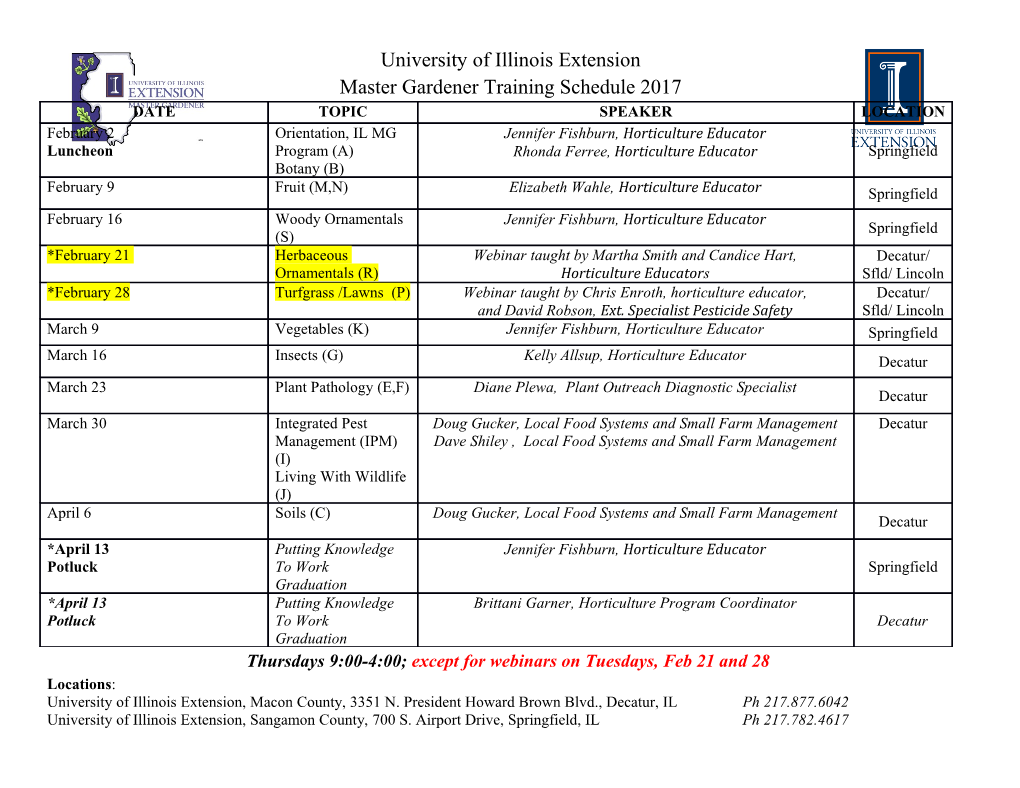
8/30/16 Unit 1 Outline • Variables & Measurement • Collecting Data Unit 1: Data Collection • Sampling Section 1.1 & 1.2 in the Text • Random Assignment (for causal inference) In God we trust. All others must bring data. – W. Edmunds Deming 2 Variables and their Measurements Categorical Variables Variable: Any characteristic that takes different values for • • Two types: Nominal and Ordinal different individuals in a sample or population • Nominal variable: a categorical variable in which the Two major Types of variables: categorical and quantitative • categories are unordered. A categorical variable is a variable that can take on a few • • Ordinal variable: a categorical variable in which the different values (categories) when measured. Sometimes categories have an order or hierarchy (and can possibly be called qualitative variables. numeric), but there is “no defined distance between levels • A quantitative variable is a variable that is measured on a on the measurement scale” numerical scale covering a large range of values. • Examples? • Examples? Categorical: Nominal: Quantitative: Ordinal: 3 4 1 8/30/16 1 + 1 = 3 What a Dummy! In English Dummy Variables Quantitative Variables please? X Y • Two types: Discrete and Continuous. • There is a special type of “nominal” categorical variable • Both are measured on an interval scale. That is called a dummy variable or indicator variable. there is a specific numerical distance between any two measurements. • These variables take on only 2 possible values: 0 or 1. The one usually stands for success or yes, while the zero usually • Discrete variable: a quantitative variable that can only take on stand for failure or no. specific numbers, like 0, 1, 2, … • By convention, they are usually named after the category • Continuous variable: a quantitative variable that can take an that is a success. infinite number of possibilities within a range of numbers • Example: to represent sex/gender, we could define a • Examples: dummy variable named female, which would be 1 for all Discrete: women, and 0 for all men: ⎧1 if female Continuous: female = ⎨ ⎩0 if male 5 6 Summary of types of Variables: Unit 1 Outline Va r i a b l e s • Variables & Measurement Categorical Quantitative • Collecting Data Nominal Ordinal Discrete Continuous (more common) (less common) • Sampling • Random Assignment (for causal inference) Dummy (special case) **Note: in this class (and most of statistics), the most important difference is that between categorical and quantitative variables. That differentiation will typically determine the type of statistics and analysis used. Nominal and ordinal variables are often treated the same. Same for discrete and continuous variables. 7 8 2 8/30/16 Anecdotal evidence Collecting Data • Anecdotal evidence is based on haphazardly selected • Data can be collected in many ways: individual cases, that often come to our attention because they are striking (probably not representative) • Example: Politicians often cite the case of a single The further individual to invoke a public response consistent with 1. Anecdotal information down the list you go, the the politicians’ desire (a sample of size n = 1) 2. Available data more reliable • “Ask for averages, not testimonials” the information is. And the 3. Observational studies conclusions you can draw will 4. Randomized experiments typically then be stronger. 9 10 Available data Observational Studies • Available data are data that were produced in the past for some other purpose but may help answer a present question • An observational study is one in which data is collected by merely observing the measurements on the individuals in • Many use available data because producing new data is the sample. No attempt to influence or intervene with the expensive (nearly always most costly part of research). subject is taken. • There are lots of reliable available datasets on the web rich • May be difficult to reach causal conclusions (that changing with information. Some examples: one variable causes another variable to change) since other : http://www.census.gov/# variables may be muddling up (called confounding) this relationship. : http://www3.norc.org/gss+website/ • Example: Does smoking cigarette increase your risk of heart disease? • Example: Let’s come up with a survey of Harvard: : http://www.hcup-us.ahrq.gov/nisoverview.jsp 11 12 3 8/30/16 Observational Studies Observational Studies Pros: Cons: • Usually cheap • Establishing causation may be impossible due to the presence of confounding variables. • The only option when randomized experiment is not • Requires advanced statistical methods and unverifiable feasible or unethical assumptions. • Showing causation is not always necessary • Risk factors for medical decisions, population statistics. • Confounding variable (or factor), sometimes referred to as a confounder or a lurking variable, affects both the group • Risk factor (common in medicine and epidemiology) - membership and the outcome (or dependent) variable. a variable associated with an increased risk of disease or infection. • This third variable causes the two variables to falsely appear to be • Examples? related. 13 14 Confounding Variables Experiments • An experiment is a study in which an investigator imposes an • Name confounding variables that may induce the following intervention (e.g. treatment) on individuals in order to associations: observe their response. • Clinical trials are a type of experiment • The association between the amount of serious crime committed and the amount of ice cream sold by street • An Example: A comparison of different drugs for women vendors. with breast cancer, often with as few as 100 people. • The experimenter chooses women in the study receive the • Drink More Diet Soda, Gain More Weight?: Overweight different levels of the drug (new therapy vs. old therapy). Risk Soars 41% With Each Daily Can of Diet Soft Drink. The levels of the drug are called the treatment. • The outcome of the study may be the measured amount of • Negative correlation between a size of one’s palm and their disease-free survival for each woman life expectancy. 15 16 4 8/30/16 Experiments: a few details Experiments • There has to always be at least two groups of the treatment to • An experiment is the best (only?) way to determine if one compare. The ‘default’ condition is often called the control group variable (the treatment) causes another variable (the outcome) (standard-of-care in clinical trials). to vary. • The control group may receive a placebo treatment. This is a • However, they are not always ethical or plausible. You treatment that looks like the active treatment (classic ex: a cannot knowingly do harm to human subjects by forcing ‘sugar pill’) them to take a dangerous treatment (ex: force to smoke) • The subjects should be randomized to the treatment groups. That is, chance should decide which patients receive the treatments • Experiments may not mimic real life (the conditions in which an experiment is run are often too ‘perfect’ or unrealistic). • This guarantees that all other variables are balanced across the So there is often some loss of generalization of them to the treatment groups real world. • To ensure this balance, the study needs to be replicated enough times. • They are also the most expensive way to collect data 17 18 Confounding Variables and Unit 1 Outline Randomization • Suppose we would like to compare two methods of teaching • Variables & Measurement introductory statistics. • At Harvard, one professor uses standard lecturing set-up in his • Collecting Data class and another professor uses an interactive clicker approach in her class. • Sampling • Students in the two classes are given achievement tests to see • Random Assignment (for causal inference) how well they learned the tests. • Confounding variables? • Better experiment? 19 20 5 8/30/16 Population vs. Sample Parameters and Statistics • Population: entire group of individuals on which we desire information. • Parameter (often called an estimand): a numerical summary of the population (like µ or p). • Technicality: actual vs. conceptual populations • For our Harvard study: • For our Harvard study: • Statistic: a numerical summary of the sample data. • For our Harvard study: • Estimator: a statistic used as a guess for the value of the • Sample: a part of the population on which we estimand ( or pˆ ). actually collect data. • Estimate: a particular realization of the estimator (4/12 • For our Harvard study: = 0.33). 21 22 Selection of Study Units Parameter vs. Estimate Study/experimental unit/subject - one member of a set of Parameter (also, estimand) - Estimate - proportion of proportion of childless entities being studied. childless households in households in the population. Two ex tremes o f a sel ectio n the sample. mechanism: • Self-selection (volunteers, haphazard) • Random sampling Analysis, Estimates, & Inference - childless household - household with children under 18 Purpose: describe population characteristics. 23 24 6 8/30/16 Entire population = all possible units Target Population: a collection of units a researcher is interested in; a group about which the researcher wishes to draw conclusions. 25 26 Census: sample everybody in target population Census Pros: • In principle, no need to use statistical inference Cons: • Expensive • Long and difficult • In practice, never perfect: • Respondents are often not representative of target population! 27 28 7 8/30/16 Sampling units whose data Collection of units that are
Details
-
File Typepdf
-
Upload Time-
-
Content LanguagesEnglish
-
Upload UserAnonymous/Not logged-in
-
File Pages12 Page
-
File Size-