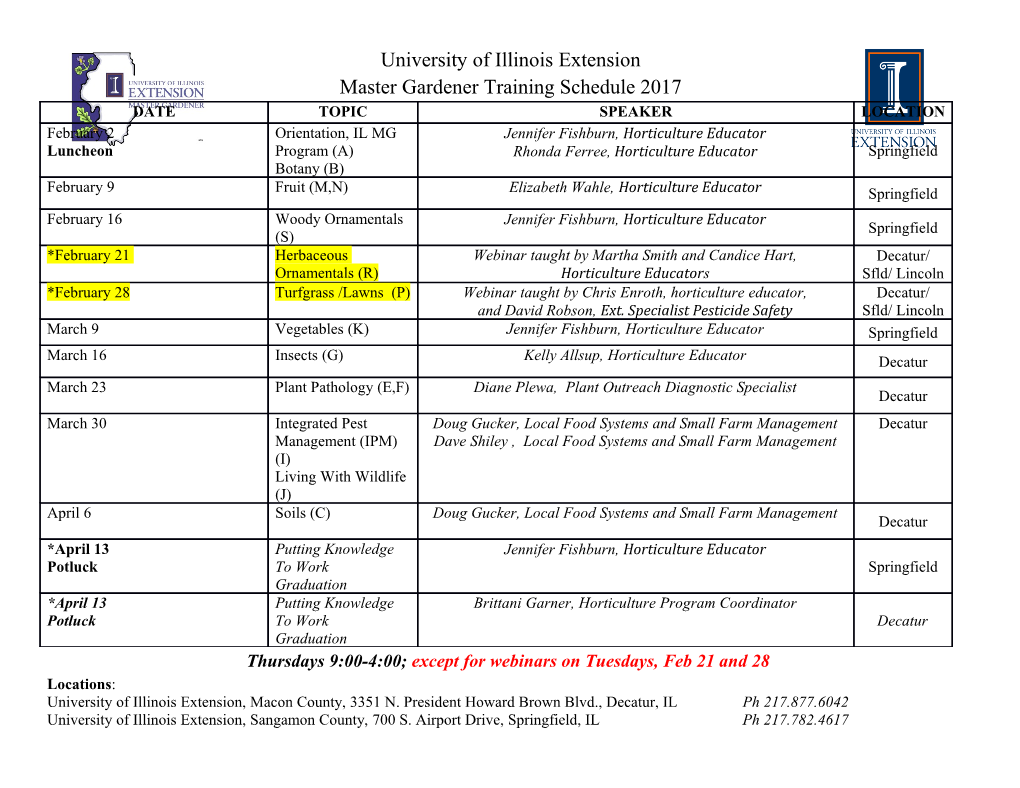
An Overview of Deep Learning Strategies for Time Series Prediction Rodrigo Neves Instituto Superior Tecnico,´ Lisboa, Portugal [email protected] June 2018 Abstract—Deep learning is getting a lot of attention in the last Jenkins methodology [2] and were developed mainly in the few years, mainly due to the state-of-the-art results obtained in area of econometrics and statistics. different areas like object detection, natural language processing, Driven by the rise of popularity and attention in the area sequential modeling, among many others. Time series problems are a special case of sequential data, where deep learning models of deep learning, due to the state-of-the-art results obtained can be applied. The standard option to this type of problems in several areas, Artificial Neural Networks (ANN), which are are Recurrent Neural Networks (RNNs), but recent results are non-linear function approximator, have also been receiving an supporting the idea that Convolutional Neural Networks (CNNs) increasing attention in time series field. This rise of popularity can also be applied to time series with good results. This raises is associated with the breakthroughs achieved in this area, with the following question - Which are the best attributes and architectures to apply in time series prediction problems? It was the successful application of CNNs and RNNs to sequential assessed which is the current state on deep learning applied to modeling problems, with promising results [3]. RNNs are a time-series and studied which are the most promising topologies type of artificial neural networks that were specially designed and characteristics on sequential tasks that are worth it to to deal with sequential tasks, and thus can be used on time be explored. The study focused on two different time series series. They have shown promising results in the area of time problems, wind power forecasting and predictive maintenance. Both experiments were conducted under the same conditions series forecasting [4] and predictive maintenance [5]. across different models to guarantee a fair comparison basis. CNNs that are a different type of neural network, originally The study showed that different models and architectures can designed to deal with images, are also being applied to be applied on distinct time series problems with some level of sequence modeling with very promising results [6]. The recent success, thus showing the value and versatility of deep learning advances in sequence tasks like, natural language processing models in distinct areas. The results also showed that CNNs, together with recurrent architectures, are a viable option to apply [7], speech recognition [8], machine translation [9], are being in time series problems. adopted to be applied in time series problems [10]. These advances in sequence modeling within the area of I. INTRODUCTION deep learning are enormous which makes it overwhelming, being complicated to follow all new advances and research Time series modeling has always been under intense de- directions. In the area of sequence modeling the default option velopment and research in statistics, and more recently, in was always to make use of recurrent models like Long-Short the machine learning area. Time series data often arises in Term Memory (LSTM) and Gated Recurrent Unit (GRU) to different areas, such as economics, business, engineering and capture and model the inherent sequential dependences. Re- many others, and can have different applications. It is possible cently, supported by new paths of research convolutional-based to obtain an understanding of the underlying structure that is models are reaching state-of-the-art results in fields like audio produced by the observed data or to fit a model in order to synthesis or machine translation. A study [11] was made to make predictions about the future. For example, in the energy asses the performance of a convolutional model in sequential sector is of extreme priority to know in advance which will tasks where the authors found that ”a simple convolutional be the power consumed in the next days, or which will be the architecture outperforms canonical recurrent networks such as amount of energy generated from renewable sources. In the LSTMs across a diverge range os tasks and datasets, while retail sector, every company wants to know, in advance which demonstrating longer effective memory”. are going to be their sales, or how many pieces of a certain In this work we present a systematic and fair comparison product will sell to make decisions on how they should manage among different deep learning approaches that are possible to their portfolio. In the heavy industry, a single machine failure use in time series problems, with the objective of assessing can lead to enormous losses, and thereby, if that fault could which are the best characteristics and topologies that we be predicted in advance, could save both time and money. should aim to have in a model. This task was performed in two Several techniques and models can be applied on the field problems: wind power generation forecasting and predictive of time series predictions, being the most known ones from the maintenance areas, where we used and compared different AutoRegressive Integrated Moving Average (ARIMA) family recurrent and convolutional architectures. models. These models were greatly influenced by the Box- The results suggests that convolutional-based algorithms, together with recurrent networks, should be seen as a good only learn one set of parameters, rather than learning a option to use in time series problems. This is supported by different set of parameters for every location. Due to parameter the results obtained on both problems, where we could see sharing CNNs have a property called invariance to translation, that convolutional-based models were able to match and even meaning that if the input changes the output changes in the outperform recurrent models. same way. A typical layer of a convolutional network consists of three II. BACKGROUND stages. In the first stage the layer performs several convolutions Machine learning, and especially deep learning, have been operations in parallel, where is produced distinct features under great development in the last few years. Machine maps. The second stage performs an activation function to learning is a subfield of artificial intelligence (AI), where the each linear activation, introducing an non-linearity. In the third systems have the ability to acquire knowledge, by extracting stage, it is used a pooling function to further modify the output patters from raw data, learning from it, and then making of the layer. This modification introduced by the pooling a determination or prediction about the application that it function replaces the nets output with a summary statistic of was designed for. Deep learning, which in turn is, a subfield nearby outputs. The most used ones are the max and average of machine learning, is mainly based on an algorithm that pooling operations. is known as neural network. Neural networks are able to B. Recurrent Neural Network build complex relationships, extracting the information from RNNs are a type of neural networks that have recurrent data, without any previous knowledge. Theoretically neural connections and are specially designed for processing sequen- networks are universal functions approximator [1] and they tial data. One key characteristic of RNNs is the use of shared can represent any function. CNNs are a type of neural network parameters across all sequence. Instead of having a different that was inspired on convolution operations and was specially set of parameters to process each sequence step, the same set designed to be used with data that has a grid-like topology. is used across all sequence, allowing the model to generalize RNNs are specifically designed to deal with sequential tasks, across sequences not seen during training. Parameter sharing due to the recurrent connections that they have. is also important when a specific piece of information can A. Convolution Neural Network occur at multiple positions within the sequence. The parameter CNNs are a family of neural networks that uses convolution sharing used in recurrent networks relies on the assumption operation in place of general matrix multiplication, in at least that the same parameters can be used for different time steps. on of their layers. The typical convolution operation applied RNNs can be formally introduced as a set of operations in CNNs is shown in equation 1, where I is the input and K represented by a directed acyclic computational graph, as in is known as the kernel. The output is refereed as the feature Figure 1. The recursive operation in a RNN is represented by h(t) x(t) map. equation 2, where is the hidden state and the input at step t. The additional layers seen in Figure 1 adds further transformations to the data. X X S(i; j) = (I∗K)(i; j) = I(m; n)K(i−m; j−n) (1) (t) (t−1) (t) m n h = f(h ; x ; θ) (2) Convolution operation leverages three important aspects that When the recurrent network is trained to perform a task can help improving a machine learning system: sparse inter- that requires predicting the next step based on previous values, actions, parameters sharing and equivariant representations. the network typically learns to use the hidden state, h(t), as Convolution also provides a way of working with variable a summary of task-relevant aspects from the past sequence input sizes. In shallow neural networks every input interacts input. with every output, but that does not happen in CNNs. For example, when processing an image, the input might have thousands or millions of pixels, but it is possible to detect small, meaningful features, such as edges, with kernels that occupy just tens or hundreds of pixels. This means that it is needed to store less parameters, which both reduces the memory requirements of the model and improves its statistical efficiency.
Details
-
File Typepdf
-
Upload Time-
-
Content LanguagesEnglish
-
Upload UserAnonymous/Not logged-in
-
File Pages10 Page
-
File Size-