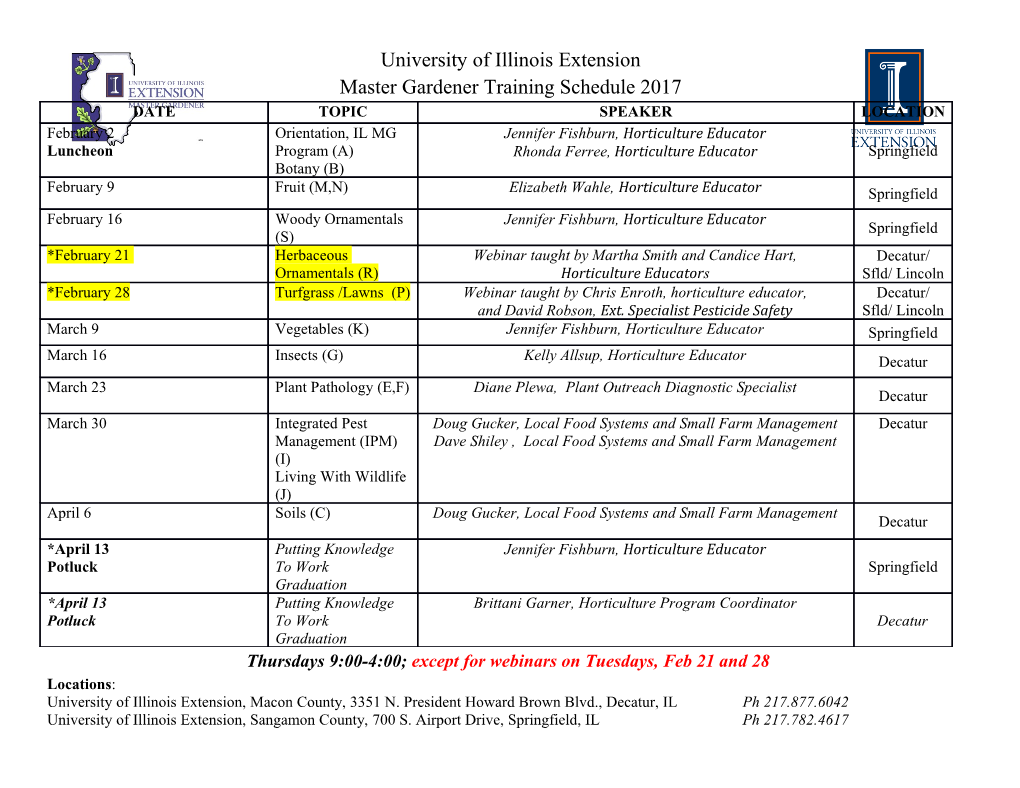
bioRxiv preprint doi: https://doi.org/10.1101/2020.06.01.107391; this version posted June 2, 2020. The copyright holder for this preprint (which was not certified by peer review) is the author/funder, who has granted bioRxiv a license to display the preprint in perpetuity. It is made available under aCC-BY 4.0 International license. 1 Using generalised dissimilarity modelling and targeted field surveys 2 to gap-fill an ecosystem surveillance network 3 4 Greg R. Guerin1,2*, Kristen J. Williams3, Emrys Leitch1,2, Andrew J. Lowe1, Ben Sparrow1,2 5 6 1School of Biological Science, The University of Adelaide, Adelaide, South Australia 5005, 7 Australia 8 2Terrestrial Ecosystem Research Network 9 3CSIRO Land and Water, Canberra, Australian Capital Territory 2601, Australia 10 11 *Email: [email protected] 12 1 bioRxiv preprint doi: https://doi.org/10.1101/2020.06.01.107391; this version posted June 2, 2020. The copyright holder for this preprint (which was not certified by peer review) is the author/funder, who has granted bioRxiv a license to display the preprint in perpetuity. It is made available under aCC-BY 4.0 International license. 13 Abstract 14 1. When considering which sites or land parcels complement existing conservation or 15 monitoring networks, there are many strategies for optimising ecological coverage in the 16 absence of ground observations. However, such optimisation is often implemented 17 theoretically in conservation prioritisation frameworks and real-world implementation is 18 rarely assessed, particularly for networks of monitoring sites. 19 2. We assessed the performance of adding new survey sites informed by predictive modelling 20 in gap-filling the ecological coverage of the Terrestrial Ecosystem Research Network’s 21 (TERN) continental network of ecosystem surveillance plots, Ausplots. Using plant cover 22 observations from 531 sites, we constructed a generalised dissimilarity model (GDM) in 23 which species composition was predicted by environmental parameters. We combined 24 predicted nearest-neighbour ecological distances for locations across Australia with practical 25 considerations to select regions for gap-filling surveys of 181 new plots across 18 trips. We 26 tracked the drop in mean nearest-neighbour distances in GDM space, and increases in the 27 actual sampling of ecological space through cumulative multivariate dispersion. 28 3. GDM explained 34% of deviance in species compositional turnover and retained 29 geographic distance, soil P, aridity, actual evapotranspiration and rainfall seasonality among 30 17 significant predictors. 31 4. Key bioregions highlighted as gaps included Cape York Peninsula, Brigalow Belt South, 32 South Eastern Queensland, Gascoyne and Dampierland. 33 5. We targeted identified gap regions for surveys in addition to opportunistic or project-based 34 gap-filling over two years. Approximately 20% of the land area of Australia received 35 increased servicing of biological representation, corresponding to a drop in mean nearest- 36 neighbour ecological distances from 0.38 to 0.33 in units of compositional dissimilarity. The 37 gain in sampled ecological space was 172% that from the previous 181 plots. Notable gaps 2 bioRxiv preprint doi: https://doi.org/10.1101/2020.06.01.107391; this version posted June 2, 2020. The copyright holder for this preprint (which was not certified by peer review) is the author/funder, who has granted bioRxiv a license to display the preprint in perpetuity. It is made available under aCC-BY 4.0 International license. 38 were filled in northern and south-east Queensland, north-east New South Wales and northern 39 Western Australia. 40 6. Biological scaling of environmental variables through GDM supports practical sampling 41 decisions for ecosystem monitoring networks. Optimising putative survey locations via 42 ecological distance to a nearest neighbour rather than to all existing sites is useful when the 43 aim is to increase representation of habitats rather than sampling evenness per se. Iterations 44 between modelled gaps and field campaigns provide a pragmatic compromise between 45 theoretical optima and real-world decision-making. 46 3 bioRxiv preprint doi: https://doi.org/10.1101/2020.06.01.107391; this version posted June 2, 2020. The copyright holder for this preprint (which was not certified by peer review) is the author/funder, who has granted bioRxiv a license to display the preprint in perpetuity. It is made available under aCC-BY 4.0 International license. 47 Introduction 48 Conservation planners have long grappled with the problem of how to maximise ecological 49 representation in the absence of perfect information on biodiversity (Albuquerque & Beier 50 2018). For example, finite budgets mean the acquisition of incompletely surveyed land 51 parcels to complement reserve systems must be prioritised. Indeed, much of the literature 52 concerning spatial representation deals with conservation planning (Ferrier 2002; Williams et 53 al. 2006; Arponen et al. 2008; Pennifold et al. 2017), and, in particular, reserve selection 54 (Faith & Walker 1996; Araújo et al. 2001; Engelbrecht et al. 2016). 55 Fewer studies have addressed optimising representation for targeted biological 56 surveys (Funk et al. 2005; Hortal & Lobo 2005; Ware et al. 2018) or the design of monitoring 57 networks (Stohlgren et al. 2011; Rose et al. 2016). Networks of ecosystem observation plots 58 have traditionally been designed to maximise spatial or environmental coverage using 59 stratification (Goring et al. 2016; Guerin et al. 2020). Even so, the problems of 60 complementary reserve selection and gap-filling monitoring networks are, in essence, the 61 same (Hopkins & Nunn 2010); both seek to maximise ecological coverage in the absence of 62 fine-scale biodiversity survey data. For this reason, biodiversity surrogates are often 63 employed to estimate and compare representativeness (Grantham et al. 2010). 64 While many easily measured indicators of biological pattern could serve as 65 surrogates, spatial information is most commonly employed, due to its relative ease of access 66 and high spatial coverage, and to enable interpolation between sparse data locations 67 (Rodrigues & Brook 2007). For example, environmental layers relating to soil type, climate 68 and land cover are frequently used as surrogates for ecological community composition, as 69 are maps of classified vegetation types or bioregions (Ware et al. 2018). The rationale for 70 environmental layers as surrogates is the assumption that species compositional turnover 71 occurs as a result of environmental heterogeneity, due to differences in realised species 4 bioRxiv preprint doi: https://doi.org/10.1101/2020.06.01.107391; this version posted June 2, 2020. The copyright holder for this preprint (which was not certified by peer review) is the author/funder, who has granted bioRxiv a license to display the preprint in perpetuity. It is made available under aCC-BY 4.0 International license. 72 niches (Araújo et al. 2001). However, while environmental layers represent the broad 73 environmental setting of ecological communities, they do not directly capture other key 74 drivers of finer scale species compositional turnover, such as local disturbance regimes, 75 microclimates, human impacts, and biotic interactions (Araújo et al. 2001; Peres-Neto et al. 76 2012; Goring et al. 2016). The spatial structure of ecological similarity (i.e., the degree to 77 which species are shared between locations in space or time) is well known and is the reason 78 that unstratified, systematic sampling performs reasonably at sampling ecological variation 79 (Peres-Neto et al. 2012; Goring et al. 2016; Guerin et al. 2020). While local (patch level) 80 drivers are opaque at large scales, ideally the purely spatial component of turnover would be 81 included in assessments of surrogates. 82 In terms of selecting additional sites that best complement the ecological pattern of an 83 existing set of sites, a range of optimisation strategies have been proposed that vary in terms 84 of effectiveness and computational efficiency. Stohlgren et al. (2011) used Maxent modelling 85 of the environmental coverage of monitoring site locations to select the most dissimilar 86 putative sampling sites. Another approach is to select new sites that maximise the pairwise 87 distances among all sample sites, so-called ‘Environmental Diversity’, which results in even 88 sampling across environmental space (Faith & Walker 1996; Faith et al. 2004; Albuquerque 89 & Beier 2018). 90 One realisation of environmental surrogate approaches is that ecological turnover is 91 rarely a linear function of environmental turnover. The relationship is often nonstationary, 92 with varying rates of turnover or even abrupt boundaries between compositionally distinct 93 communities or geographic ecotones between structural vegetation types (Ferrier et al. 2007; 94 Gibson et al. 2015; Goring et al. 2016). Generalised dissimilarity modelling (GDM; Ferrier et 95 al 2007) has become the standard in community ecology for deriving biotically-scaled 96 environmental surrogates (ecological environments) where sufficient biological training data 5 bioRxiv preprint doi: https://doi.org/10.1101/2020.06.01.107391; this version posted June 2, 2020. The copyright holder for this preprint (which was not certified by peer review) is the author/funder, who has granted bioRxiv a license to display the preprint in perpetuity. It is made available under aCC-BY 4.0 International
Details
-
File Typepdf
-
Upload Time-
-
Content LanguagesEnglish
-
Upload UserAnonymous/Not logged-in
-
File Pages38 Page
-
File Size-