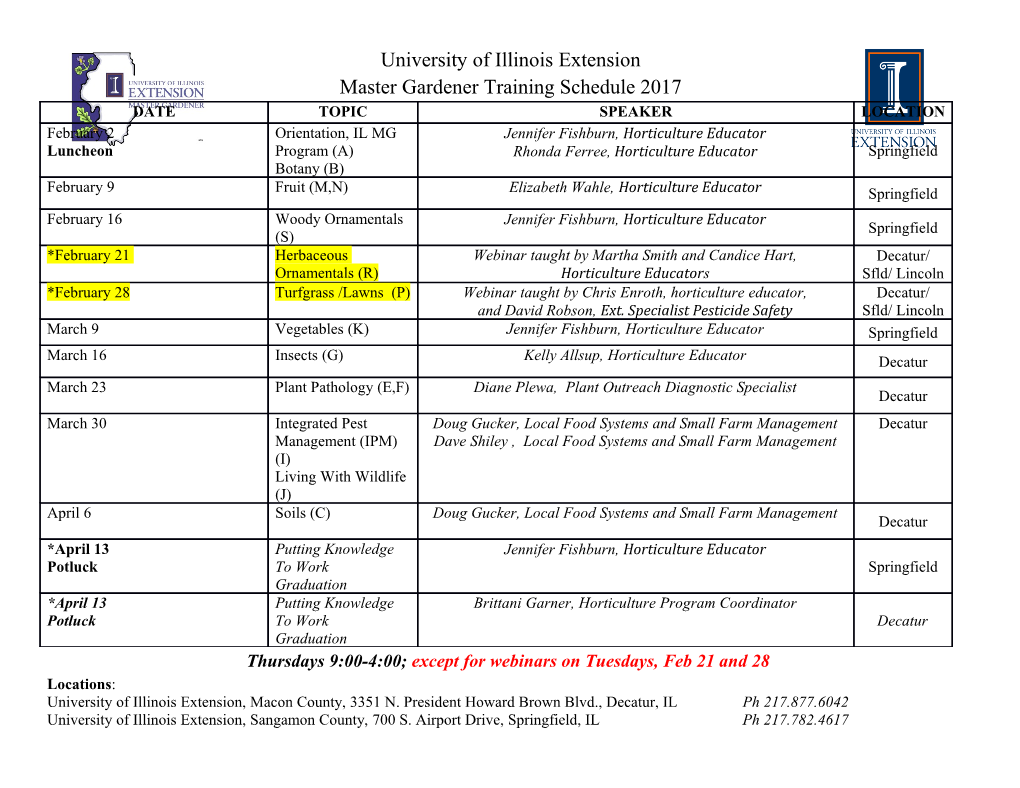
STAT 141 REGRESSION: CONFIDENCE vs PREDICTION INTERVALS 12/2/04 Inference for coefficients Mean response at x vs. New observation at x Linear Model (or Simple Linear Regression) for the population. (“Simple” means single explanatory variable, in fact we can easily add more variables ) – explanatory variable (independent var / predictor) – response (dependent var) Probability model for linear regression: 2 i ∼ N(0, σ ) independent deviations Yi = α + βxi + i, α + βxi mean response at x = xi 2 Goals: unbiased estimates of the three parameters (α, β, σ ) tests for null hypotheses: α = α0 or β = β0 C.I.’s for α, β or to predictE(Y |X = x0). (A model is our ‘stereotype’ – a simplification for summarizing the variation in data) For example if we simulate data from a temperature model of the form: 1 Y = 65 + x + , x = 1, 2,..., 30 i 3 i i i Model is exactly true, by construction An equivalent statement of the LM model: Assume xi fixed, Yi independent, and 2 Yi|xi ∼ N(µy|xi , σ ), µy|xi = α + βxi, population regression line Remark: Suppose that (Xi,Yi) are a random sample from a bivariate normal distribution with means 2 2 (µX , µY ), variances σX , σY and correlation ρ. Suppose that we condition on the observed values X = xi. Then the data (xi, yi) satisfy the LM model. Indeed, we saw last time that Y |x ∼ N(µ , σ2 ), with i y|xi y|xi 2 2 2 µy|xi = α + βxi, σY |X = (1 − ρ )σY Example: Galton’s fathers and sons: µy|x = 35 + 0.5x ; σ = 2.34 (in inches). For comparison: σY = 2.7. Note: σ is SD of y given x. It is less than the unconditional SD of Y , σY (without x fixed). e.g. consider extreme case when y = x: σ=0, but σY = σX . Estimating parameters (α, β, σ2) from a sample P 2 Recall the least squares estimates: (a,b) chosen to minimize (yi − a − bxi) : P sy (xi − x¯)(yi − y¯) a =y ¯ − bx¯ b = r = P 2 sx (xi − x¯) Fitted values: yˆ = a + bxi Residuals: ei = yi − yˆi = yi − a − bxi = α + βxi + i − a − bxi = (α − a) + (β − b)xi + i e = deviations in sample, where i i = deviations in model Estimate of σ2: Again, use the squared scale: 2 1 X 2 1 X 2 q 2 sY |x = ei = (yi − a − bxi)i , s = sY |x (n − 2) = “degrees of freedom” n − 2 i n − 2 i Why n-2?? 1 2 2 1 P 2 1. Recall with SRS Yi ∼ N(µ, σ ), s = n−1 (yi − y¯) (and y¯ estimates µ – we estimate one parameter) Here, two parameter estimates: a estimates α , and b estimates β. 2. There are two linear constraints on ei: • e¯ =y ¯ − a − bx¯ =y ¯ − (¯y − bx¯) − bx¯ = 0 P • (xi − x¯)ei = 0 2 2 Properties of Regression Estimates. Assume model (LM ): then (a, b, s = sY |x) are random variables. 2 2 2 1. Means (a, b, s = sY |x) are unbiased estimates of (α, β, σ ) 2. Variances For (a, b) variances are ( 2 ) 2 2 2 1 x¯ 2 σ σa = V ar(a) = σ + P 2 , σb = V ar(b) = P 2 n (xi − x¯) (xi − x¯) 3. (a, b) are jointly normal – in particular s2 a ∼ N(α, σ2), b ∼ N(β, σ2), (n − 2) ∼ χ2 (and a,b, and s2 are independent). a b σ2 n−2 Confidence Intervals: at level α CI’s always have the form: Est ± t α SE 1− 2 ,(n−2) Est SEEstmeans σEst with σ replaced by its estimate s. Thus v u 2 s u 1 x¯ SEb = q , SEa = st + P 2 n P(x − x¯)2 (xi − x¯) i slope b ± t1− α ,(n−2)SEb Thus, (1 − α)% CI for: 2 intercept a ± t α SE 1− 2 ,(n−2) a Tests Described here for slope β , ( but same for intercept α). b−β0 For H0 : β = β0, use t = which under H0 has the tn−2 distribution. SEb P values: One sided: (e.g.) H1 : β < β0, P = P (tn−2 < tobs) Two sided: H1 : β 6= β0, P = 2P (tn−2 > |tobs|) ( Aside: Why tn−2? t ∼ N(0,1) By definition ν q 2 , with numerator and denominator variable independent. But we can write the χν ν slope test statistic in the form (b − β )/σ t = 0 b r 2 SEb 2 σb and now we can note from properties (1) – (3) that 2 2 2 2 2 (b − β0)/σb ∼ N(0, 1) and SEb /σb = s /σ ∼ χn−2 2 and it can be shown that b and s are independent. This shows that the test statistic t ∼ tn−2.) 2 Vs New Observation at x Mean response at x new ∗ ∗ new ∗ Want to predict y (x ) = α + βx + Want to estimate µY |x∗ = α + βx ∗ ∗ new draw of y from subpopulation given x = x mean for subpopulation given x = x ∗ ∗ ∗ Point prediction:yˆ(x ) = a + bx Point estimate:µˆ ∗ = a + bx Y |x Sources of uncertainty :a, b estimated. Sources of uncertainty :a, b estimated. random error of new observ. v u 1 (x∗ − x¯)2 2 2 2 u SE ∗ = s + SE SE = st + yˆ(x ) Y |x µˆY |x∗ µˆY |x∗ P 2 n (xi − x¯) Prediction Interval : level (1 − α)% n-2 df CI :level (1 − α)% n-2 df ∗ yˆ(x ) ± t1−α/2,n−2SEyˆ(x∗) ∗ µˆY |x ± t1−α/2,n−2SEµˆY |x∗ Claim: 95% of the time , Pred. Interv. covers Claim: 95% of the time , CI covers new ∗ ∗ y (x ) µ ∗ = α + βx Y |x ie ie new yˆ − t1−α/2,n−2SE ≤ y ≤ yˆ + t1−α/2,n−2SE µˆ − t1−α/2,n−2SEµˆ ≤ µ ≤ µˆ + t1−α/2,n−2SEµˆ predict(lm(y ~ x), data, > predict(lm(y ~ x), new, interval="prediction",se.fit=T) interval="confidence",se.fit=T) $fit $fit fit lwr upr fit lwr upr 1 -1.827565 -3.99873 0.34360 1 -1.82756 -3.165220 -0.48991 2 -1.558351 -3.60936 0.49266 2 -1.55835 -2.690602 -0.42610 3 -1.289137 -3.23751 0.65924 3 -1.28914 -2.222694 -0.35558 4 -1.019922 -2.88608 0.84624 4 -1.01992 -1.766869 -0.27298 3.
Details
-
File Typepdf
-
Upload Time-
-
Content LanguagesEnglish
-
Upload UserAnonymous/Not logged-in
-
File Pages3 Page
-
File Size-