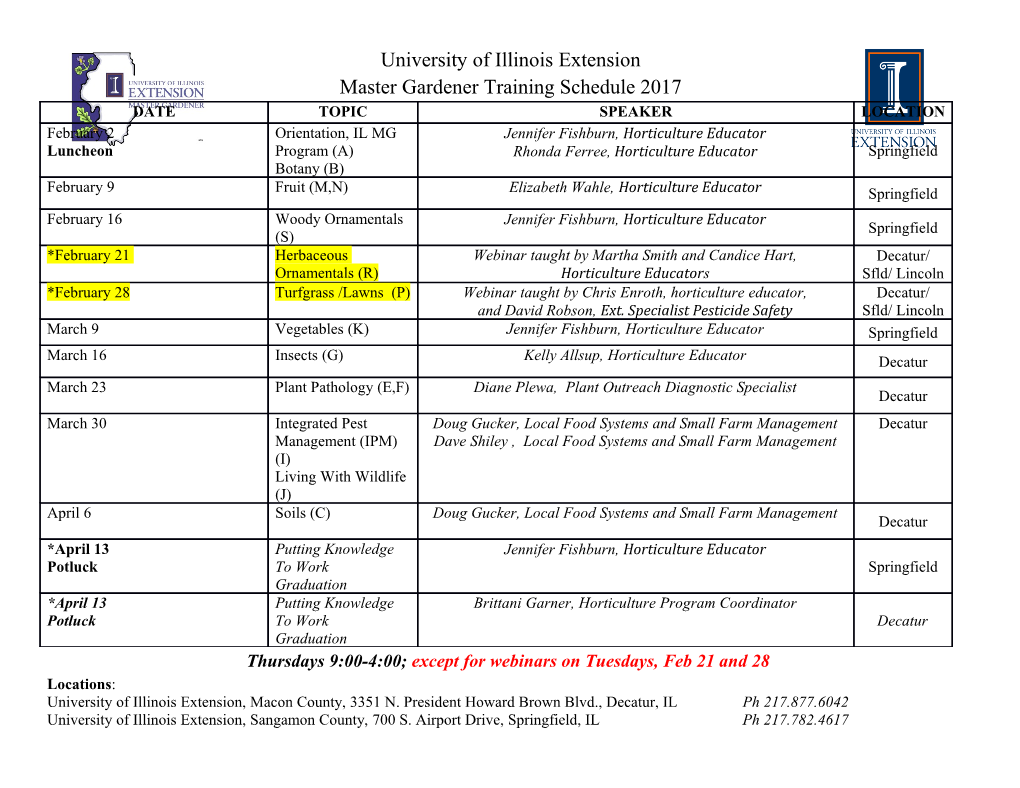
Probability Cheatsheet v2.0 Thinking Conditionally Law of Total Probability (LOTP) Let B1;B2;B3; :::Bn be a partition of the sample space (i.e., they are Compiled by William Chen (http://wzchen.com) and Joe Blitzstein, Independence disjoint and their union is the entire sample space). with contributions from Sebastian Chiu, Yuan Jiang, Yuqi Hou, and Independent Events A and B are independent if knowing whether P (A) = P (AjB )P (B ) + P (AjB )P (B ) + ··· + P (AjB )P (B ) Jessy Hwang. Material based on Joe Blitzstein's (@stat110) lectures 1 1 2 2 n n A occurred gives no information about whether B occurred. More (http://stat110.net) and Blitzstein/Hwang's Introduction to P (A) = P (A \ B1) + P (A \ B2) + ··· + P (A \ Bn) formally, A and B (which have nonzero probability) are independent if Probability textbook (http://bit.ly/introprobability). Licensed and only if one of the following equivalent statements holds: For LOTP with extra conditioning, just add in another event C! under CC BY-NC-SA 4.0. Please share comments, suggestions, and errors at http://github.com/wzchen/probability_cheatsheet. P (A \ B) = P (A)P (B) P (AjC) = P (AjB1;C)P (B1jC) + ··· + P (AjBn;C)P (BnjC) P (AjB) = P (A) P (AjC) = P (A \ B1jC) + P (A \ B2jC) + ··· + P (A \ BnjC) P (BjA) = P (B) Last Updated September 4, 2015 Special case of LOTP with B and Bc as partition: Conditional Independence A and B are conditionally independent P (A) = P (AjB)P (B) + P (AjBc)P (Bc) given C if P (A \ BjC) = P (AjC)P (BjC). Conditional independence Counting does not imply independence, and independence does not imply P (A) = P (A \ B) + P (A \ Bc) conditional independence. Multiplication Rule Unions, Intersections, and Complements Bayes' Rule De Morgan's Laws A useful identity that can make calculating Bayes' Rule, and with extra conditioning (just add in C!) C probabilities of unions easier by relating them to intersections, and cake V vice versa. Analogous results hold with more than two sets. P (BjA)P (A) C waffl S e c c c P (AjB) = cake (A [ B) = A \ B P (B) cake V c c c (A \ B) = A [ B P (BjA; C)P (AjC) waffl wa e P (AjB; C) = ffl C S e P (BjC) V cake Joint, Marginal, and Conditional S We can also write wa ffle Joint Probability P (A \ B) or P (A; B) { Probability of A and B. P (A; B; C) P (B; CjA)P (A) P (AjB; C) = = Let's say we have a compound experiment (an experiment with Marginal (Unconditional) Probability P (A) { Probability of A. P (B; C) P (B; C) multiple components). If the 1st component has n1 possible outcomes, Conditional Probability P (AjB) = P (A; B)=P (B) { Probability of the 2nd component has n2 possible outcomes, . , and the rth A, given that B occurred. Odds Form of Bayes' Rule component has nr possible outcomes, then overall there are Conditional Probability is Probability P (AjB) is a probability P (AjB) P (BjA) P (A) n1n2 : : : nr possibilities for the whole experiment. = function for any fixed B. Any theorem that holds for probability also P (AcjB) P (BjAc) P (Ac) holds for conditional probability. Sampling Table The posterior odds of A are the likelihood ratio times the prior odds. Probability of an Intersection or Union Intersections via Conditioning Random Variables and their Distributions P (A; B) = P (A)P (BjA) PMF, CDF, and Independence P (A; B; C) = P (A)P (BjA)P (CjA; B) Probability Mass Function (PMF) Gives the probability that a Unions via Inclusion-Exclusion 2 8 discrete random variable takes on the value x. 5 P (A [ B) = P (A) + P (B) − P (A \ B) 7 9 pX (x) = P (X = x) 1 4 P (A [ B [ C) = P (A) + P (B) + P (C) 3 6 − P (A \ B) − P (A \ C) − P (B \ C) The sampling table gives the number of possible samples of size k out + P (A \ B \ C): of a population of size n, under various assumptions about how the 1.0 sample is collected. Simpson's Paradox 0.8 Order Matters Not Matter 0.6 heart k n + k − 1 pmf With Replacement n ● k 0.4 n! n ● ● Without Replacement (n − k)! k 0.2 ● ● band-aid 0.0 Naive Definition of Probability 0 1 2 3 4 If all outcomes are equally likely, the probability of an event A Dr. Hibbert Dr. Nick x It is possible to have happening is: The PMF satisfies c c c c P (A j B; C) < P (A j B ;C) and P (A j B; C ) < P (A j B ;C ) X number of outcomes favorable to A pX (x) ≥ 0 and pX (x) = 1 Pnaive(A) = c number of outcomes yet also P (A j B) > P (A j B ): x Cumulative Distribution Function (CDF) Gives the probability Indicator Random Variables LOTUS that a random variable is less than or equal to x. Indicator Random Variable is a random variable that takes on the Expected value of a function of an r.v. The expected value of X FX (x) = P (X ≤ x) value 1 or 0. It is always an indicator of some event: if the event is defined this way: occurs, the indicator is 1; otherwise it is 0. They are useful for many problems about counting how many events of some kind occur. Write X E(X) = xP (X = x) (for discrete X) ( x ● 1 if A occurs, 1.0 IA = ● ● 0 if A does not occur. Z 1 E(X) = xf(x)dx (for continuous X) 0.8 2 −∞ ● ● Note that IA = IA;IAIB = IA\B ; and IA[B = IA + IB − IAIB . The Law of the Unconscious Statistician (LOTUS) states that 0.6 Distribution IA ∼ Bern(p) where p = P (A). you can find the expected value of a function of a random variable, cdf Fundamental Bridge The expectation of the indicator for event A is g(X), in a similar way, by replacing the x in front of the PMF/PDF by 0.4 ● ● g(x) but still working with the PMF/PDF of X: the probability of event A: E(IA) = P (A). 0.2 X E(g(X)) = g(x)P (X = x) (for discrete X) ● ● Variance and Standard Deviation ● x 0.0 Var(X) = E (X − E(X))2 = E(X2) − (E(X))2 0 1 2 3 4 Z 1 q E(g(X)) = g(x)f(x)dx (for continuous X) x SD(X) = Var(X) −∞ The CDF is an increasing, right-continuous function with Continuous RVs, LOTUS, UoU What's a function of a random variable? A function of a random variable is also a random variable. For example, if X is the number of F (x) ! 0 as x ! −∞ and F (x) ! 1 as x ! 1 X X bikes you see in an hour, then g(X) = 2X is the number of bike wheels Independence Intuitively, two random variables are independent if X X(X−1) Continuous Random Variables (CRVs) you see in that hour and h(X) = 2 = 2 is the number of knowing the value of one gives no information about the other. pairs of bikes such that you see both of those bikes in that hour. Discrete r.v.s X and Y are independent if for all values of x and y What's the probability that a CRV is in an interval? Take the difference in CDF values (or use the PDF as described later). What's the point? You don't need to know the PMF/PDF of g(X) P (X = x; Y = y) = P (X = x)P (Y = y) to find its expected value. All you need is the PMF/PDF of X. P (a ≤ X ≤ b) = P (X ≤ b) − P (X ≤ a) = F (b) − F (a) Expected Value and Indicators X X For X ∼ N (µ, σ2), this becomes Universality of Uniform (UoU) Expected Value and Linearity b − µ a − µ When you plug any CRV into its own CDF, you get a Uniform(0,1) P (a ≤ X ≤ b) = Φ − Φ random variable. When you plug a Uniform(0,1) r.v. into an inverse Expected Value (a.k.a. mean, expectation, or average) is a weighted σ σ CDF, you get an r.v. with that CDF. For example, let's say that a average of the possible outcomes of our random variable. random variable X has CDF Mathematically, if x1; x2; x3;::: are all of the distinct possible values What is the Probability Density Function (PDF)? The PDF f that X can take, the expected value of X is is the derivative of the CDF F . −x F (x) = 1 − e ; for x > 0 P E(X) = xiP (X = xi) F 0(x) = f(x) i By UoU, if we plug X into this function then we get a uniformly distributed random variable. X Y X + Y A PDF is nonnegative and integrates to 1. By the fundamental theorem of calculus, to get from PDF back to CDF we can integrate: 3 4 7 F (X) = 1 − e−X ∼ Unif(0; 1) 2 2 4 Z x 6 8 14 F (x) = f(t)dt Similarly, if U ∼ Unif(0; 1) then F −1(U) has CDF F . The key point is 10 23 33 −∞ 1 –3 –2 that for any continuous random variable X, we can transform it into a Uniform random variable and back by using its CDF.
Details
-
File Typepdf
-
Upload Time-
-
Content LanguagesEnglish
-
Upload UserAnonymous/Not logged-in
-
File Pages10 Page
-
File Size-