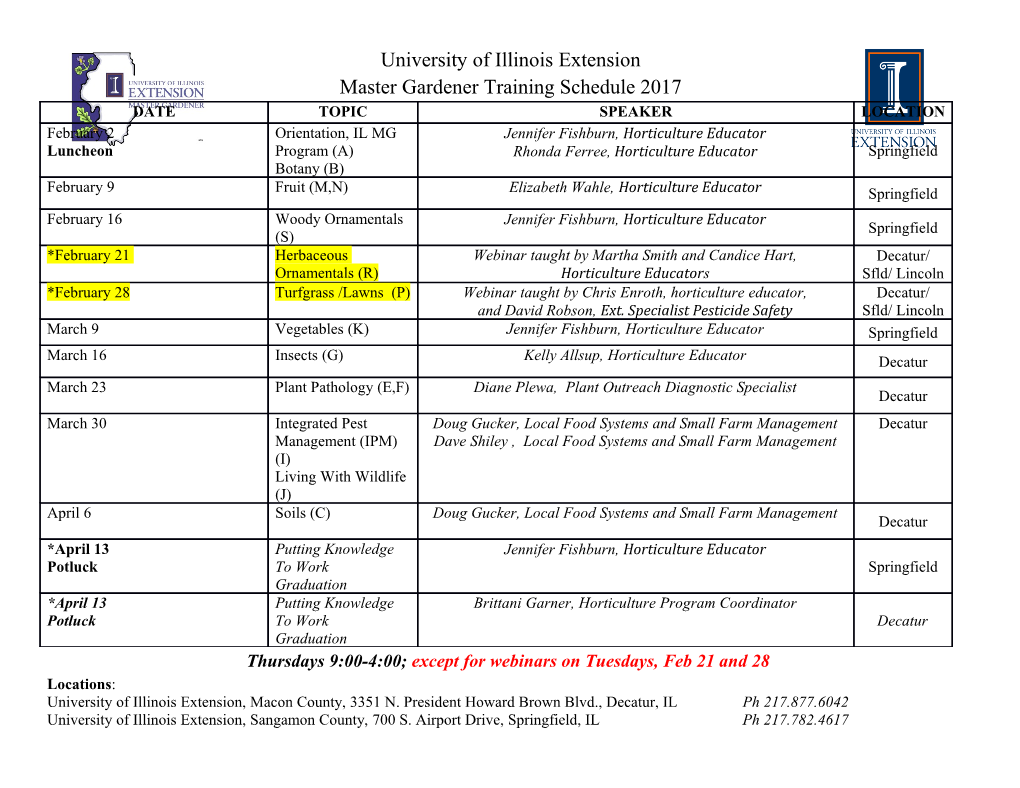
Feedback Mechanisms and constraints on Climate Sensitivity from a Perturbed Physics Ensemble of General Circulation Models Benjamin Mark Sanderson Trinity College A thesis submitted to the Mathematical and Physical Sciences Division for the degree of Doctor of Philosophy in the University of Oxford Trinity Term, 2007 Atmospheric, Oceanic and Planetary Physics, University of Oxford Feedback Mechanisms and constraints on Climate Sensitivity from a Perturbed Physics Ensemble of General Circulation Models Benjamin Mark Sanderson, Trinity College, Oxford Submitted for the degree of Doctor of Philosophy, Trinity Term, 2007 Abstract One of the major uncertainties plaguing predictions of future climate is so-called “structural uncertainty”, which describes the difference between models and the physical systems to which they relate. In General Circulation Models of the climate, the major structural uncertainty lies in finding the most appropriate parameterisa- tions for processes occurring at scales smaller than that of the model itself. Until recently, the computing power required to explicitly simulate thousands of models using various possible parameter configurations has been unattainable. The avail- ability of distributing computing architectures has allowed such an experiment to take place. Two analyses are presented for the analysis of this multi-thousand member “per- turbed physics” GCM ensemble. In the first, a linear analysis is used to identify the dominant physical processes responsible for variation in climate sensitivity across the ensemble. Model simulations are provided by the distributed computing project, climateprediction.net.A principal component analysis of model radiative response reveals two dominant independent feedback processes, each largely controlled by a single parameter change. These parameters are found to account for a large fraction of the variation in equilibrium climate sensitivity within the ensemble. Regression techniques enable a prediction of the real-world strength of these dominant feedback mechanisms using reanalysis data. In the second analysis, a climate model emulator is developed using a feed-forward neural network, trained with the data from climateprediction.net.The emulator is used to simulate a much larger ensemble which explores model parameter space more fully. This emulated ensemble is used to search for models closest to observations over a wide range of equilibrium response to greenhouse gas forcing - thus identifying regions of interest in the parameter space of the model which may be explored in future experiments. The relative discrepancies of these models from observations provide a constraint on climate sensitivity by identifying the sensitivity at which the model discrepancy from observations is minimised. As more observations are added to the error metric, it is found that the discrepancy between ensemble models and observation rapidly exceeds the discrepancy between the models themselves. This result highlights a pos- sible oversight in previous ensemble-based predictions of climate sensitivity, which tend to ignore this systematic component of model error. Acknowledgements The research described in this dissertation would not have been possible without the help, support and patience provided by many individuals. I extend my gratitude to my supervisor, Myles Allen for providing unique insight into the problems contained herein, and without whom the collaborations and opportunities I have enjoyed over the last three years would have been impossible. I would also like to thank my co-supervisor, D´aith´ıStone for his ideas, support and sound advice. Much of this work was made possible with the help of external collaborators. Many, many thanks to Reto Knutti, for his endless support, inspiration and hospitality - both in the office and on the mountains! I would like also to thank the members of the Climate and Global Dynamics group at NCAR for their support and cooperation during my time there, their friendliness and support made my time in Colorado always enjoyable. My great thanks go also to Claudio Piani (and family) for his guidance and true Italian hospitality. Thanks also to the staff at ICTP who accommodated me so well. To all past and present members of the Climate Dynamics group in Oxford, thank you. The regular discussions which I’ve had with so many of you have been invalu- able in forming and developing my ideas. A special thanks to William Ingram for patiently answering all my questions and showing so much interest in my work. I would like also to thank those who provided the data to make this analysis possible, the rest of the climateprediction.net team: Tolu Aina, Carl Christensen, Dave Frame, Nick Faull, Dave Stainforth, Sylvia Knight, Milo Thurston and Hiro Yamazaki and many more. Many thanks also to CEH for their support. Thanks also to my examiners, David Marshall and Mat Collins, for new ideas and a really interesting discussion. Mum, Dad, Charlotte: Thank you for always being there in hard times. And finally, I reserve my greatest thanks for Carly. Your love and guidance pulled me through even when I could not see the end - I am forever grateful. Contents 1 Introduction 2 1.1 ClimateChange.............................. 2 1.2 Uncertainty in predictions of future climate . ...... 4 1.3 ClimateModels .............................. 8 1.3.1 Simplemodelsofclimate. 9 1.3.2 Observational Constraints on Sensitivity . ... 11 1.3.3 GeneralCirculationModels . 15 1.4 EnsemblePredictions. 20 1.4.1 The Coupled Model Intercomparison Project . 20 1.4.2 Perturbed Physics Ensembles . 21 1.4.3 Thesisoutline ........................... 25 2 Ensemble Analysis: Background 27 2.1 PreliminaryResults. .. .. .. 28 2.2 SensitivityAnalyses. .. .. .. 29 2.2.1 Pianietal. ............................ 32 2.2.2 Knuttietal............................. 36 2.2.3 RougierandSexton. 41 2.3 Motivationforfurtherwork . 42 i 2.3.1 PhysicalInterpretation . 42 2.3.2 SystematicModelError . 44 3 Technical Background 46 3.1 PerturbedParameterisations . 48 3.1.1 Large-ScalePrecipitation. 49 3.1.2 Saturated Humidity and Large-Scale Clouds . 52 3.1.3 ConvectionScheme . 56 3.1.4 Sea Ice Albedo Temperature dependence . 59 4 Linear feedback analysis 60 4.1 Introduction................................ 60 4.1.1 Feedbacks and Climate Sensitivity . 60 4.2 Methodology ............................... 64 4.2.1 Regionalfeedbackanalysis . 64 4.2.2 EOFAnalysis ........................... 66 4.2.3 RegressionTechniques . 67 4.2.4 Projection of Observations onto Feedbacks . .. 69 4.3 Results................................... 71 4.3.1 EnsembleMeanResponse . 71 4.3.2 EOFAnalysis ........................... 74 4.3.3 ObservationalProjections . 83 4.3.4 Sub-ensemble analysis . 85 4.4 Sensitivity Distributions . 89 4.4.1 Frequency Distribution of Sensitivity . .. 89 4.4.2 Prediction of likely climate sensitivity . .... 91 4.5 Conclusions ................................ 93 5 Model Optimisation with Neural Networks 96 5.1 Introduction................................ 96 5.2 Methodology ............................... 99 5.2.1 DataPreparation .. .. .. 99 5.2.2 NeuralNetworkArchitecture. .103 5.3 Results...................................106 5.3.1 Verification ............................106 5.3.2 Monte-CarloSimulation . .109 5.4 ParameterDependence . .116 5.5 EmulatorVerification. .120 5.6 Conclusions ................................121 6 Systematic Constraints on Climate Sensitivity 125 6.1 ProbabilityDistributions . 127 6.2 Methodologies...............................128 6.3 Results...................................130 6.3.1 Absolute Likelihood Distribution . 130 6.3.2 Directly Scaled Distributions . 132 6.3.3 RelativeScaling. .133 6.4 Verification ................................135 6.5 Discussion .................................139 7 Summary and Future Work 143 7.1 SummaryofResults ...........................144 7.1.1 Chapter4 .............................144 7.1.2 Chapter5 .............................147 7.1.3 Chapter6 .............................149 1 7.2 Caveats and possible extensions for this thesis . .151 7.2.1 Caveats to the feedback analysis technique . .152 7.2.2 Caveats to the model emulation technique . 153 A Empirical Orthogonal Functions i B Neural Network Architecture v Chapter 1 Introduction “The old men of the valley declare that the climate is changing, and they are very positive that there are now no such winters as they remembered as boys...” —The Valley of Kashmir, Walter Lawrence, 1895 1.1 Climate Change The weather is changing. This is nothing new. On every timescale we experience change. Some of these changes we claim to understand; most creatures on Earth have at least an intuitive sense of how temperatures will respond to the daily and seasonal cycles. At the heart of these cycles lies the mostly predictable variation in the flux of the Sun’s radiation that reaches the Earth’s surface. Yet these basic patterns of heating provoke a fantastically complex response on the surface of our planet. The difference in heating between tropical and polar regions cause an elaborate sequence of dynamical processes which transport heat polewards. Such transport is complicated by the fact we live on a rotating sphere - the geometry of which causes large scale weather systems to form in the mid-latitudes which themselves produce enormous variability in the weather we experience. The presence of water serves to further complicate things. This molecule, which 2 3 exists in three phases in our atmosphere, allows latent heat
Details
-
File Typepdf
-
Upload Time-
-
Content LanguagesEnglish
-
Upload UserAnonymous/Not logged-in
-
File Pages184 Page
-
File Size-