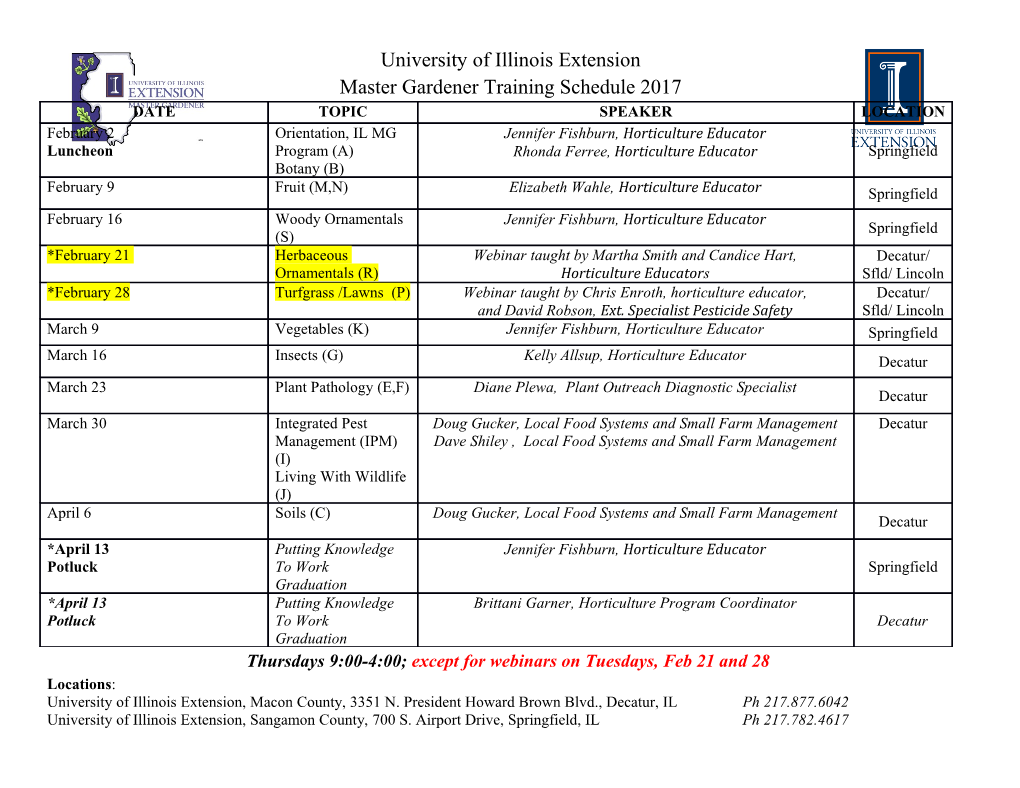
American Journal of Bioinformatics 1 (1): 20-29, 2012 ISSN 1948-9862 © 2012 Science Publications Bayesian Determination of Disease Associated Differences in Haplotype Blocks 1Ivan Kozyryev and 2Jing Zhang 1Department of Physics, Faculty of Arts and Sciences, Harvard University, Cambridge, MA, USA 2Department of Statistics, Faculty of Arts and Sciences, Yale University, New Haven, CT, USA Abstract: Problem statement: While experimental ascertainment of haplotype blocks in the genome- scale case-control studies is expensive, accurate computational phasing is still a daunting task for bioinformatics approaches. We used a statistical method to determine differences, potentially associated with a certain disease, in linkage disequilibrium block boundaries in whole-genome Single Nucleotide Polymorphisms (SNPs) data. Approach: We utilized a Bayesian model for calculating the posterior probabilities of the block boundaries in the SNPs data and used Metropolis-Hastings algorithm to sample from that posterior distribution. Our method was applied to search for haplotype- block boundary differences associated with two autoimmune diseases: Type I Diabetes (T1D) and Rheumatoid Arthritis (RA). Results: We located the regions on chromosome 6 with significant control-case difference in haplotype blocks around the SNPs and genes that were previously known to be associated with T1D and RA (in the HLA complex), as well as around genes whose association with the autoimmune diseases should be further explored in future studies. Conclusion/Recommendations: The statistical approach explored in this study provides an efficient and accurate way to study connection of haplotype-block differences to multiple important diseases. Key words: Linkage disequilibrium, autoimmune diseases, HLA complex, type 1 diabetes, rheumatoid arthritis INTRODUCTION be thought of as “tiny typos” in the genome with one base being replaced by another. While some SNPs Statistical approaches in genetics: Even though it has directly contribute to the disease, others can be linked been a decade since researchers first sequenced the to the genes that do (Carmichael, 2010). However, human genome, obvious links between the genes and complications arise when we try to analyze such data, specific diseases have been much slower to appear than due to the fact that the number of possible interaction originally expected by everybody. Because original combinations among genotype markers is humongous approach based on simple correlation analysis was not for a large size genetic association study and we are successful, now many researchers believe that new interested in finding very few disease-related advances in genomics will come from a rich interactions (Zhang and Liu, 2007). Additionally, some statistical understanding of complex interactions of nearby SNPs are highly correlated due to linkage our genetic code (Bansal et al ., 2010; Zhang and Liu, disequilibrium (Zhang et al ., 2011b), which further 2007; Svoboda, 2010). However, it is necessary to complicates statistical analysis aiming at determining perform statistical analysis on a vast amount of data disease related interactions. consisting out of the sequences including millions of Importance of linkage disequilibrium: Linkage genomes in order to completely understand how our Disequilibrium (LD) describes the phenomenon when genetic code interacts with the environment to make the genotypes at nearby markers are highly correlated us the way we are (Svoboda, 2010). (Zhang et al ., 2011a). This correlation arises due to Whole-genome Single Nucleotide Polymorphisms shared ancestry of contemporary chromosomes (The (SNPs) data from individuals in the case-control studies International HapMap Consortium, 2005). LD patterns has potential to help us understand complex interactions have many important applications in biology and among multiple genes (Zhang et al ., 2011a). SNPs can genetics. These patterns can be used for inferring the Corresponding Author: Jing Zhang, Department of Statistics, Faculty of Arts and Sciences, Yale University, New Haven, CT, USA 20 Am. J. of Bioinformatics 1 (1): 20-29, 2012 distribution of cross-over events at short scales which descriptions of RA and T1D genetic landscapes are still are hard to study experimentally, studying gene far from being understood (Coenen and Gregersen, conversion, about which there is only a very limited 2009; WTCCC, 2007). We chose to focus our study on amount of experimental data, understanding the RA and T1D at the same time because they are already evolutionary history of humans and detecting natural known to share common loci and are both autoimmune selection (Wall and Pritchard, 2003). diseases (WTCCC, 2007). Patterns of linkage disequilibrium are unpredictable and very noisy (Wall and Pritchard, 2003). Additionally, Goals of the project: The goal of this project was extent of LD defers from one genomic region to using as a starting point a highly successful approach to another. Population history, fine-scale heterogeneity in classification problems with discrete covariates (Zhang et recombination rates and population genetic models all al ., 2011b) specifically used for determining block-based contribute to noisy appearance of spatial structure of epistasis associations, to develop a statistical model to LD. However, this complex reality is described by a search for disease associated differences in LD-block simple model known as haplotype-block model (Wall structure between control and case groups. We and Pritchard, 2003). According to this model, determined haplotype-block structure for controls and genotype data is divided into discrete blocks with cases independently and used this information to find highly correlated SNPs within each block and regions with genetic variants that could potentially be adjacent blocks are separated by recombination associated with the disease. Our methods were applied to events (Zhang et al ., 2011b; 2004; Ding et al ., the actual large data sets consisting of whole-genome 2005a; 2005b). Even though described model is single nucleotide polymorphisms data from chromosome simple, experimental data confirms its validity (Wall 6 (chr6) for T1D and RA patients and control groups. and Pritchard, 2003; The International HapMap Consortium, 2005). MATERIALS AND METHODS Background on type 1 diabetes and rheumatoid Our problem of using haplotype-block structure for arthritis: Type 1 Diabetes (T1D) affects 0.5% of the whole-genome chr6 SNPs data from patients and world population and 1.4 million US people (Bottini et controls to determine regions of genetic variants that al ., 2004). It is a chronic disease that occurs when not increase susceptibility to the disease can be divided into enough insulin is produces to control the sugar levels in two smaller sub-problems. First, since there is no blood. It can occur at any age but most frequently it is complete experimental data on spatial structure of LD diagnosed in children and young adults (Devendra et for our regions of interest, we determined LD blocks for al ., 2004). Even though the exact cause of the disease is controls and cases using computational statistical unknown, most researchers suspect that there is some methods and then extracted the disease relevant sort of trigger (environmental or viral) that causes an information hidden in the background noise. Each of immune reaction in genetically susceptible group of these steps used different modeling and data analysis people. As of right now, no cure is available and the techniques that are described in detail below. outcome for people with this type of diabetes varies (Devendra et al ., 2004). Previous studies have validated Statistical model for determining block boundaries the hypothesis that common genetic variants play due to linkage disequilibrium: The observations important role in the disease formation (Bottini et al ., consist of genotypes of L SNP markers observed on N 2004; Polychronakos and Li, 2011; Zhang et al ., patients, combined into final data set D. Each marker 2011a). can have one of three values. The goal is to partition Like T1D, Rheumatoid Arthritis (RA) has a L markers into blocks in such a way that the significant genetic contribution to its development but correlations between SNPs in different blocks are the detailed heritability is still not known (Newton et close to zero, yet the number of observed genotype al ., 2004). RA is a chronic disease that is accompanied combinations of SNPs within each block is small. by severe pain that arises from destruction of the Below we describe a method that uses a Bayesian synovial joints (WTCCC, 2007). Even though recent model and a Monte Carlo algorithm to determine advances in the Genome-Wide Association Studies block structure for the given SNPs data set. (GWAS) are starting to show the directions for new therapies and advancement of understanding the General overview: Let B be the block partition complex relationships between different autoimmune variable which has a form of L binary indicators where disorders and their genetic causes, complete value of one (1) corresponds to the start of the next 21 Am. J. of Bioinformatics 1 (1): 20-29, 2012 block. Using multiplication law for conditional particular diplotype. We model the diplotype counts by probability, we can calculate combined probability of the multinomial distribution with its frequency the Data (D) and Block structure (B) to be Eq. 1: parameters following a Dirichlet prior distribution: P∼ b-s Dirichlet(a 1,a 2,…,a 3 ), where Dirichlet density P(D,B) = P(B | D)P(D). (1) function is given by (let K = 3 b-s) Eq. 6: Therefore, we find the posterior probability of the 1 K ai -1 block boundaries given by Eq. 2: f(p,a) =∏ p i (6) B(a) i=1 P(D,B) P(B | D) = (2) P(D) and the normalizing constant B(a) is specified by K ∏ Γ(a ) i=1 i where Γ is the gamma function and a is the Let's consider numerator and denominator of the K Γ(∑ ai ) above equation separately. Using multiplication law i=1 ones more, we can express the numerator as Eq. 3: vector of Dirichlet parameters. Let ni denote the number of counts of a specific diplotype i out of K in the block P(D,B) = P(D | B)P(B) (3) under consideration.
Details
-
File Typepdf
-
Upload Time-
-
Content LanguagesEnglish
-
Upload UserAnonymous/Not logged-in
-
File Pages10 Page
-
File Size-