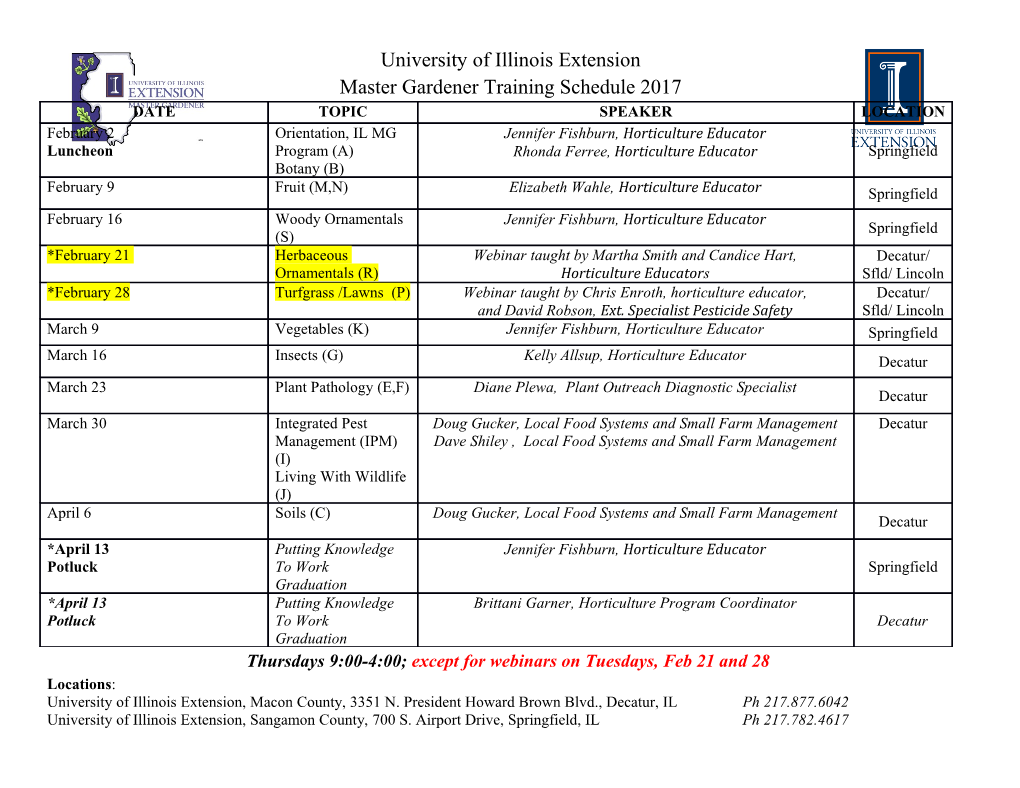
Functional Analysis Lyudmila Turowska Department of Mathematical Sciences Chalmers University of Technology and the University of Gothenburg These lectures concern the theory of normed spaces and bounded linear oper- ators between normed spaces. We shall define these terms and study their prop- erties. You can consult the following books. 1. G. Folland: Real Analysis. Modern Techniques and their Applications, John Wiley & Sons, 1999, Chapters 5-7 and parts of Chapter 4. 2 1 Vector Spaces Definition 1.1. A complex vector space (or a vector space over the field C) (or a complex linear space) is a set V with addition V × V ! V; (x; y) 7! x + y the sum of x and y; and scalar multiplication C × V ! V; (λ, x) 7! λx the product of λ and x; satisfying the following rules: x + (y + z) = (x + y) + z 8x; y; z 2 V; x + y = y + x 8x; y 2 V; 90 2 V : 0 + x = x 8x 2 V; 8x 2 V 9 − x 2 V : x + (−x) = 0; λ(x + y) = λx + λy 8x; y 2 V; 8λ 2 C; (λ + µ)x = λx + µx 8x 2 V; 8λ, µ 2 C; λ(µx) = (λµ)x 8x 2 V; 8λ, µ 2 C; 1x = x 8x 2 V: It is easy to see that the usual arithmetic holds in V . Remark 1.2. Similarly, we can define a real vector space by replacing C with R in the definition. n Examples 1.3. 1. C = f(x1; : : : ; xn): xi 2 C 8ig, the complex vector space of n-tuples of complex numbers with coordinatewise addition n (x1; : : : ; xn) + (y1; : : : ; yn) = (x1 + y1; : : : ; xn + yn); (x1; : : : ; xn); (y1; : : : ; yn) 2 C and coordinatewise scalar multiplication n λ(x1; : : : ; xn) = (λx1; : : : ; λxn); λ 2 C; (x1; : : : ; xn) 2 C : 2. An infinite-dimensional complex vector space: N C = f(x1; : : : ; xn;:::): xi 2 C 8ig 3 is the complex vector space of sequences of complex numbers with coordinatewise addition and scalar multiplication: x + y = (x1 + y1; : : : ; xn + yn;:::); λx = (λx1; : : : ; λxn;:::); N x = (x1; : : : ; xn;:::); y = (y1; : : : ; yn;:::) 2 C ; λ 2 C: 3. The complex vector space of all continuous complex-valued functions on [0; 1] C[0; 1] = ff : [0; 1] ! C : f is continuous on [0; 1]g with pointwise addition (f + g)(t) = f(t) + g(t); f; g 2 C[0; 1]; t 2 [0; 1]; and scalar multiplication (λf)(t) = λf(t); f 2 C[0; 1]; λ 2 C; t 2 [0; 1]: These operations are well-defined due to the following fact (from the basic analysis course): If f; g are continuous functions on [0; 1] and λ 2 C then (f + g) and λf are continuous on [0; 1]. C f n 2 C g × 4. Mn( ) = (aij)i;j=1 : aij ; i; j = 1; : : : ; n , the complex vector space of n n matrices with complex entries with addition n n n (aij)i;j=1 + (bij)i;j=1 = (aij + bij)i;j=1; and scalar multiplication n n λ(aij)i;j=1 = (λaij)i;j=1: (So we have addition and scalar multiplication by entries.) 1.1 Vector Subspaces Definition 1.4. A non-empty subset of W of a vector space V is called a vector subspace (or a linear subspace) if 1. for every x; y 2 W; x + y 2 W ; 2. for every x 2 W and every λ 2 C (or R), λx 2 W . Remark 1.5. A vector subspace W is itself a vector space with respect to the addition and scalar multiplication defined on V . 4 Question 1.6. Let D be the closed unit disc, that is D = fz 2 C : jzj ≤ 1g: It is obvious that D is a subset of C. Is D a vector subspace of C? No. Conditions (1) and (2) of the definition of a vector subspace are not satisfied: 1. For z1 = z2 = 1, we have z1 + z2 = 2, so jz1 + z2j = 2. Thus z1 + z2 2= D. 2. For z = i, we have jλzj = jλj for every λ 2 C. Thus λz2 = D when λ is such that jλj > 1. R f 2 1 g Example 1.7. Show that W = f C[0; 1] : 0 f(x) dx = 0 is a linear subspace of C[0; 1]. Proof. 1) It is obvious that W is a subset of C[0; 1], i.e., W ⊂ C[0; 1]. − R2) W is a non-emptyR subset. For example, f0(t) = 2t 1 belongs to W , since 1 1 − t2 − j1 0 f0(t) dt = 0 2t 1 dt = (2 2 t) 0 = 0. 3) For every f; g 2 W , we have Z Z 1 1 (f + g)(t) dt = f(t) + g(t) dt 0 Z0 Z 1 1 = f(t) dt + g(t) dt (by the linearity of integrals) 0 0 = 0 + 0 = 0: Thus f + g 2 W . 4) For every f 2 W and λ 2 C, we have Z Z 1 1 (λf)(t) dt = λf(t) dt 0 0Z 1 = λ f(t) dt (by the linearity of integrals) 0 = 0: Thus λf 2 W . Hence W is a vector subspace of C[0; 1]. 5 2 1 Example 1.8. Let ` be the set of all complex sequences x = (xn)n=1 which are square summable, i.e. satisfy X1 2 jxnj < 1; n=1 we write X1 2 2 ` = f(x1; : : : ; xn;:::): xi 2 C 8i and jxnj < 1g: n=1 2 N N Show that ` is a vector subspace of C . Recall that C = f(x1; : : : ; xn;:::): xi 2 C 8ig with x + y = (x1 + y1; : : : ; xn + yn;:::) and λx = (λx1; : : : ; λxn;:::). Proof. 1). It is obvious that `2 is a subset of CN. P 1 1 1 2 2 1 1 2). The sequence x = (1; 2 ; 3 ;:::; n ;:::) ` since the series n=1 n2 converges. Thus `2 is non-empty. 1 1 2 2 3). We have to prove that, for any x = (xn)n=1; y = (yn)n=1 ` , x + y = 2 P(x1 + y1; : : : ; xn + yn;:::) belongs to ` . Thus we have to show that the series 1 j j2 n=1 xn + yn converges. We will prove this in Theorem 1.11. 1 2 2 2 C 4). For any x = (xn)n=1 ` and λ we have λx = (λx1; : : : ; λxn;:::). Note that X1 X1 2 2 2 jλxnj = jλj jxnj n=1 n=1 X1 2 2 = jλj jxnj < 1; n=1 so λx 2 `2. Hence `2 is a vector subspace of CN, and `2 is a complex vector space. The Cauchy-Schwarz Inequality 1.9. Xn Xn Xn 2 1 2 1 ≤ 2 2 akbk ( ak) ( bk) k=1 k=1 k=1 for all ak; bk 2 R; ak; bk ≥ 0; k = 1; 2; : : : ; n; n 2 N. 6 It follows from ! 2 Xn Xn Xn 1 Xn Xn a b = a2 b2 − (a b − b a )2: k k k k 2 i j i j k=1 k=1 k=1 i=1 j=1 P 2 2 1 Lemma 1.10. For any x; y ` , the series n=1 xnyn converges absolutely and ( ) 1 ( ) 1 X1 X1 2 X1 2 2 2 jxnynj ≤ jxnj jynj : n=1 n=1 n=1 Proof. Using the Cauchy-Schwarz inequality, for every k 2 N, we have Xk Xk jxnynj = jxnjjynj n=1 n=1 ( ) 1 ( ) 1 Xk 2 Xk 2 2 2 ≤ jxnj jynj by the Cauchy-Schwarz inequality n=1 n=1 ( ) 1 ( ) 1 X1 2 X1 2 2 2 ≤ jxnj jynj : n=1 n=1 The latter expression is a finite number independent of k, and so the series X1 jxnynj n=1 converges and ( ) 1 ( ) 1 X1 X1 2 X1 2 2 2 jxnynj ≤ jxnj jynj : n=1 n=1 n=1 Theorem 1.11. For any x; y 2 `2, 0 1 ( ) 1 ( ) 1 2 X1 X1 2 X1 2 2 @ 2 2 A jxn + ynj ≤ jxnj + jynj n=1 n=1 n=1 and x + y 2 `2. 7 Proof. For every n 2 N, 2 jxn + ynj = (xn + yn)(xn + yn) = xnxn + ynxn + xnyn + ynyn) 2 2 = jxnj + xnyn + xnyn + jynj 2 2 ≤ jxnj + 2jxnynj + jynj : Hence, for every k 2 N, Xk Xk 2 2 2 jxn + ynj ≤ (jxnj + 2jxnynj + jynj ) n=1 n=1 Xk Xk Xk 2 2 = jxnj + 2 jxnynj + jynj n=1 n=1 n=1 X1 X1 X1 2 2 ≤ jxnj + 2 jxnynj + jynj n=1 n=1 n=1 ! 1 ! 1 X1 X1 2 X1 2 X1 2 2 2 2 ≤ jxnj + 2 jxnj jynj + jynj 0n=1 n=1 n=11 n=1 ( ) 1 ( ) 1 2 X1 2 X1 2 @ 2 2 A = jxnj + jynj : n=1 n=1 The latter expression is a finite number independent of k, so the series X1 2 jxn + ynj n=1 converges and 0 1 ( ) 1 ( ) 1 2 X1 X1 2 X1 2 2 @ 2 2 A jxn + ynj ≤ jxnj + jynj : n=1 n=1 n=1 The theorem is proved. 8 2 Normed Spaces Suppose we have a vector space V , the generalisation of Rn, and we want to know about size of vectors x 2 V , and about the distance between two vectors x; y 2 V . Suppose we would also like to estimate the distance from a vector x 2 V to a vector subspace W ⊂ V . To deal with this kind of problem we define a norm on V .
Details
-
File Typepdf
-
Upload Time-
-
Content LanguagesEnglish
-
Upload UserAnonymous/Not logged-in
-
File Pages105 Page
-
File Size-