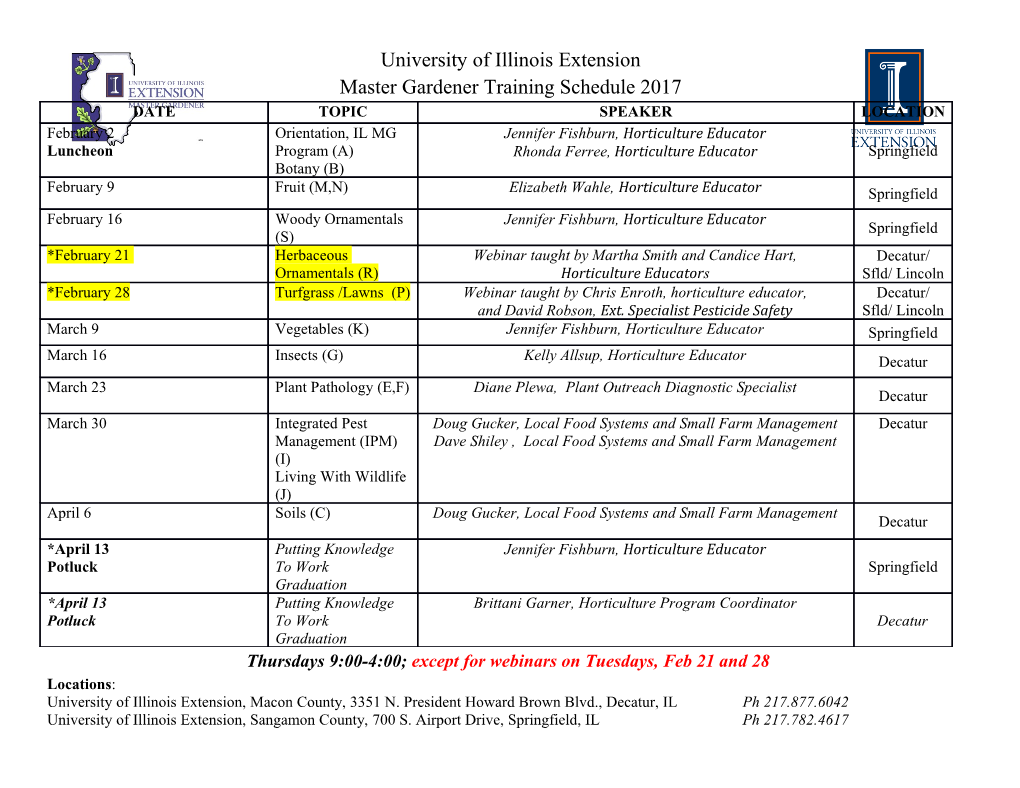
DEGREE PROJECT IN ELECTRICAL ENGINEERING, SECOND CYCLE, 30 CREDITS STOCKHOLM, SWEDEN 2020 Programmable Address Generation Unit for Deep Neural Network Accelerators MUHAMMAD JAZIB KHAN KTH ROYAL INSTITUTE OF TECHNOLOGY SCHOOL OF ELECTRICAL ENGINEERING AND COMPUTER SCIENCE KTH ROYAL INSTITUTE OF TECHNOLOGY Electrical Engineering and Computer Science Programmable Address Generation Unit for Deep Neural Network Accelerators Muhammad Jazib Khan Master in Electrical Engineering Supervisor KTH: Yu Yang Examiner: Prof. Ahmed Hemani School of Electrical Engineering and Computer Science Host company: Robert Bosch GmbH Supervisor Bosch: Sebastian Vogel and Dr. Leonardo Ecco Abstract The Convolutional Neural Networks are getting more and more popular due to their applications in revolutionary technologies like Autonomous Driving, Biomedical Imaging, and Natural Language Processing. With this increase in adoption, the complexity of underlying algorithms is also increasing. This trend entails implications for the computation platforms as well, i.e. GPUs, FPGA, or ASIC based accelerators, especially for the Address Generation Unit (AGU), which is responsible for the memory access. Existing accelerators typically have Parametrizable Datapath AGUs, which have minimal adaptability towards evolution in algorithms. Hence new hardware is required for new algorithms, which is a very inefficient approach in terms of time, resources, and reusability. In this research, six algorithms with different implications for hardware are evaluated for address generation, and a fully Programmable AGU (PAGU) is presented, which can adapt to these algorithms. These algorithms are Standard, Strided, Dilated, Upsampled and Padded convolution, and MaxPooling. The proposed AGU architecture is a Very Long Instruction Word based Application Specific Instruction Processor which has specialized components like hardware counters and zero-overhead loops and a powerful Instruction Set Architecture (ISA), which can model static and dynamic constraints and affine and non-affine Address Equations. The target has been to minimize the flexibility vs. area, power, and performance trade-off. For a working test network of Semantic Segmentation, results have shown that PAGU shows close to the ideal performance, one cycle per address, for all the algorithms under consideration excepts Upsampled Convolution for which it is 1.7 cycles per address. The area of PAGU is approx. 4.6 times larger than the Parametrizable Datapath approach, which is still reasonable considering the high flexibility benefits. The potential of PAGU is not just limited to neural network applications but also in more general digital signal processing areas, which can be explored in the future. Keywords Address Generation Unit; Deep Neural Network Accelerators; Very Long Instruction Word; Application Specific Instruction Processor; Hardware- Software Co-design Abstract Convolutional Neural Networks blir mer och mer populära på grund av deras applikationer inom revolutionerande tekniker som autonom körning, biomedicinsk bildbehandling och naturligt språkbearbetning. Med denna ökning av antagandet ökar också komplexiteten hos underliggande algoritmer. Detta medför implikationer för beräkningsplattformarna såväl som GPU: er, FPGA- eller ASIC-baserade acceleratorer, särskilt för Adressgenerationsenheten (AGU) som är ansvarig för minnesåtkomst. Befintliga acceleratorer har normalt Parametrizable Datapath AGU: er som har mycket begränsad anpassningsförmåga till utveckling i algoritmer. Därför krävs ny hårdvara för nya algoritmer, vilket är en mycket ineffektiv metod när det gäller tid, resurser och återanvändbarhet. I denna forskning utvärderas sex algoritmer med olika implikationer för hårdvara för adressgenerering och en helt programmerbar AGU (PAGU) presenteras som kan anpassa sig till dessa algoritmer. Dessa algoritmer är Standard, Strided, Dilated, Upsampled och Padded convolution och MaxPooling. Den föreslagna AGU-arkitekturen är en Very Long Instruction Word-baserad applikationsspecifik instruktionsprocessor som har specialiserade komponenter som hårdvara räknare och noll-overhead-slingor och en kraftfull Instruktionsuppsättning Arkitektur (ISA) som kan modellera statiska och dynamiska begränsningar och affinera och icke-affinerad adress ekvationer. Målet har varit att minimera flexibiliteten kontra avvägning av område, kraft och prestanda. För ett fungerande testnätverk av semantisk segmentering har resultaten visat att PAGU visar nära den perfekta prestanda, 1 cykel per adress, för alla algoritmer som beaktas undantar Upsampled Convolution för vilken det är 1,7 cykler per adress. Området för PAGU är ungefär 4,6 gånger större än Parametrizable Datapath-metoden, vilket fortfarande är rimligt med tanke på de stora flexibilitetsfördelarna. Potentialen för PAGU är inte bara begränsad till neurala nätverksapplikationer utan också i mer allmänna digitala signalbehandlingsområden som kan utforskas i framtiden. Nyckelord Adressgenereringsenhet; Deep Neural Network Accelerators; Mycket långt instruktionsord; Applikationsspecifik instruktionsprocessor; Hårdvaruprogramvara Samdesign Acknowledgment This research work has been carried out in Robert Bosch GmbH Corporate Research Center, Renningen Germany, in collaboration with KTH Royal Institute of Technology, Stockholm, as the affiliated academic institute. I would like to thank my Examiner, Prof. Ahmed Hemani, and my supervisors, Sebastian Vogel, Leonardo Ecco, and Yu Yang, for providing me constant support on any technical and non-technical hurdles throughout the process. This dissertation is dedicated to my parents and my siblings, M. Muzaffar Khan, A. Moiz Khan, and Maham Khan, who have supported me my whole career and made me who I am today. “ALLAH DOES NOT BURDEN A SOUL BEYOND THAT IT CAN BEAR” (Al-Quran; Surah al Baqarah: 286) i Table of Contents 1 Introduction ............................................................................................................. 1 1.1 Background .................................................................................................................. 1 1.2 Problem .......................................................................................................................... 3 1.3 Purpose ........................................................................................................................... 3 1.4 Goal ................................................................................................................................... 3 1.5 Methodology ................................................................................................................ 4 1.6 Scope................................................................................................................................. 4 1.7 Outline ............................................................................................................................. 5 2 Theoretical Framework & Related Work ................................................. 7 2.1 Convolutional Neural Networks ....................................................................... 7 2.1.1 Classification ........................................................................................................................... 7 2.1.2 Object Localization and Detection ................................................................................ 8 2.1.3 Semantic Segmentation ..................................................................................................... 8 2.2 Hardware Acceleration of DNNs...................................................................... 9 2.2.1 GPU ............................................................................................................................................10 2.2.2 FPGA .........................................................................................................................................10 2.2.3 ASICs .........................................................................................................................................11 2.3 Address Generation Unit .................................................................................... 12 2.3.1 Algorithmic Components of Address Generation ...............................................12 2.3.2 Address Equation Classification ..................................................................................13 Affine, Piece-wise Affine, Non-Linear .................................................................. 13 2.3.3 AGU Classification and Examples ..............................................................................14 Lookup Table Based......................................................................................................... 14 Datapath Based – Parametrizable, Programmable ................................... 15 2.4 Memory Hierarchy ................................................................................................. 16 Temporal / Vector ............................................................................................................ 16 Distributed / Dataflow .................................................................................................. 16 3 Methodology and Design Approach ......................................................... 19 3.1 Assumptions .............................................................................................................. 19 3.1.1 Compiler/Static Optimizations (still needs repositioning)
Details
-
File Typepdf
-
Upload Time-
-
Content LanguagesEnglish
-
Upload UserAnonymous/Not logged-in
-
File Pages85 Page
-
File Size-