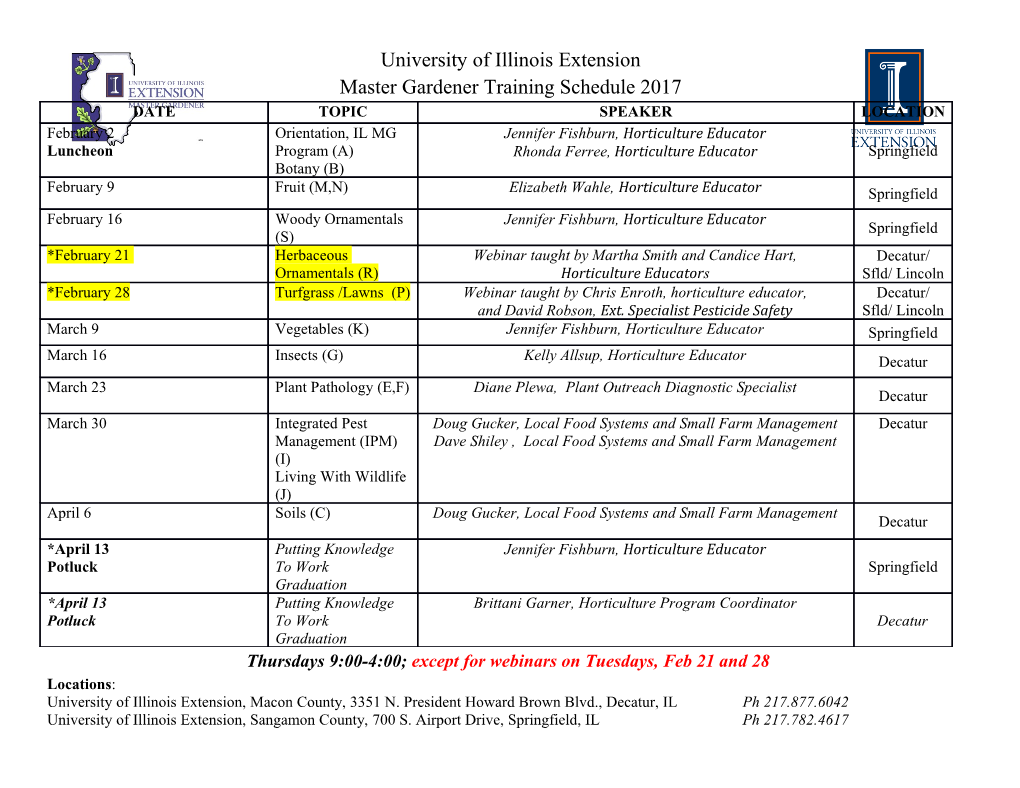
Outline Lecture 1: Parallel Computer Architecture Concepts n Parallel computer, multiprocessor, multicomputer Parallel Computer n SIMD vs. MIMD execution Architecture Concepts n Shared memory vs. Distributed memory architecture n Interconnection networks TDDE35 Lecture 1 n Parallel architecture design concepts l Instruction-level parallelism Christoph Kessler l Hardware multithreading PELAB / IDA l Multi-core and many-core Linköping university Sweden l Accelerators and heterogeneous systems l Clusters n Implications for programming and algorithm design 2019 2 Traditional Use of Parallel Computing: High Performance Computing Large-Scale HPC Applications Application Areas (Selection) n High Performance Computing (HPC) n Computational Fluid Dynamics n Weather Forecasting and Climate Simulation l E.g. climate simulations, particle physics, proteine docking, … n Aerodynamics / Air Flow Simulations and Optimization l Much computational work n Structural Engineering (in FLOPs, floatingpoint operations) n Fuel-Efficient Aircraft Design l Often, large data sets n Molecular Modelling n Material Science n Computational Chemistry n Single-CPU computers and even today’s n Battery Simulation and Optimization multicore processors cannot provide such n Galaxy Simulations massive computation power n Earthquake Engineering, Oil Reservoir Simulation n Flood Prediction n Aggregate LOTS of computers à Clusters n Bioinformatics (DNA Pattern Matching, Proteine Docking) n Fluid / Structural Interaction l Need scalable parallel algorithms n Blood Flow Simulation l Need exploit multiple levels of parallelism n fRMI Image Analysis n ... l Cost of communication, memory access 3 NSC Tetralith 4 w ww.e-science.se Another Classical Use of Parallel Computing: Example: Weather Forecast (very simplified…) Parallel Embedded Computing • 3D Space discretization (cells) • Air pressure • Time discretization (steps) • Temperature n High-performance embedded computing • Start from current observations • Humidity (sent from weather stations etc.) • Sun radiation l E.g. on-board realtime image/video processing, gaming, … cell • Wind direction • Simulation step by evaluating l Much computational work weather model equations • Wind velocity (often fixed point operations) • … l Often, in energy-constrained mobile devices E.g., cell size 1km3 and 10min time discretization à 10-day simulation: 1015 FLOPs n Sequential programs on single-core computers SMHI: 4 forecasts per day, cannot provide sufficient computation power 50 variants (simulations) per forecast at a reasonable power budget n Use many small cores at low frequency l Need scalable parallel algorithms l Cost of communication, memory access l Energy cost (Power x Time) https://www.smhi.se/kunskapsbanken/meteorologi/sa-gor-smhi-en-vaderprognos-1.4662 5 6 NSC Tetralith 1 More Recent Use of Parallel Computing: HPC vs Big-Data Computing Big-Data Analytics Applications n Big Data Analytics n Both need parallel computing n Same kind of hardware – Clusters of (multicore) servers l Data access intensive (disk I/O, memory accesses) n Same OS family (Linux) 4Typically, very large data sets (GB … TB … PB … EB …) n Different programming models, languages, and tools l Also some computational work for combining/aggregating data l E.g. data center applications, business analytics, click stream HPC application Big-Data application analysis, scientific data analysis, machine learning, … HPC prog. languages: Big-Data prog. languages: l Soft real-time requirements on interactive querys Fortran, C/C++ (Python) Java, Scala, Python, … Par. programming models: Par. programming models: n Single-CPU and multicore processors cannot MPI, OpenMP, … MapReduce, Spark, … provide such massive computation power and I/O bandwidth+capacity Scientific computing Big-data storage/access: libraries: BLAS, … HDFS, … n Aggregate LOTS of computers à Clusters OS: Linux OS: Linux l Need scalable parallel algorithms HW: Cluster HW: Cluster l Need to exploit multiple levels of parallelism l Fault tolerance à Let us start with the common basis: Parallel computer architecture 7 NSC Tetralith 8 Parallel Computer Parallel Computer Architecture Concepts Classification of parallel computer architectures: n by control structure n by memory organization l in particular, Distributed memory vs. Shared memory n by interconnection network topology 9 10 Classification by Control Structure Classification by Memory Organization op … vop e.g. (traditional) HPC cluster e.g. multiprocessor (SMP) or computer with a standard multicore CPU Most common today in HPC and Data centers: Hybrid Memory System • Cluster (distributed memory) op op op op 1 2 3 4 of hundreds, thousands of shared-memory servers each containing one or several multi-core CPUs 11 12 NSCNSC Triolith Tetralith 2 Hybrid (Distributed + Shared) Memory Interconnection Networks (1) n Network = physical interconnection medium (wires, switches) + communication protocol (a) connecting cluster nodes with each other (DMS) (b) connecting processors with memory modules (SMS) Classification M M n Direct / static interconnection networks l connecting nodes directly to each other P R l Hardware routers (communication coprocessors) can be used to offload processors from most communication work n Switched / dynamic interconnection networks 13 SC Tetralith 14 Interconnection Networks (2): P Interconnection Networks (3): P Simple Topologies P Fat-Tree Network P fully connected P P n Tree network extended for higher bandwidth (more switches, more links) closer to the root l avoids bandwidth bottleneck n Example: Infiniband network (www.mellanox.com) 15 16 Instruction Level Parallelism (1): More about Interconnection Networks Pipelined Execution Units n Hypercube, Crossbar, Butterfly, Hybrid networks… à TDDC78 n Switching and routing algorithms n Discussion of interconnection network properties l Cost (#switches, #lines) l Scalability (asymptotically, cost grows not much faster than #nodes) l Node degree l Longest path (à latency) l Accumulated bandwidth l Fault tolerance (worst-case impact of node or switch failure) l … 17 18 3 SIMD computing Instruction-Level Parallelism (2): e.g., vector supercomputers VLIW and Superscalar with Pipelined Vector Units Cray (1970s, 1980s), Fujitsu, … n Multiple functional units in parallel n 2 main paradigms: l VLIW (very large instruction word) architecture ^ 4Parallelism is explicit, progr./compiler-managed (hard) l Superscalar architecture à 4Sequential instruction stream 4Hardware-managed dispatch 4power + area overhead n ILP in applications is limited l typ. < 3...4 instructions can be issued simultaneously l Due to control and data dependences in applications 19 n Solution: Multithread the 20application and the processor Hardware Multithreading SIMD Instructions ”vector register” n “Single Instruction stream, Multiple Data streams” l single thread of control flow op SIMD unit l restricted form of data parallelism 4apply the same primitive operation (a single instruction) in parallel to multiple data elements stored contiguously l SIMD units use long “vector registers” 4each holding multiple data elements n Common today E.g., l data MMX, SSE, SSE2, SSE3,… dependence l Altivec, VMX, SPU, … n Performance boost for operations on shorter data types n Area- and energy-efficient n Code to be rewritten (SIMDized) by programmer or compiler n Does not help (much) for memory bandwidth P P 21 P P 22 The Memory Wall Moore’s Law (since 1965) n Performance gap CPU – Memory Exponential increase in transistor density n Memory hierarchy n Increasing cache sizes shows diminishing returns l Costs power and chip area 4 GPUs spend the area instead on many simple cores with little memory l Relies on good data locality in the application n What if there is no / little data locality? l Irregular applications, e.g. sorting, searching, optimization... n Solution: Spread out / overlap memory access delay l Programmer/Compiler: Prefetching, on-chip pipelining, SW-managed on-chip buffers l Generally: Hardware multithreading, again! Data from Kunle Olukotun, Lance Hammond, Herb Sutter, 23 24Burton Smith, Chris Batten, and Krste Asanoviç 4 The Power Issue Moore’s Law vs. Clock Frequency n Power = Static (leakage) power + Dynamic (switching) power n Dynamic power ~ Voltage2 * Clock frequency where Clock frequency approx. ~ voltage • #Transistors / mm2 still 3 growing exponentially à Dynamic power ~ Frequency according to Moore’s Law n Total power ~ #processors ~3GHz • Clock speed flattening out 2003 More transistors + Limited frequency Þ More cores 25 26 Solution for CPU Design: Multicore + Multithreading Main features of a multicore system n There are multiple computational cores on the same chip. n Single-thread performance does not improve any more since ca. 2003 l Homogeneous multicore (same core type) l ILP wall l Heterogeneous multicore (different core types) l Memory wall n The cores might have (small) private on-chip memory l Power wall (end of “Dennard Scaling”) modules and/or access to on-chip memory shared by several cores. n but thanks to Moore’s Law we could still put more cores on a n The cores have access to a common off-chip main memory chip n There is a way by which these cores communicate with each l And hardware-multithread the cores to hide memory other and/or with the environment. latency l All major chip manufacturers produce multicore CPUs today 27 28 Standard CPU Multicore Designs Some early dual-core CPUs (2004/2005) n Standard desktop/server CPUs have a few cores with shared off-chip main memory
Details
-
File Typepdf
-
Upload Time-
-
Content LanguagesEnglish
-
Upload UserAnonymous/Not logged-in
-
File Pages10 Page
-
File Size-