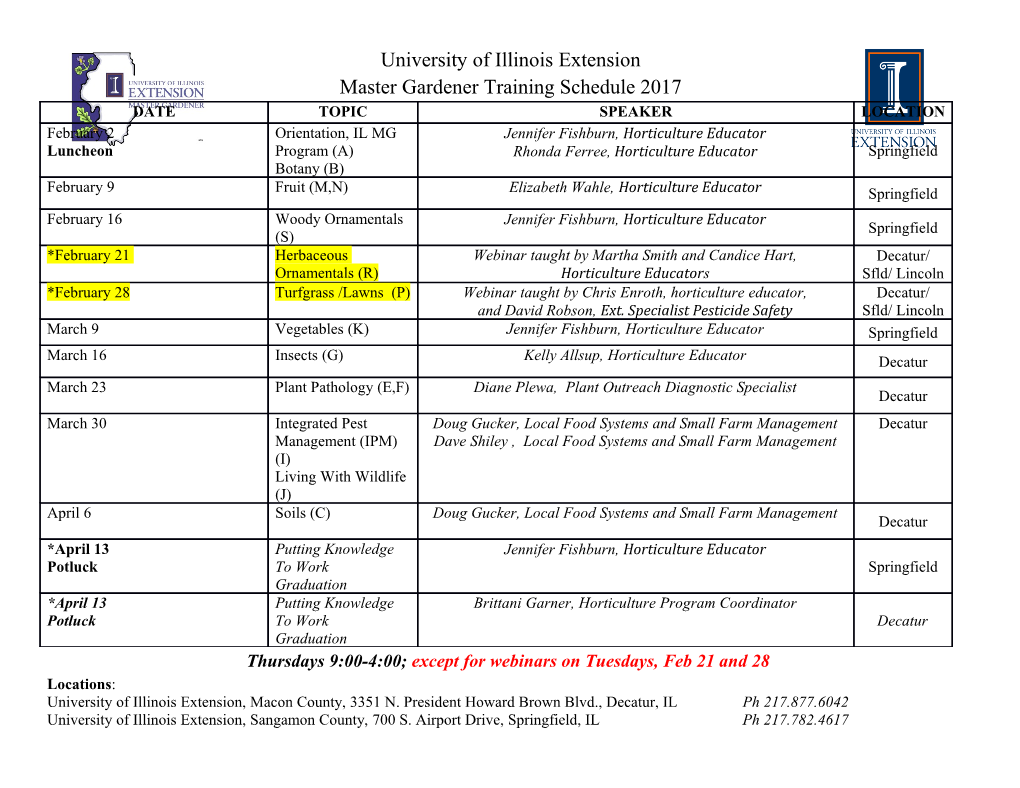
62 1 Journal of Molecular B B Misra et al. Approaches and tools in 62:1 R21–R45 Endocrinology integrated omics REVIEW Integrated omics: tools, advances and future approaches Biswapriya B Misra1, Carl Langefeld1,2, Michael Olivier1 and Laura A Cox1,3 1Center for Precision Medicine, Section on Molecular Medicine, Department of Internal Medicine, Wake Forest School of Medicine, Winston-Salem, North California, USA 2Department of Biostatistics, Wake Forest School of Medicine, Winston-Salem, North California, USA 3Southwest National Primate Research Center, Texas Biomedical Research Institute, San Antonio, Texas, USA Correspondence should be addressed to L A Cox: [email protected] Abstract With the rapid adoption of high-throughput omic approaches to analyze biological Key Words samples such as genomics, transcriptomics, proteomics and metabolomics, each f integrated analysis can generate tera- to peta-byte sized data files on a daily basis. These data file f omics sizes, together with differences in nomenclature among these data types, make the f genomics integration of these multi-dimensional omics data into biologically meaningful context f transcriptomics challenging. Variously named as integrated omics, multi-omics, poly-omics, trans-omics, f proteomics pan-omics or shortened to just ‘omics’, the challenges include differences in data f metabolomics cleaning, normalization, biomolecule identification, data dimensionality reduction, f network biological contextualization, statistical validation, data storage and handling, sharing and f statistics data archiving. The ultimate goal is toward the holistic realization of a ‘systems biology’ f Bayesian understanding of the biological question. Commonly used approaches are currently f machine learning limited by the 3 i’s – integration, interpretation and insights. Post integration, these f principal component very large datasets aim to yield unprecedented views of cellular systems at exquisite analysis resolution for transformative insights into processes, events and diseases through f correlation various computational and informatics frameworks. With the continued reduction in f clustering costs and processing time for sample analyses, and increasing types of omics datasets generated such as glycomics, lipidomics, microbiomics and phenomics, an increasing number of scientists in this interdisciplinary domain of bioinformatics face these challenges. We discuss recent approaches, existing tools and potential caveats in the integration of omics datasets for development of standardized analytical pipelines that Journal of Molecular could be adopted by the global omics research community. Endocrinology (2019) 62, R21–R45 Introduction Access to large-scale omics datasets (genomics, tran- integration has created both exciting opportunities scriptomics, proteomics, metabolomics, metagenomics, and immense challenges for biologists, computational phenomics, etc.) has revolutionized biology and led to biologists, biostatisticians and biomathematicians. As an the emergence of systems approaches to advance our example of a comprehensive analysis approach, Yugi understanding of biological processes. With decreasing et al. (2016) proposed a trans-omics concept of dynamic time and cost to generate these datasets, omics data networks that includes the three most commonly used https://jme.bioscientifica.com © 2019 Society for Endocrinology https://doi.org/10.1530/JME-18-0055 Published by Bioscientifica Ltd. Printed in Great Britain Downloaded from Bioscientifica.com at 10/01/2021 05:51:28PM via free access -18-0055 Journal of Molecular B B Misra et al. Approaches and tools in 62:1 R22 Endocrinology integrated omics layers of omics datasets – transcriptomics, proteomics and of readouts from each omics regime independently, (ii) metabolomics and also included newer datasets such as recognizing non-obvious relationships that exist between phosphoproteomics, protein–protein interactions, DNA– omics regimes within their original biological context and protein interactions and allosteric regulation, which (iii) capitalizing on time resolution in omics data, such as can reveal critical components of dynamic biological time course studies, to inform directionality (Buescher & networks when omics data are successfully integrated. Driggers 2016). A recent review provided data integration Using three case studies in datasets from bacteria and strategies for genomics and proteomics datasets (Huang rats, they showed the interplay of the omics layers, and et al. 2017), but did not mention and include approaches, introduced phenome-wide association, pathway-wide which allow integration of metabolomics datasets. association and trans-ome-wide association (Trans-OWAS) Although all individual omics datasets might not studies to connect phenotypes with omics networks that have the four vs associated with integration of ‘big data’, reflect genetic and environmental factors. These multi- i.e., volume, variety, velocity and veracity, they pose layered, multifactorial approaches are computationally similar challenges, especially in studies with large sample challenging and difficult to display and comprehend numbers. In addition, for high-dimensional datasets of visually. Additional data from microRNA/gene, protein/ more than 1000 variables, popularly known as the ‘curse protein, DNA/protein, and protein/RNA interactions of dimensionality’, variances among samples become further increase the complexity. A recent review enlists large and sparse and render cluster analysis uninformative genome-based systems biology tools and applications (Ronan et al. 2016), further posing challenges interpreting available for network analysis, pathway construction, integrated omic datasets. For clarity, we use ‘integrated genome alignments, assemblies, tree viewers and omics’ to denote multi-omics approaches integrating phylogenies, microarray and RNA-Seq viewers, genome three or more omics datasets and include the major omics browsers, visualization tools for comparative genomics, data types, i.e., genomics, transcriptomics, proteomics and tools for building visual prototypes (Pavlopoulos et al. and metabolomics. 2015). Similarly, tools, resources, databases and software for analysis and visualization of proteomics (Oveland et al. 2015) and metabolomics data (Misra & van der Strengths and challenges of individual omics Hooft 2016, Misra et al. 2017, Misra 2018) are reviewed on Genomics and transcriptomics a yearly basis. However, none of these recent publications provide a comprehensive overview of approaches for Genomics and transcriptomics have been applied to various integrating three or more omics datasets. aspects of research and clinical applications ranging from Although the need for, and the importance of, the pharmaceutical industry, diagnostics and therapeutics, integration of omics data has been realized for a broad gene therapy applications, pharmacogenomics and disease range of research areas, including food and nutrition prevention, to developmental biology, evolutionary science (Kato et al. 2011), systems microbiology (Fondi genomics and comparative genomics. Thus, the ability & Liò 2015), analysis of microbiomes (Muller et al. to manage and analyze these types of data has become 2014), genotype–phenotype interactions (Ritchie et al. necessary for a biomedical scientist’s skill set. The surge 2015), systems biology (Mochida & Shinozaki 2011, in advancements of next-generation sequencing (NGS) Fukushima & Kusano 2013), natural product discovery technologies and progress in genomic data analysis (Yang et al. 2011) and disease biology (Pathak & Dave have led to high-throughput data generation for 2014), successful implementation of more than two genomes (single nucleotide polymorphisms (SNPs), copy omics datasets is very rare. Since Gehlenborg et al. number variants (CNVs), loss of heterozygosity variants, (2010) produced a useful comprehensive compendium genomic rearrangements, and rare variants), epigenomes for visualization of omics data for systems biology using (DNA methylation, histone modifications, chromatin data from microarrays, RNA deep sequencing, mass accessibility, transcription factor (TF) binding) and spectrometry (MS), nuclear magnetic resonance (NMR) transcriptomes (gene expression, alternative splicing, long and protein interactions, considerable progress has been non-coding RNAs and small RNAs such as microRNAs) made to develop additional tools and approaches for (Ritchie et al. 2015). Generally speaking, the nucleic integrated omics analysis. Broad experimental challenges acid-based omics approaches for data generation rely on in these integrated omics approaches include, but are five major steps: appropriate sample collection, high- not limited to (i) understanding the statistical behavior quality nucleic acid extraction, library preparation, clonal https://jme.bioscientifica.com © 2019 Society for Endocrinology https://doi.org/10.1530/JME-18-0055 Published by Bioscientifica Ltd. Printed in Great Britain Downloaded from Bioscientifica.com at 10/01/2021 05:51:28PM via free access Journal of Molecular B B Misra et al. Approaches and tools in 62:1 R23 Endocrinology integrated omics amplification, and sequencing (e.g., pyrosequencing, liquid chromatography (LC) approaches, followed by sequencing-by ligation, or sequencing-by synthesis). The MS, peptide and protein identification and quantification specific approach used for each step varies based on the and additional bioinformatics analyses such
Details
-
File Typepdf
-
Upload Time-
-
Content LanguagesEnglish
-
Upload UserAnonymous/Not logged-in
-
File Pages25 Page
-
File Size-