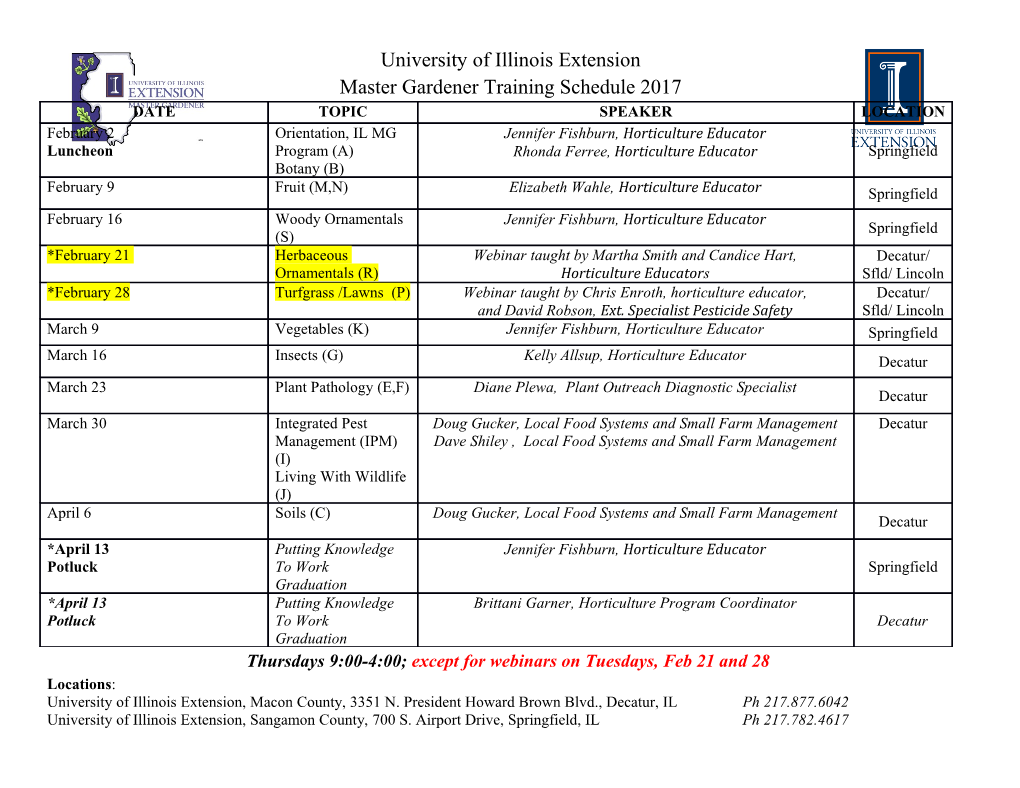
Language Detection Engine for Multilingual Texting on Mobile Devices Sourabh Vasant Gothe, Sourav Ghosh, Sharmila Mani, Guggilla Bhanodai, Ankur Agarwal, Chandramouli Sanchi Samsung R&D Institute Bangalore, Karnataka, India 560037 Email: fsourab.gothe, sourav.ghosh, sharmila.m, g.bhanodai, ankur.a, [email protected] Abstract—More than 2 billion mobile users worldwide type with English word “Ransomware”, in such cases, character- in multiple languages in the soft keyboard. On a monolingual based probabilistic models alone fail to identify the exact keyboard, 38% of falsely auto-corrected words are valid in language as probability will be higher for multiple languages. another language. This can be easily avoided by detecting the language of typed words and then validating it in its respective Also, the user may type based on phonetic sound of the word language. Language detection is a well-known problem in natural that leads to variations like, “somwaar”, “somvar”, “somvaar” language processing. In this paper, we present a fast, light-weight etc. which are completely user dependent. and accurate Language Detection Engine (LDE) for multilingual The soft keyboard provides next-word predictions, word typing that dynamically adapts to user intended language in real- time. We propose a novel approach where the fusion of character completions, auto-correction, etc. while typing. Language N-gram model [1] and logistic regression [2] based selector Models (LMs) responsible for those are built using Long model is used to identify the language. Additionally, we present Short-Term Memory Recurrent Neural Networks (LSTM a unique method of reducing the inference time significantly RNN) [5] based Deep Neural networks (DNN) model [6] with by parameter reduction technique. We also discuss various a character-aware CNN embedding [7]. We use knowledge optimizations fabricated across LDE to resolve ambiguity in input text among the languages with the same character pattern. distilling method proposed by Hinton et al. [8] to train the LM Our method demonstrates an average accuracy of 94.5% for [9]. Along with LMs, adding another DNN based model for Indian languages in Latin script and that of 98% for European detecting the language that executes on every character typed, languages on the code-switched data. This model outperforms will increase the inference time and memory and leads to lag in 1 fastText [3] by 60.39% and ML-Kit by 23.67% in F1 score [4] mobile device. Additionally, in soft-keyboards extensibility is for European languages. LDE is faster on mobile device with an average inference time of 25.91µ seconds. a major concern. Adding one or more language in the keyboard Index Terms—Language detection, multilingual, character N- based on the locality or discontinuing the support of a language gram, logistic regression, parameter reduction, mobile device, should be effortless. Indian macaronic languages, European languages, soft-keyboard Considering the above constraints into account, we present the Language Detection Engine (LDE), an amalgamation of character N-gram models and a logistic regression based I. INTRODUCTION selector model. The engine is fast in inferencing on mobile device, light-weight in model size and accurate for both code- In the current era of social media, language detection switched (switching between the languages) and monolingual is a much required intelligence in mobile device for many text. This paper discusses various optimizations performed to applications viz. translation, transliteration, recommendations, increase engine accuracy compared to DNN based solutions etc. Language detection algorithms work almost accurately in ambiguous cases of the code-switched input text. when the language scripts are distinct using simple script We also discuss how LDE performs on five Indian Mac- arXiv:2101.03963v1 [cs.CL] 7 Jan 2021 detection method. In India, there are 22 official languages, and almost every language has its own script but in general user aronic languages Hinglish (Hindi in English), Marathinglish prefers to type in Latin script. As per our statistical analysis, (Marathi in English), Tenglish (Telugu in English), Tanglish 39.78% of words typed in QWERTY layout are from Indian (Tamil in English), Benglish (Bengali in English) and four languages. Hindi is a popular Indian language, 22.8% of Hindi European languages- Spanish, French, Italian, and German. language users use QWERTY keyboard for typing, that implies Since the typing layout is of English language (Latin script), the need of support for languages written in Latin script. we term English as a primary language and all other languages as a secondary language. Standard languages written in Latin script i.e typed in QWERTY keyboard are referred to as Macaronic languages. These languages can have the same character pattern with other languages, unlike standard ones. For example, when II. RELATED WORK Hindi language is written in Latin script (Hinglish), the word “somwar” which means Monday, shares the same text pattern In this section, we discuss about work related to language detection, both N-gram based and deep learning based ap- 1https://firebase.google.com/docs/ml-kit/android/identify-languages proaches. 978-1-7281-6332-1/20/$31.00 © 2020 IEEE. Personal use of this material is permitted. Permission from IEEE must be obtained for all other uses, in any current or future media, including reprinting/republishing this material for advertising or promotional purposes, creating new collective works, for resale or redistribution to servers or lists, or reuse of any copyrighted component of this work in other works. https://doi.org/10.1109/ICSC.2020.00057 same problem as ours but in a different environment. They 100 Hinglish Tenglish train basic feed-forward network that predicts the language Spanish for every token passed where multiple features are used to obtain the accurate results. However, such models require huge 90 German training data and are not feasible in terms of extensibility and model size for mobile devices. This paper presents a novel method to resolve the ambiguity 80 in input text and detect the language accurately in multilingual soft-keyboard for five Indian macaronic languages and four Accuracy (%) European languges. 70 III. PROPOSED METHOD 60 We propose the Language Detection Engine (LDE) that enhances user experience in multilingual typing by accurately 50 100 150 200 250 deducing the language of input text in real-time. LDE is a Corpus Size (×1000 lines) union of (a) character N-gram model which gives emission probability of input text originating from a particular language, Fig. 1: Char N-gram accuracy over corpus size and (b) a selector model which uses the emission probabilities to identify the most probable language for a given text using logistic regression [15]. Unique architecture of independent A. N-gram based models character N-gram models with selector model is able to detect Ahmed et al. [10] detail about language identification using the code-mixed multilingual context accurately. N-gram based cumulative frequency addition to increase the A. Emission probability estimation using Character N-gram classification speed of a document. It is achieved by reducing counting and sorting operations by cumulative frequency addi- Character N-gram is a statistical model which estimates tion method. In our problem, we detect the language, based on probability distribution over the character set of a particular user typed text rather than document with large information. language given its corpus. Vatanen et al. [1] compared the naive bayes classifer based 1) Train data: Training corpus is generated by crawling charater N-gram and ranking method for language detection online data from various websites. For Indian macaronic task. Their paper focuses on detecting short segments of length languages, we crawled the native script (Example: Devanagari 5 to 21 characters, and all the language models are constructed script for Hindi) data of the languages and reverse translit- independently of each other without considering the final clas- erated to Latin script. This data is validated by the language sification in the process. We have adopted similar methodology experts for quality purpose. for building char N-gram models in our approach. We experimented with various sizes of corpus to train Erik Tromp et al. [11] discuss Graph-Based N-gram Lan- character N-gram model and found out that 100k sentences guage Identification (LIGA) for short and ill-written texts. show the best accuracy from the model on sample test set, as LIGA outperforms other N-gram based models by capturing detailed in Fig. 1. the elements of language grammar as a graph. However LIGA 2) Model training: For every supported language li, we does not handle the case of code-switched text. train Character N-gram model Cli independent of the other All the above referred models do not prioritize recently languages as shown in Fig. 2. Probability of a sequence of typed words. For seamless multilingual texting which involves words (t1::n) in language li is given by, continuous code-switching, more priority must be given to the n Y rn−k recent words so that the suggestions from currently detected Pli (t1::n) = Pli (tk) ; where r 2 (0; 1] (1) language model can be fetched from the soft keyboard and k=1 shown to the user. We prioritize the probability of most recent word over the B. Deep learning based models previous words using a variable r, value ranging between 0 and 1, that effectively reduces the impact of the leading Lopez et al. propose a DNN based language detection for words probability by converging values closer to 1. To prevent spoken utterance [12] motivated by the success in acoustic the underflow of values we use logarithmic probabilities. modeling using DNN. They train a fully connected feed- Mathematically, forward neural network along with the logisic regression n calibration to identify the exact language. Javier Gonzalez- X log P (t ) = rn−k · log P (t ) Dominguez et al.
Details
-
File Typepdf
-
Upload Time-
-
Content LanguagesEnglish
-
Upload UserAnonymous/Not logged-in
-
File Pages8 Page
-
File Size-