langues (AMALAN) in Mali and tion, Arabic and French mixed with local lan- the Institut National des Langues et Civilisations guages, resulting in a lingua franca, e.g., Urban Orientales (INALCO) in France, as well as a num- Bambara. Most of the existing Bambara resources ber of individual linguists and machine translation are cultural (folk stories or news/topical) or come experts throughout the world. These two organ- 4https://github.com/israaar/mt_ isations play key roles in the definition and the bambara_data_models promotion of a standard form of written Bambara 24 through the collection and annotation of corpora, Bambara French English the publishing of dictionaries, and, in formulating glosses 3,548 4,847 4,855 recommendations for language policy in Mali. examples 2,023 2,021 2,021 Our efforts uncovered several sources of parallel Dict. aligned 2,158 2,146 2,158 texts between Bambara and French and/or English that are listed in Table5 in AppendixA. The table chapters 27 provides a rating of each of the identified resources, files 336 and the rationale why they were in- or excluded paragraphs 9,336 9,367 9,356 from our translation study. Ultimately, most of unigrams 8,209 9,893 6,935 these resources proved either of very little or no Medical bigrams 26,430 25,746 31,412 practical use as sources of training data. Many trigrams 5,816 11,312 21,398 did not actually contain aligned texts and some not stopwords 147 123 69 even suitable monolingual text. A systematic problem was lack of adherence to Table 1: The dictionary data set from SIL Mali and the the standardized Bambara orthography, due to it medical health guide “Where there is no doctor” with being a predominately oral language. This is also examples in French, English, and Bambara. one of the reasons why our search for parallel data on the web generally did not yield many finds— their translations in French and in English. Most of commonly being used in written form, Bambara these are single sentences, so there is sentence-to- is used even less on the web.
Details
-
File Typepdf
-
Upload Time-
-
Content LanguagesEnglish
-
Upload UserAnonymous/Not logged-in
-
File Pages10 Page
-
File Size-