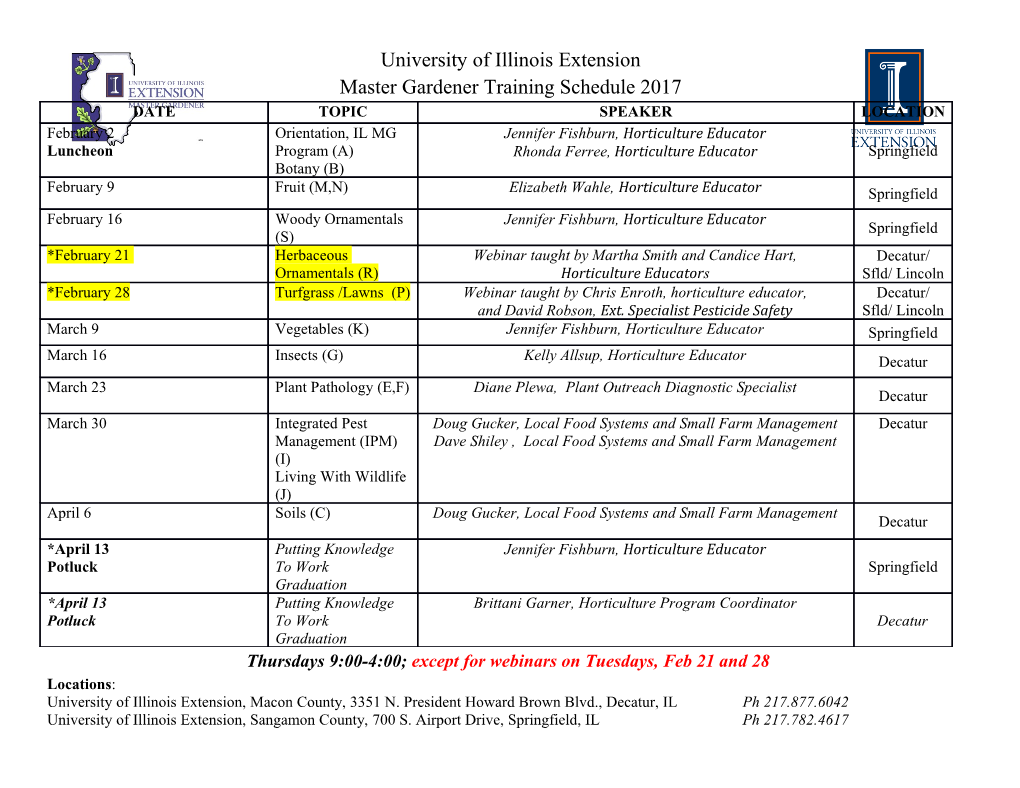
fgene-12-698570 July 30, 2021 Time: 16:49 # 1 ORIGINAL RESEARCH published: 05 August 2021 doi: 10.3389/fgene.2021.698570 Comprehensive Bioinformatics Analysis Identifies POLR2I as a Key Gene in the Pathogenesis of Hypertensive Nephropathy Shilong You, Jiaqi Xu, Boquan Wu, Shaojun Wu, Ying Zhang*, Yingxian Sun* and Naijin Zhang* Department of Cardiology, The First Hospital of China Medical University, Shenyang, China Hypertensive nephropathy (HN), mainly caused by chronic hypertension, is one of the major causes of end-stage renal disease. However, the pathogenesis of HN remains unclarified, and there is an urgent need for improved treatments. Gene expression profiles for HN and normal tissue were obtained from the Gene Expression Omnibus Edited by: database. A total of 229 differentially co-expressed genes were identified by weighted Zhi-Ping Liu, Shandong University, China gene co-expression network analysis and differential gene expression analysis. These Reviewed by: genes were used to construct protein–protein interaction networks to search for hub Mohadeseh Zarei Ghobadi, genes. Following validation in an independent external dataset and in a clinical database, University of Tehran, Iran POLR2I, one of the hub genes, was identified as a key gene related to the pathogenesis Menghui Yao, First Affiliated Hospital of Zhengzhou of HN. The expression level of POLR2I is upregulated in HN, and the up-regulation of University, China POLR2I is positively correlated with renal function in HN. Finally, we verified the protein *Correspondence: levels of POLR2I in vivo to confirm the accuracy of our analysis. In conclusion, our Naijin Zhang [email protected] study identified POLR2I as a key gene related to the pathogenesis of HN, providing new Yingxian Sun insights into the molecular mechanisms underlying HN. [email protected] Ying Zhang Keywords: hypertensive nephropathy (HN), weighted gene co-expression network analysis (WGCNA), [email protected] differentially expressed genes (DEGs), pathogenesis, key gene, POLR2I Specialty section: This article was submitted to INTRODUCTION Computational Genomics, a section of the journal Hypertension is a disease that usually leads to the impairment of target organs, especially kidney. Frontiers in Genetics Hypertensive nephropathy (HN), mainly caused by chronic hypertension, is one of the major Received: 28 April 2021 causes of end-stage renal disease. It has been estimated that 60–90% of patients with chronic Accepted: 08 July 2021 kidney disease are hypertensive (Ku et al., 2019). However, existing data have demonstrated that Published: 05 August 2021 even when blood pressure is reduced to the recommended goal, it can only slow, but not stop, Citation: the progression of HN (Udani et al., 2011). Therefore, in addition to effectively reducing blood You S, Xu J, Wu B, Wu S, pressure, it is particularly important to understand the pathogenesis of hypertensive nephropathy. Zhang Y, Sun Y and Zhang N (2021) The tubulointerstitial compartment constitutes 95% of the total kidney mass (Berthier et al., 2012), Comprehensive Bioinformatics and the tubulointerstitial changes in HN patients are deemed as a major determinant in the Analysis Identifies POLR2I as a Key Gene in the Pathogenesis development of renal damage (Nath, 1992). Moreover, interstitial changes in hypertension-induced of Hypertensive Nephropathy. renal injury occurs before glomerular changes become apparent, suggesting that tubulointerstitial Front. Genet. 12:698570. compartments may be the crucial initial site of injury (Mai et al., 1993). However, the detailed doi: 10.3389/fgene.2021.698570 molecular mechanism of tubulointerstitial lesions in HN is poorly understood. Frontiers in Genetics| www.frontiersin.org 1 August 2021| Volume 12| Article 698570 fgene-12-698570 July 30, 2021 Time: 16:49 # 2 You et al. The Key Genes in HN A growing body of evidence supported that genetic (RLE) analysis were performed to evaluate the removal of batch background affects the progression of nephropathy in HN effect. After using hierarchical clustering to identify and exclude patients (Zhang et al., 2013; Guo et al., 2019; Sun et al., 2020). outliers, samples from the HN and control groups were used Weighted gene co-expression network analysis (WGCNA) for a subsequent analysis. The flowchart of our study is shown (Horvath and Dong, 2008) is an effective bioinformatics in Figure 1. approach for constructing a co-expression network based on gene expression data profile, which provides new insights for Weighted Gene Co-expression Network predicting co-expressed genes related to clinical traits and the Analysis pathogenesis of diseases. Differential gene expression analysis After filtering out genes with an average expression value of is a widely used and excellent bioinformatics method to detect less than 1, the top 75% of genes with the largest median changes in gene expression levels between different groups absolute deviation in the samples were selected to construct a co- (Segundo-Val and Sanz-Lozano, 2016). Thus, WGCNA and expression network using the R package “WGCNA” (Langfelder differential gene expression analysis were combined to screen key and Horvath, 2008). When 0.85 was used as the correlation genes related to the pathogenesis of tubulointerstitial lesions in coefficient threshold, the most appropriate soft-thresholding HN. We hypothesized that the identification of key genes would powers (b) value with the maximum average connectivity provide a new insight into HN biomarker discovery. was selected from 1 to 20. Then, the scale-free network was In the present study, datasets from Gene Expression Omnibus transformed into a topological overlap matrix (TOM) according (GEO) were adopted to establish a gene co-expression network to the most proper b. Based on the TOM, co-expression and to identify differentially expressed genes (DEGs) between modules were defined as branches of a hierarchical cluster HN tubulointerstitial tissues and matched controls. Then, the tree using the dynamic tree cut method with a minimum overlapping genes that are present in DEGs and the trait-related size cutoff of 50. To merge possible similar modules, we modules were used to construct a protein–protein interaction defined 0.2 as the threshold for cut height. Furthermore, (PPI) network to select hub genes. Meanwhile, Gene Ontology the adjacency of module eigengenes (Horvath and Dong, (GO) and Kyoto Encyclopedia of Genes and Genomes (KEGG) 2008) and the correlation among randomly picked genes analysis were performed to assess the potential functions of were calculated to evaluate the reliability of the constructed the overlapping genes. After GEO and clinical validation, a key modules. The results were visualized using heat maps. Next, gene was screened from hub genes. Finally, the key gene was the trait-related modules that were highly correlated with validated by in vivo experiments, and the potential biological clinical traits were selected by us for a subsequent analysis. functions of the key gene was investigated by gene set variation The gene significance (GS) and module membership (MM) of analysis (GSVA). trait-related modules were calculated and visualized using a scatter plot diagram. MATERIALS AND METHODS Differential Gene Expression Analysis Data Collection and Data Pre-processing and Identification of Overlapping Genes The DEGs between the HN and control samples were defined All HN and healthy control tubulointerstitial tissue samples using the “limma” R package (Ritchie et al., 2015). False discovery were selected from the GEO database1 with the GSE numbers rate (FDR) adjusted p-values < 0.05 and | log FC| > 0.5 in all four of GSE37455 (Berthier et al., 2012), GSE104954 (Grayson et al., comparisons were considered statistically significant. The PCA 2018), and GSE99325 (Shved et al., 2017). These datasets were of DEGs was applied to show the clustering of HN and control in accord with the following criteria: (1) containing both HN samples, and then the overlapping genes that are present in trait- and healthy control tubulointerstitial tissues, (2) including at related modules and DEGs were analyzed to identify potential least six HN tubulointerstitial samples, (3) the species was Homo hub genes. The differential expression of the overlapping genes sapiens, and (4) complete expression profiles were available. was visualized using a heat map. The gene expression profiles of these datasets were collected from tubulointerstitial compartments of kidney biopsies from Function Enrichment Analyses HN patients and healthy control donors (Table 1), whose clinical information is provided in Supplementary Tables 1–3, To further explore the biological significance of the overlapping respectively. More detailed clinical characteristics could genes, we used the R package “clusterprofiler” (Yu et al., 2012) to be found in the corresponding references (Table 1). Each conduct GO function and KEGG pathway enrichment analyses. < transcriptome array was normalized independently using Robust The cutoff value was set to p-value 0.05. Multiarray Average, followed by quantile normalization and log2 transformation. The removal of batch effects was performed by PPI Network Creation and Identification ComBat method, which is a widely used and highly effective of Hub Genes method, particularly with smaller sample sizes (Chen et al., 2011). In order to identify real hub genes, we first screened genes Principal component analysis (PCA) and relative log expression from the overlapping genes according to the criteria that
Details
-
File Typepdf
-
Upload Time-
-
Content LanguagesEnglish
-
Upload UserAnonymous/Not logged-in
-
File Pages15 Page
-
File Size-