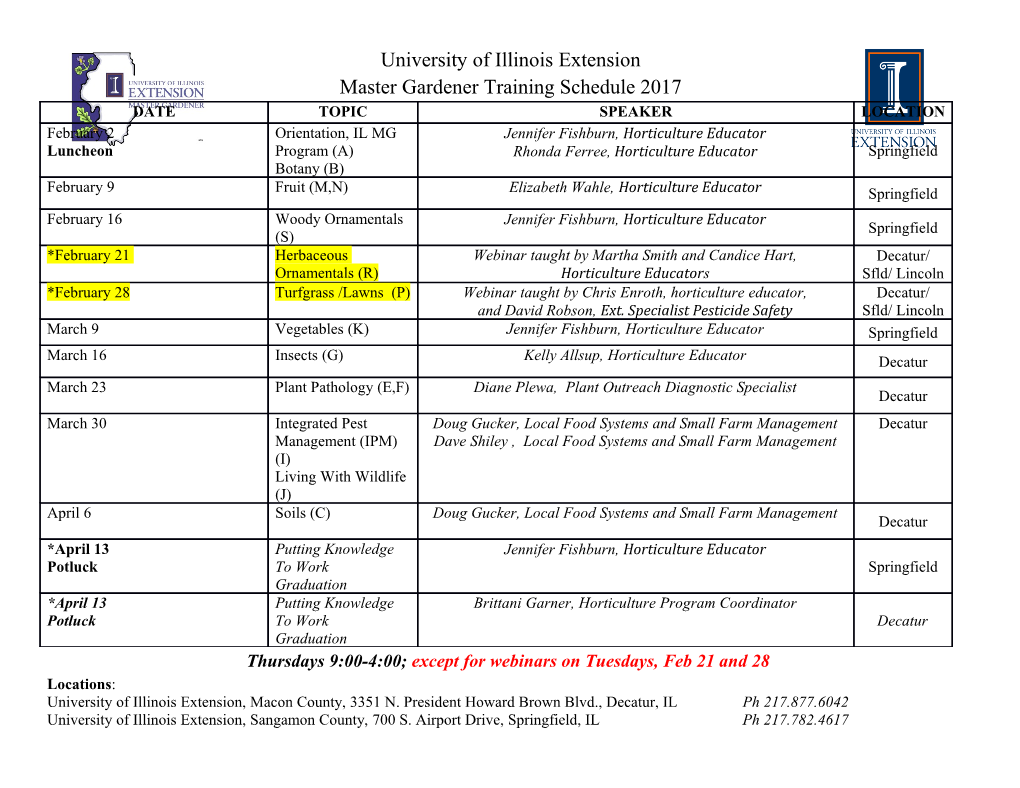
Vol. 40: 147–161, 2009 CLIMATE RESEARCH Published December 10 doi: 10.3354/cr00821 Clim Res Contribution to CR Special 20 ‘Integrating analysis of regional climate change and response options’ OPENPEN ACCESSCCESS Growing-season rainfall and scenarios of future change in southeast Africa: implications for cultivating maize Mark Tadross1,*, Pablo Suarez2, Alex Lotsch3, Sepo Hachigonta1, Marshall Mdoka1, Leonard Unganai4, Filipe Lucio5, Donald Kamdonyo6, Maurice Muchinda7 1Climate Systems Analysis Group, Department of Environmental & Geographical Science, University of Cape Town, Rondebosch 7701, South Africa 2Red Cross/Red Crescent Climate Centre, Leeghwaterplein 27, 2521 CV Den Haag, The Netherlands 3Commodity Risk Management Group, Agriculture and Rural Development, World Bank, 1818 H Street, Washington, DC, USA 4National Meteorological Service of Zimbabwe, PO Box BE150, Belvedere, Harare, Zimbabwe 5Instituto Nacional de Meteorologia, Rua de Mukumbura 164, C. P. 256, Maputo, Mozambique 6National Meteorological Service of Malawi, Llilongwe, Malawi 7National Meteorological Service of Zambia, Lusaka, Zambia ABSTRACT: Global climate change is a detectable and attributable global phenomenon, yet its man- ifestation at the regional scale, especially within the rainfall record, can be difficult to identify. This problem is particularly acute over southern Africa, a region characterised by a low density of obser- vations and highly dependent on rural agriculture, where the impact of rainfall changes on maize cul- tivation critically depends on the timing with respect to the crop phenological cycle. To evaluate changes in rainfall affecting maize cropping, daily rainfall observations from 104 stations across Malawi, Mozambique, Zambia and Zimbabwe were used to detect trends in planting dates, rainfall cessation and duration of the rainfall season, as well as number of dry days, length of dry spells and measures of rainfall intensity during critical periods for growing maize. Correlations with the South- ern Oscillation Index (SOI) and Antarctic Oscillation (AAO) were used to infer how large-scale cli- mate variability affects these attributes of rainfall and highlight where (and when) trends may con- tribute to more frequent crossings of critical thresholds. The El Niño Southern Oscillation (ENSO) was associated with changes in planting and cessation dates as well as the frequency of raindays dur- ing the rainfall season (particularly early in the season). AAO mainly affected raindays towards the end of the season when maize was planted late. Trends are discussed relative to changes projected in empirically downscaled scenarios of rainfall from 7 general circulation models for the 2046–2065 period, assuming an SRES A2 emissions scenario. KEY WORDS: Rainfall · Maize · Crops · Africa · Climate change · Downscaling Resale or republication not permitted without written consent of the publisher 1. INTRODUCTION the Southern African Development Community (SADC) region (excluding South Africa, but including Africa is highly vulnerable to climate change, largely Zimbabwe, Zambia, Malawi and Mozambique) fell because many socioeconomic activities in Africa, par- from an average of 11.3 million to 6.2 million t, neces- ticularly agriculture, depend on climate and especially sitating extensive food imports (FAO 2004). Further- rainfall. Within the agriculture sector, drought is more, southern Africa’s agriculture sector is particu- arguably the most important climatic challenge and larly vulnerable to climate change, with potentially has major impacts on rural livelihoods (Buckland et al. negative impacts on revenue from dryland farming 2000). During the 1991–1992 drought, cereal output in (Kurukulasuriya et al. 2006). There is therefore a press- *Email: [email protected] © Inter-Research 2009 · www.int-res.com 148 Clim Res 40: 147–161, 2009 ing need to assess the capacity of southern African bique, Zambia and Zimbabwe. To see if observed countries to cope with, and adapt to, the impact of cli- trends were consistent with expected future changes mate change within the agriculture sector. we then compared these results with empirically One major problem for any climate adaptation initia- downscaled rainfall projections for the mid-21st cen- tive, particularly at the local level, is insufficient infor- tury from 7 general circulation models (GCMs, forced mation about what to adapt to. This often results from using the SRES A2 emissions scenario). This compari- a lack of awareness of how the climate has changed in son was used to suggest where and when the 2 data the past, how it is expected to change in the future and sets were (in)consistent and highlighted some of the if observed changes are consistent with projected difficulties in using either observations or climate pro- changes based on models of the climate system (e.g. jections to suggest impacts (and implied adaptation Jones et al. 2004, Tadross et al. 2005b, Hewitson & options) in the future. Crane 2006). Complications may also arise when attempting to relate changes in monthly or seasonal averages to impacts in a particular sector. For the agri- 2. DATA AND METHODS cultural sector, it is crucial to know how rainfall charac- teristics which affect planting dates and the crop 2.1. Study region growth cycle (Dennett 1987, Ati et al. 2002, Usman et al. 2005) may be changing. Fig. 1 presents the study region and the geographi- Besides long-term change, year-to-year variability cal distribution of the 104 stations used in the present and its effect on farm management decisions is also study. The rainfall season is unimodal, with the start crucial. The superposition of this shorter-term climate of the rains typically occurring during September– variability on longer term change, within a region that October in the far north and south, whereas rains operates close to critical thresholds, is key to formu- arrive later (November–December) in the central lating adequate adaptation options. Whilst aspects of regions (Reason et al. 2005, Tadross et al. 2005a). Rain- year-to-year climate variability, such as the El Niño fed crop production normally occurs during December– Southern Oscillation (ENSO) and Antarctic Oscillation February, which is when rainfall variability and hence (AAO), have a well-documented relationship with sea- rain-fed crop production often varies with ENSO; e.g. sonal climate anomalies in the region (e.g. Lindesay there is a strong relationship demonstrated between 1988, Mason 1995, Richard et al. 2000, Hulme et al. maize yields and ENSO in Zimbabwe (Cane et al. 2001, Reason & Rouault 2005), relatively fewer studies 1994). Whilst these relationships between total sea- have investigated relationships with rainfall character- sonal rainfall and maize yield are clear, it is often the istics that are closely tied to management decisions intraseasonal character of rainfall (start of the rains, and the crop growth cycle (e.g. Usman & Reason 2004 dry spells, etc.) that is important. For example, Phillips Reason et al. 2005, Tadross et al. 2005a). Certainly, et al. (1998) demonstrated that earlier planting during within southern Africa little is currently known regard- ENSO years can avoid the mid-season dry spell or ing how trends (see New et al. 2006) and variability nitrogen-removing excessive rainfall when the maize combine to affect daily rainfall characteristics at the crop is in its most vulnerable growth phase. The impact local level and the potential impacts on crops. of rainfall variability on maize production is therefore The present study explored observed changes in clearly tied to the planting date and timing with daily rainfall records and evaluated the agricultural respect to the crop phenological phases. implications of these changes by calculating indices that can be directly related to farm management deci- sions and impacts on crop growth. These indices, 2.2. Rainfall data based on the frequency and intensity of rainfall, were developed to represent planting dates, rainfall cessa- Metadata for observed daily station rainfall data tion, dry spell length, frequency of dry days, rainfall used in this analysis are given in Appendix 1. All the intensity and total rainfall during critical stages of the station data were quality controlled using Rclimdex crop growth cycle. As maize is the main SADC software (http://cccma.seos.uvic.ca/ETCCDMI/index. food/nutrition source (Smale & Jayne 2003), we tai- shtml) which tested for negative precipitation and long lored these indices to be relevant for growing maize. periods of zero precipitation (see Peterson et al. 2002, To understand how variations in these indices (and New et al. 2006). Daily precipitation values >450 mm, the suggested impact on maize cropping) are linked whilst potentially real, are also potentially erroneous to large-scale climate modes, we correlated them to and were removed as a precaution. All negative rain- ENSO and AAO and calculated trends using daily fall values were removed. Missing data were often rainfall data from 104 stations across Malawi, Mozam- present, especially over Mozambique during periods Tadross et al.: Growing-season rainfall changes and maize 149 10° S 15° 20° 25° 25°E 30° 35° 40° Fig. 1. Study area with meteorological stations of civil strife, and an increase in missing data over time specific farm management decisions facing a farmer can lead to false trends. Therefore, to prevent missing planting rain-fed crops: when to plant and which seed data from introducing false trends
Details
-
File Typepdf
-
Upload Time-
-
Content LanguagesEnglish
-
Upload UserAnonymous/Not logged-in
-
File Pages15 Page
-
File Size-