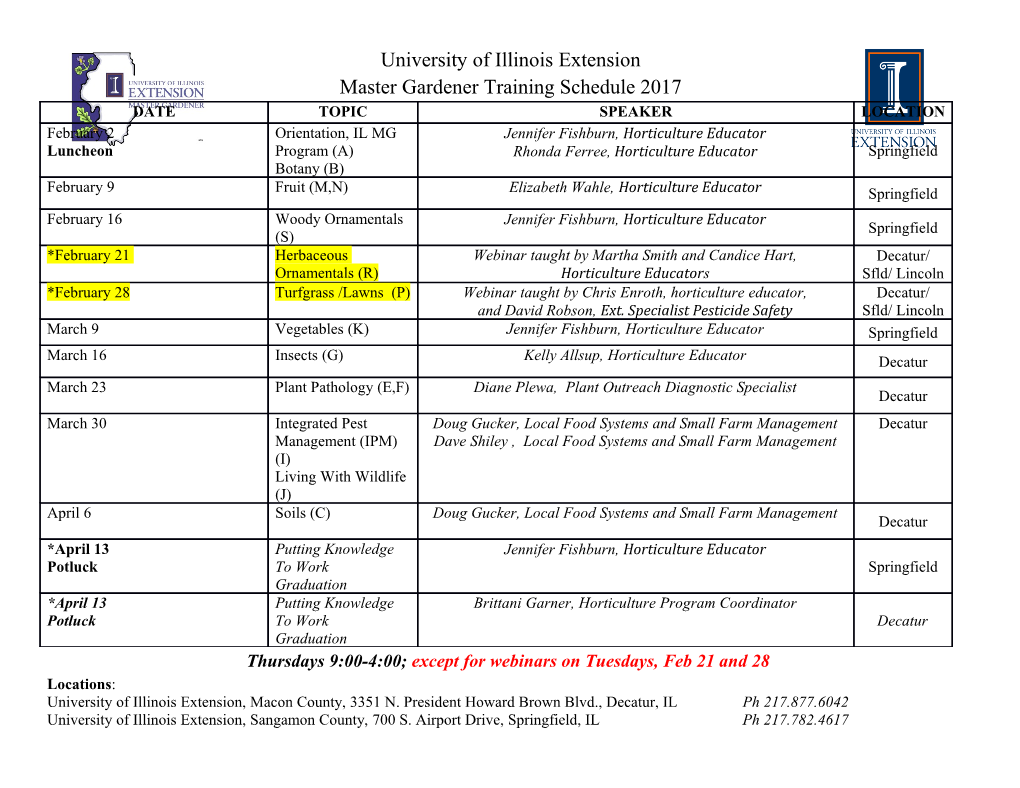
JOURNAL OF APPLIED MEASUREMENT, 10(2), Copyright© 2009 Local Independence and Residual Covariance: A Study of Olympic Figure Skating Ratings John M. Linacre University of the Sunshine Coast, Australia Rasch fit analysis has focused on tests of global fit and tests of the fit of individual parameter estimates. Critics have noted that slight, but pervasive, patterns of misfit to a Rasch model within the data may escape detection using these approaches. These patterns contradict the Rasch axiom of local independence, and so degrade measurement and may bias measures. Misfit to a Rasch model is captured in the observation residuals. Traces of pervasive, but faint, secondary dimensions within the observations may be identified using factor analytic techniques. To illustrate these techniques, the ratings awarded during the Pairs Figure Skating competition at the 2002 Winter Olympic Games are examined. The intention is to detect analytically the patterns of rater bias admitted publicly after the event. It is seen that the one-parameter-at-a-time fit statistics and differential item functioning approaches fail to detect the crucial misfit patterns. Factor analytic methods do. In fact, the competition was held in two stages. Factor analytic techniques already detect the rater bias after the first stage. This suggests that remedial rater retraining or other rater-related actions could be taken before the final ratings are collected. Requests for reprints should be sent to 2 LINACRE Introduction performers will fail on an item, and higher per- formers will succeed on it, then that item has high Local independence is a property required discrimination. In classical test theory (CTT), of measures. As Wright (1996) remarks, statisti- these are regarded as the best items. In Rasch cal independence in data occurs when the value theory, however, responses to such items may of one datum has no influence on the value of be seen to be too predictable from the responses another. Thus, the outcome of a “head” for a coin to the other items. This indicates that they lack toss does not increase the probability that the the local independence required for objective next toss produces a “tail”. Local independence measurement. Indeed, even in CTT, extreme over- specifies that the value of one datum has no influ- predictability leads to the “attenuation paradox” ence on another once the underlying variable, the in which increases in test reliability actually re- latent trait or dimension, has been accounted for duce test statistical validity (Loevinger, 1954) (conditioned out). Local independence includes, but goes beyond, unidimensionality. Including If there is an item with nothing in common the same math item twice in a math test would with the other items, it will tend to be entirely not alter its substantive dimensionality. Yet an unpredictable. It will indeed represent another examinee would be expected to either succeed dimension, but may be the only representative or fail on both items together, so responses to of that dimension. Such other dimensions include the two identical items would not be locally “misprints,” “miskeys,” “data entry errors” and independent. the like. Most commonly encountered Rasch quality-control fit statistics are designed to flag Successful implementation of Rasch mea- statistically unexpected responses or response surement requires items that approximate local patterns by considering the performance of one independence. The relationship between fit respondent or one test item at a time. These sta- statistics and dimensionality is not direct. Every tistics are very powerful for detecting guessing, item contains many dimensions. The intention of carelessness, response sets, social conformity, the test constructor is that, when a set of items miskeyed items, data entry errors and the like. is compiled into a test, what they share together These, however, are not what is usually meant by will be the dimension that the test constructor test multi-dimensionality. It cannot be determined intends, and, further, that particular dimension from one-item-at-a-time statistics whether the off- will overwhelm all the other little dimensional dimension behavior in an item is shared with other differences between the items. It can then be said items (i.e., forms another dimension) or is unique that what the items share is their “dimension”, and to each item. Even this approach is not hopeless, all the other dimensions in each item are acting because the analyst can often guessat the defini- like random noise. tion of shared secondary dimensions by looking Of course, this ideal is never realized in at the item content and response patterns. practice. Some items have more of what all items share, and some have less. This is basically what Dimensionality Detection item-level fit statistics are reporting. If there is What is required is a technique for discov- an item that only consists of material shared ering shared commonalities among some items with other items, then it will tend to be overly which are not explained by the shared dimension. predictable. An item of this type is the “overall In fact, local deviations from the model specifica- impression” item commonly printed at the end of tion of local independence “can be measured by customer satisfaction surveys. the size of residual covariances. Unfortunately, From a naive perspective, it would seem some computer programs for fitting the Rasch that the more highly predictable items in a test model do not give any information about these. are the best items. If it is fairly certain that lower A choice would be to examine the covariance matrix of the item residuals, not the sizes of the LOCA L INDEPENDENCE AND RESIDUA L COVARIANCE 3 residuals themselves, to see if the items are in- 2. For each observation, X, compute its expected deed conditionally uncorrelated, as required by value according to the measurement model, the principle of local independence” (McDonald, E, and the model variance of the observed 1985, p. 212). about expected Q. (For dichotomous data, Q In fact, not only does the covariance matrix is Jacob Bernoulli’s binomial variance). The of item residuals identify correlated items, it also part of the observation not directly explained facilitates the identification of patterns of shared by the measurement model, the residual, is correlations through factor analysis of the matrix. X-E. This is still in the raw score metric. This indicates which items are operating together 3. Normalize the residuals. Each residual indi- in an other-dimensional way, and how much this cates how much locally easier or harder that perturbs the variance structure in the data. item was than expected (or how much more or Not all multidimensionality is unintended less locally competent the examinee). These or unproductive. Papers which discuss other ap- residuals are anticipated to be the outcome proaches to the investigation of multidimension- of an infinity of infinitesimal perturbations ality, such as Wang, Wilson and Adams (1997) of the expected response, i.e., to follow a nor- and Chen and Davison (1996), are included in mal distribution. The model variance of that the reference list. distribution, for each response, is Q. So the standardized residuals, (X-E)/CQ, are mod- A Factor-analytic Approach eled to conform to an N(0,1) distribution. Smith and Miao (1994) demonstrate that 4. For each pair of items, compute the Pearson factor analysis is a useful method for identifying correlation between the standardized residu- multidimensionality in data that has been con- als across all examinees who responded to structed to be unidimensional. They analyze the both items. Potentially locally dependent matrix of raw responses, but this has the drawback pairs of items will have high positive cor- that the first factor only approximates the Rasch relations (e.g., items which embody the dimension. Indeed, multiple factors have been re- same perspective on a sub-dimension) or ported in data known to fit the Rasch model. “One high negative correlations (e.g., items which might expect the emergence of only one factor embody opposing perspectives). when a factor analysis would be performed on all 5. Perform a factor analysis of the item correla- newly defined subsets [of unidimensional items]. tion matrix. The first factor in the residuals, However, factor analysis of the newly defined reported here, is conceptually the second subsets yielded two factors. Further inspection factor overall, because the Rasch dimension of the factor plot showed that the emergence of is the first factor overall. This secondoverall a second factor could be considered as an artefact factor identifies the strongest shared pattern due to the skewness of the subset scores” (Van in local dependency among the items as der Ven and Ellis, 2000). reflected in their correlations. Subsequent This suggests an improved method of factor factors in the residuals may also be useful analysis in which the non-linearity and distri- diagnostically, but reflect weaker patterns butional aspects of the raw scores are removed in the data. prior to the investigation of multi-dimensionality If the data accord with the Rasch model, (Wright, 1996). i.e., the data exhibit local independence, the 1. Perform a conventional Rasch analysis. The standardized residuals for each item will load on resulting Rasch dimension is analogous to a an individual item-specific, statistically unique, first factor in a factor analysis, but now in a factor. There will be no commonality, and so no linear framework. second shared dimension. When this holds, the data are effectively unidimensional or perhaps en- tirely random. Divgi (1986) provides an example 4 LINACRE of randomness, the fitting of the Rasch model to components are orthogonal and unrotated. The coin tosses. In this case, the Rasch dimension has first PCAR component explains as much of the collapsed to a point. residual variance in the data as possible.
Details
-
File Typepdf
-
Upload Time-
-
Content LanguagesEnglish
-
Upload UserAnonymous/Not logged-in
-
File Pages13 Page
-
File Size-