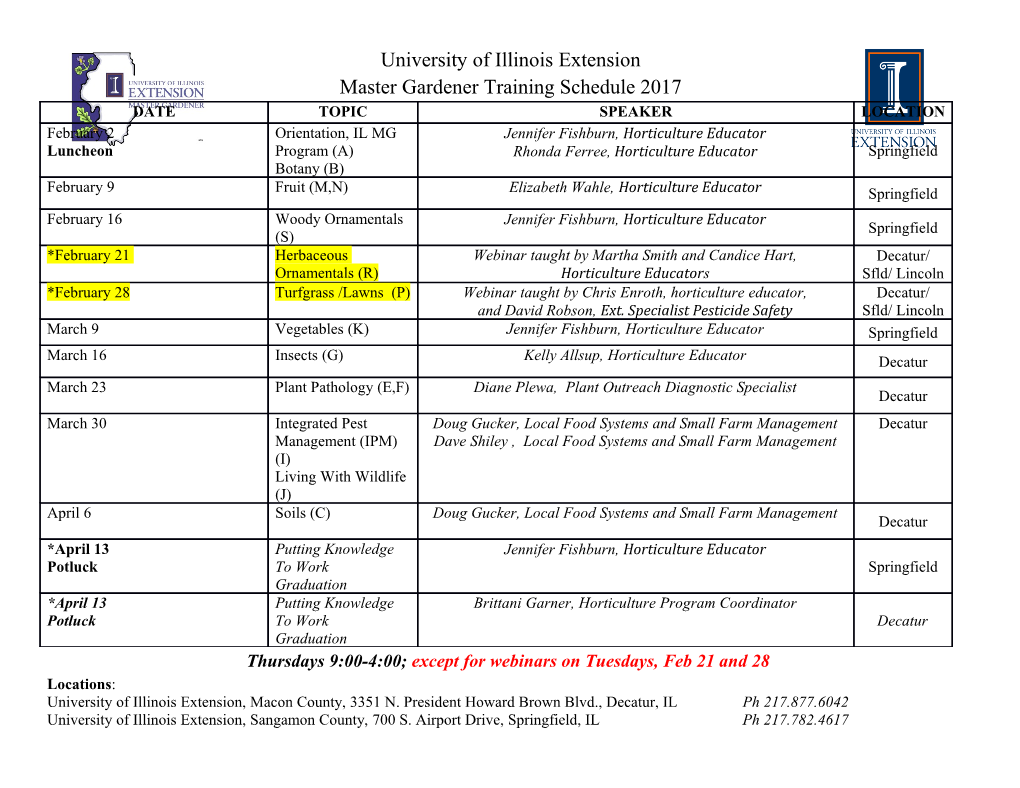
Chapter 6 Linear operators and adjoints Contents Introduction .......................................... ............. 6.1 Fundamentals ......................................... ............. 6.2 Spaces of bounded linear operators . ................... 6.3 Inverseoperators.................................... ................. 6.5 Linearityofinverses.................................... ............... 6.5 Banachinversetheorem ................................. ................ 6.5 Equivalenceofspaces ................................. ................. 6.5 Isomorphicspaces.................................... ................ 6.6 Isometricspaces...................................... ............... 6.6 Unitaryequivalence .................................... ............... 6.7 Adjoints in Hilbert spaces . .............. 6.11 Unitaryoperators ...................................... .............. 6.13 Relations between the four spaces . ................. 6.14 Duality relations for convex cones . ................. 6.15 Geometric interpretation of adjoints . ............... 6.15 Optimization in Hilbert spaces . .............. 6.16 Thenormalequations ................................... ............... 6.16 Thedualproblem ...................................... .............. 6.17 Pseudo-inverseoperators . .................. 6.18 AnalysisoftheDTFT ..................................... ............. 6.21 6.1 Introduction The field of optimization uses linear operators and their adjoints extensively. Example. differentiation, convolution, Fourier transform, Radon transform, among others. Example. If A is a n m matrix, an example of a linear operator, then we know that y Ax 2 is minimized when x = 1 × k − k [A0A]− A0y. We want to solve such problems for linear operators between more general spaces. To do so, we need to generalize “transpose” and “inverse.” 6.1 6.2 c J. Fessler, December 21, 2004, 13:3 (student version) 6.2 Fundamentals We write T : D when T is a transformation from a set D in a vector space to a vector space . →Y X Y The set D is called the domain of T . The range of T is denoted R(T ) = y : y = T (x) for x D . { ∈Y ∈ } If S D, then the image of S is given by ⊆ T (S) = y : y = T (s) for s S . { ∈Y ∈ } If P , then the inverse image of P is given by ⊆Y 1 T − (P ) = x D : T (x) P . { ∈ ∈ } Notation: for a linear operator A, we often write Ax instead of A(x). For linear operators, we can always just use D = , so we largely ignore D hereafter. X Definition. The nullspace of a linear operator A is N(A) = x : Ax = 0 . It is also called the kernel of A, and denoted ker(A). { ∈X } Exercise. For a linear operator A, the nullspace N(A) is a subspace of . Furthermore, if A is continuous (in a normed space ), then N(A) is closedX [3, p. 241]. X Exercise. The range of a linear operator is a subspace of . Y Proposition. A linear operator on a normed space (to a normed space ) is continuous at every point if it is continuous at a single point in . X Y X X Proof. Exercise. [3, p. 240]. Luenberger does not mention that needs to be a normed space too. Y Definition. A transformation T from a normed space to a normed space is called bounded iff there is a constant M such that T (x) M x , x . X Y k k ≤ k k ∀ ∈X Definition. The smallest such M is called the norm of T and is denoted T . Formally: ||| ||| T , inf M R : T (x) M x , x . ||| ||| { ∈ k k ≤ k k ∀ ∈ X } Consequently: T (x) T x , x . k kY ≤ ||| |||k kX ∀ ∈X Fact. For a linear operator A, an equivalent expression (used widely!) for the operator norm is A = sup Ax . ||| ||| x 1 k k k k≤ Fact. If is the trivial vector space consisting only of the vector 0, then A = 0 for any linear operator A. X ||| ||| Fact. If is a nontrivial vector space, then for a linear operator A we have the following equivalent expressions: X Ax A = sup k k = sup Ax . ||| ||| x=0 x x =1 k k 6 k k k k c J. Fessler, December 21, 2004, 13:3 (student version) 6.3 ................................................... ................................................... ................ Example. Consider = (Rn, ) and = R. X k·k∞ Y Clearly Ax = a1x1 + +anxn so Ax = Ax = a1x1 + +anxn a1 x1 + + an xn ( a1 + + an ) x . · · · k kY | | | · · · | ≤ | || | · · · | || | ≤ | | · · · | | k k∞ In fact, if we choose x such that xi = sgn(ai), then x = 1 we get equality above. So we conclude A = a1 + + an . k k∞ ||| ||| | | · · · | | Example. What if = Rn, ? ?? X k·kp Proposition. A linear operator is bounded iff it is continuous. Proof. Exercise. [3, p. 240]. More facts related to linear operators. If A : is linear and is a finite-dimensional normed space, then A is continuous [3, p. 268]. • X →Y X If A : is a transformation where and are normed spaces, then A is linear and continuous iff A( ∞ α x ) = • X →Y X Y i=1 i i i∞=1 αiA(xi) for all convergent series i∞=1 αixi. [3, p. 237]. This is the superposition principle as described in introductory signals and systems courses. P P P Spaces of bounded linear operators Definition. If T1 and T2 are both transformations with a common domain and a common range , over a common scalar field, then we define natural addition and scalar multiplication operations as follows:X Y (T1 + T2)(x) = T1(x) + T2(x) (αT1)(x) = α(T1(x)). Lemma. With the preceding definitions, when and are normed spaces the following space of operators (!) is a vector space: X Y B( , ) = bounded linear transformations from to . X Y { X Y} (The proof that this is a vector space is within the next proposition.) This space is analogous to certain types of dual spaces (see Ch. 5). Not only is B( , ) a vector space, it is a normed space when one uses the operator norm A defined above. X Y ||| ||| Proposition. (B( , ), ) is a normed space when and are normed spaces. X Y |||· ||| X Y Proof. (sketch) Claim 1. B( , ) is a vector space. Suppose T ,TX Y B( , ) and α . 1 2 ∈ X Y ∈F (αT1 + T2)(x) = αT1(x) + T2(x) α T1(x) + T2(x) α T1 x + T2 x = K x , where k kY k kY ≤ | | k kY k kY ≤ | |||| |||k kX ||| |||k kX k kX K , α T + T . So αT + T is a bounded operator. Clearly αT + T is a linear operator. | |||| 1 ||| ||| 2 ||| 1 2 1 2 Claim 2. is a norm on B. The “hardest”|||· ||| part is verifying the triangle inequality: T1 + T2 = sup (T1 + T2)x sup T1 x + sup T2 x = T1 + T2 . ||| ||| x =1 k kY ≤ x =1 k kY x =1 k kY ||| ||| ||| ||| k k k k k k 2 Are there other valid norms for B( , )? ?? X Y Remark. We did not really “need” linearity in this proposition. We could have shown that the space of bounded transformations from to with is a normed space. X Y |||· ||| 6.4 c J. Fessler, December 21, 2004, 13:3 (student version) Not only is B( , ) a normed space, but it is even complete if is. X Y Y Theorem. If and are normed spaces with complete, then (B( , ), ) is complete. X Y Y X Y |||· ||| Proof. Suppose T is a Cauchy sequence (in B( , )) of bounded linear operators, i.e., T T 0 as n, m . { n} X Y ||| n − m||| → → ∞ Claim 0. x , the sequence T (x) is Cauchy in . ∀ ∈X { n } Y Tn(x) Tm(x) = (Tn Tm)(x) Tn Tm x 0 as n, m . k − kY k − kY ≤ ||| − |||k kX → → ∞ Since is complete, for any x the sequence T (x) converges to some point in . (This is called pointwise convergence.) Y ∈X { n } Y So we can define an operator T : by T (x) , limn Tn(x). X →Y →∞ To show B is complete, we must first show T B, i.e., 1) T is linear, 2) T is bounded. Then we show 3) T T (convergence w.r.t.∈ the norm ). n → |||· ||| Claim 1. T is linear T (αx + z) = lim Tn(αx + z) = lim [αTn(x) + Tn(z)] = αT (x) + T (z) . n n →∞ →∞ (Recall that in a normed space, if u u and v v, then αu + v αu + v.) n → n → n n → Claim 2: T is bounded Since T is Cauchy, it is bounded, so K < s.t. T K, n N. Thus, by the continuity of norms, for any x : { n} ∃ ∞ ||| n||| ≤ ∀ ∈ ∈X T (x) = lim Tn(x) lim Tn x K x . n n k kY →∞ k kY ≤ →∞ ||| |||k kX ≤ k kX Claim 3: Tn T Since T is→ Cauchy, { n} ε > 0, N N s.t. n, m > N = T T ε ∀ ∃ ∈ ⇒ ||| n − m||| ≤ = Tn(x) Tm(x) ε x , x ⇒ k − kY ≤ k kX ∀ ∈X = lim Tn(x) Tm(x) ε x , x m ⇒ →∞ k − kY ≤ k kX ∀ ∈X = Tn(x) T (x) ε x , x by continuity of the norm ⇒ k − kY ≤ k kX ∀ ∈X = T T ε. ⇒ ||| n − ||| ≤ We have shown that every Cauchy sequence in B( , ) converges to some limit in B( , ), so B( , ) is complete. 2 X Y X Y X Y Corollary. (B( , R), ) is a Banach space for any normed space . X |||· ||| X Why? ?? We write A B( , ) as shorthand for “A is a bounded linear operator from normed space to normed space .” ...................................................∈ X Y ...................................................X ................Y Definition. In general, if S : and T : , then we can define the product operator or composition as a transformation T S : by (TSX →Y)(x) = T (S(x))Y. → Z X → Z Proposition. If S B( , ) and T B( , ), then TS B( , ). ∈ X Y ∈ Y Z ∈ X Z Proof. Linearity of the composition of linear operators is trivial to show. To show that the composition is bounded: TSx T Sx T S x . 2 k kZ ≤ ||| |||k kY ≤ ||| |||||| |||k kX Does it follow that T S = T S ? ?? ||| ||| ||| |||||| ||| c J. Fessler, December 21, 2004, 13:3 (student version) 6.5 6.3 Inverse operators Definition. T : is called one-to-one mapping of into iff x , x and x = x = T (x ) = T (x ). X →Y X Y 1 2 ∈X 1 6 2 ⇒ 1 6 2 1 Equivalently, T is one-to-one if the inverse image of any point y is at most a single point in , i.e., T − ( y ) 1, y .
Details
-
File Typepdf
-
Upload Time-
-
Content LanguagesEnglish
-
Upload UserAnonymous/Not logged-in
-
File Pages27 Page
-
File Size-