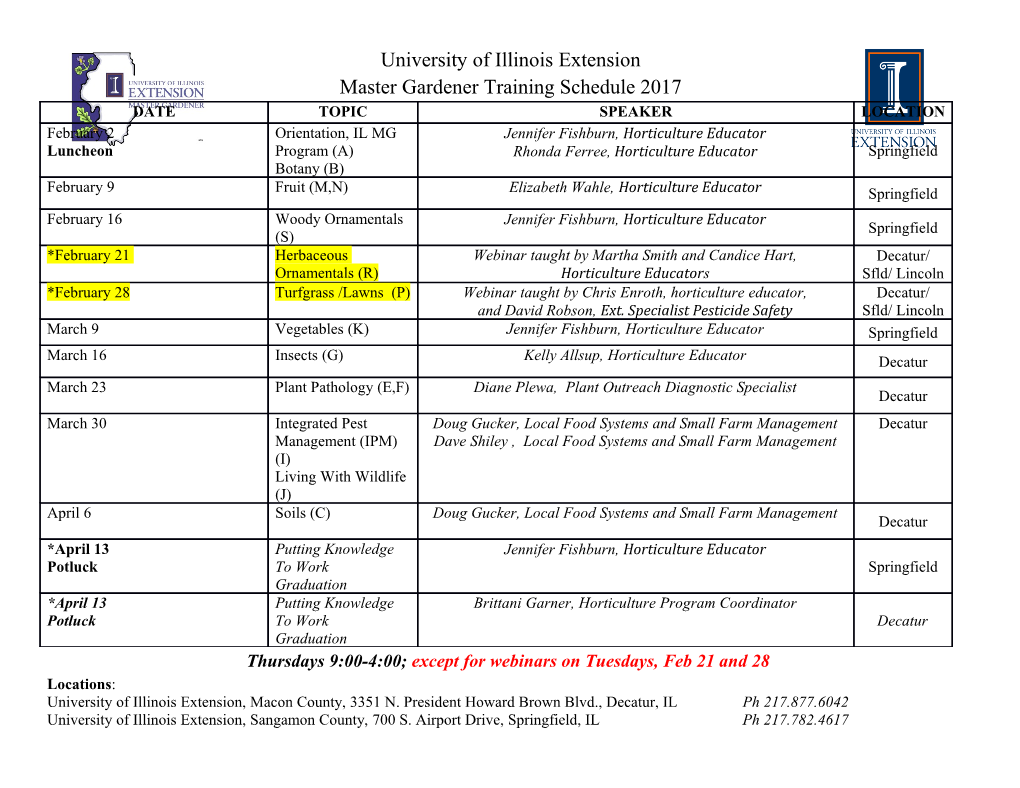
From the Institute of Animal Breeding and Genetics Justus-Liebig-University Gießen Exploring the potential of machine learning methods and selection signature analyses for the estimation of genomic breeding values, the estimation of SNP effects and the identification of possible candidate genes in dairy cattle Dissertation to obtain the doctoral degree (Dr. agr.) in the Faculty of Agricultural Science, Nutritional Science and Environmental Management of Justus-Liebig-University Gießen, Germany presented by Saeid Naderi Darbaghshahi born in Esfahan, Iran Gießen, March 2018 1st Referee: Prof. Dr. Sven König Institute of Animal Breeding and Genetics Justus-Liebig-University Gießen, Germany 2nd Referee: Prof. Dr. Nicolas Gengler Animal Science Unit, Numerical Genetics, Genomics and Modeling Agriculture, Bio-engineering and Chemistry Department University of Liège - Gembloux Agro-Bio Tech, Belgium Table of Contents SUMMARY ........................................................................................................................... 1 1st Chapter General introduction ........................................................................................... 3 From conventional pedigree-based selection towards genomic selection ............................. 4 Effects of genomic selection on rate of genetic gain ............................................................. 5 Factors that affect accuracy of genomic prediction ............................................................... 5 Methods of genomic prediction ............................................................................................. 7 Genomic selection for disease resistance using cow training set ........................................ 10 From genome wide association study (GWAS) to identification of selection signatures ... 10 Cross-population extended haplotype homozygosity (XP-EHH). ....................................... 12 Objectives of the thesis ........................................................................................................ 13 2nd Chapter Random forest estimation of genomic breeding values for disease susceptibility over different disease incidences and genomic architectures in simulated cow calibration groups ………………………………………………………………….......….....….....….......………......21 3rd Chapter Genomic breeding values, SNP effects and gene identification for disease traits in cow training sets …………………………………………………………..………....………….. 47 4th Chapter Assessing signatures of selection through variation in linkage disequilibrium within and between dual-purpose black and white (DSN) and German Holstein cattle populations …...………………………………………………………………………………………………............81 5th Chapter General Discussion ...................................................................................... 118 Preface and Overview ........................................................................................................ 119 Impact of disease incidences in the cow training set on accuracies of GEBV .................. 120 Impact of genetic architecture of traits on accuracies of GEBV ....................................... 121 Impact of the model choice on accuracies of GEBV ......................................................... 123 Assessing predictive ability using receiver operator characteristic (ROC) curves ............ 123 Theoretical expectations and AUCmax ............................................................................... 124 Utilization SNP correlations from random forest for genome wide association studies ... 126 Detection of selection signature ......................................................................................... 127 Signatures of positive selection revealed by FST .............................................................. 128 Holstein and DSN .............................................................................................................. 128 East-DSN and West-DSN .................................................................................................. 129 Sick and healthy Holstein cows ......................................................................................... 130 CONCLUSION .................................................................................................................. 131 LIST OF TABLES 2nd Chapter Table 1: The evaluated scenarios with respect to the no. of markers and QTL, the heritability of the trait, and the level of linkage disequilibrium (LD) .................................................................... 25 Table 2: Parameters of the simulation process .............................................................................. 27 Table 3: Correlation between true and predicted genomic breeding values for S_I (10K SNP, h2 =0.30 and 725 QTL), S_II (10K SNP, h2 =0.30 and 290 QTL) and S_III (10K SNP, h2 =0.10 and 290 QTL) from RF and GBLUP applications (the values in parenthesis show the SD from ten replicates) ........................................................................................................................................ 33 Table 4: The area under the receiving operating characteristic curve (AUROC) for S_I (10K SNP, h2 =0.30 and 725 QTL), S_II (10K SNP, h2 =0.30 and 290 QTL) and S_III (10K SNP, h2 =0.10 and 290 QTL) from RF and GBLUP applications (the values in parenthesis show the SD from ten replicates). ......................................................................................................................... 34 Table 5: Correlation between true and predicted genomic breeding values for S_IV (50K SNP, h2 =0.30 and 725 QTL), S_V (50K SNP, h2 =0.30 and 290 QTL), S_VI (50K SNP, h2 =0.10 and 290 QTL) and S_VII (50K SNP, h2 =0.10, 290 QTL and high LD) from RF and GBLUP applications (the values in parenthesis show the SD from ten replicates) .......................................................... 35 Table 6: The area under the receiving operating characteristic curve (AUROC) for S_IV (50K SNP, h2 =0.30 and 725 QTL), S_V (50K SNP, h2 =0.30 and 290 QTL), S_VI (50K SNP, h2 = 0.10 and 290 QTL) and S_VII (50K SNP, h2 = 0.10, 290 QTL and high LD) from RF and GBLUP applications (the values in parenthesis show the SD from ten replicates). ..................................... 36 Table 7: The calculated r2 between each of ten most effective QTL and the most important SNP located in the same chromosome for scenarios S_I (10K SNP chip, h2 = 0.3 and QTL = 725) and S_IV (50K SNP chip, h2 = 0.3 and QTL = 725) ............................................................................ 38 3rd Chapter Table 1: Overview of health disorders as used in the present study along with their disease incidences (in %) in the total data set (T) and in the dataset of genotyped cows (G) ................. 52 Table 2: SNP associations for laminitis, dermatitis digitalis, clinical mastitis and endometritis with false discovery rate (FDR) ≤ 10% from GWAS, the most important variable from RF (VIM = 1) and annotated genes in 500 Kb up and downstream of the given SNP ................................... 68 4th Chapter Table 1: The regions under positive selection in the DSN population and the annotated potential candidate genes within a window of 250 Kb downstream and upstream of each core SNP .......... 92 Table 2: The regions under positive selection in the Holstein population and the annotated genes in a window of 250 Kb downstream and upstream of each core SNP ........................................... 94 Table 3: The regions under positive selection in the East-DSN sub-population and the annotated genes in a window of 250 Kb downstream and upstream of each core SNP .................................. 97 Table 4: The regions under positive selection in West-DSN sub-population and the annotated genes in a window of 250 Kb downstream and upstream of each core SNP ................................ 100 Table 5: The regions under positive selection in the healthy Holstein sub-population and the annotated genes in a window of 250 Kb downstream and upstream of each core SNP ............... 103 Table 6: The regions under positive selection in the sick Holstein sub-population and the annotated genes in a window of 250 Kb downstream and upstream of each core SNP ............... 107 LIST OF FIGURES 2nd Chapter Figure 1: Strategy for the creation of training and testing sets ...................................................... 30 Figure 2: The relative importance of each 10K (a) and 50K (b) SNP, and positions and percentage of phenotypic variance related to ten top QTL (black circles) along 29 chromosomes ......................................................................................................................................................... 42 3rd Chapter Figure 1: Correlation between de-regressed proofs and genomic breeding values (rGBV) and the corrected prediction accuracy using the ―Wellmann-equation‖ (rGBV-cor) for claw disorders (A), for clinical mastitis (B) and for infertility (C) from random forest (RF) and genomic BLUP (GBLUP) applications ...................................................................................................................................... 60 Figure 2: Prediction accuracy (rGBV-PCP) for claw disorders (A), for clinical mastitis (B) and for infertility (C) from random forest (RF), genomic BLUP (GBLUP) and single step genomic BLUP (ssGBLUP) applications .................................................................................................................
Details
-
File Typepdf
-
Upload Time-
-
Content LanguagesEnglish
-
Upload UserAnonymous/Not logged-in
-
File Pages143 Page
-
File Size-