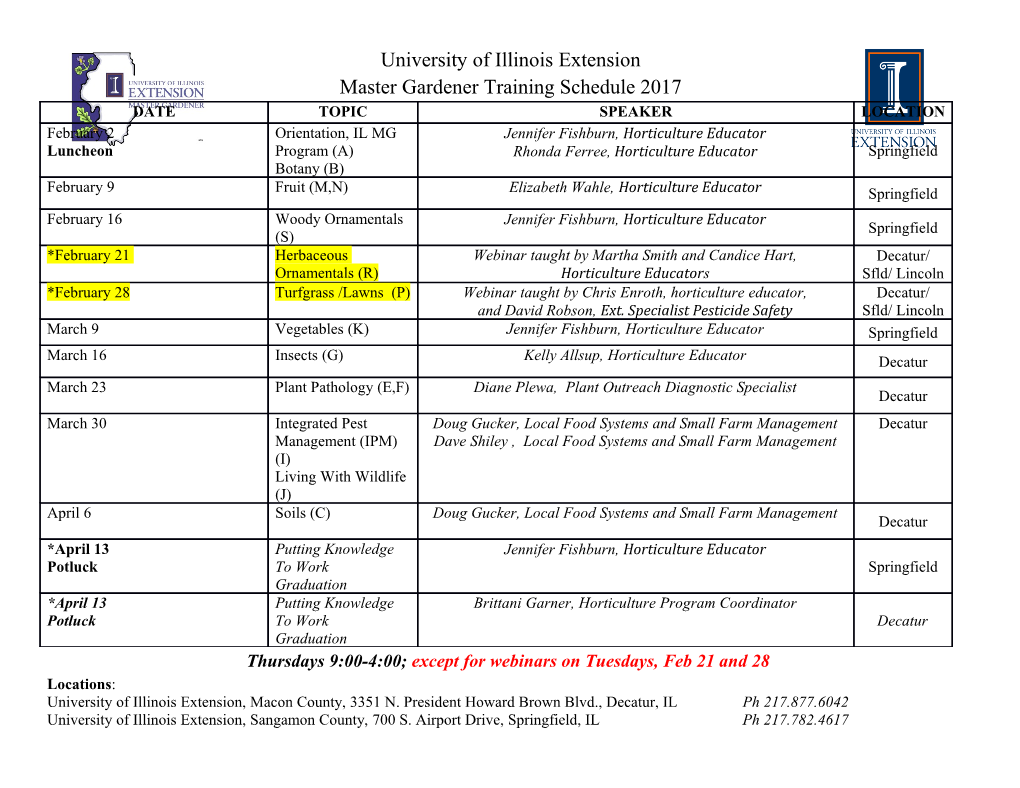
Edlin: an easy to read linear learning framework Kuzman Ganchev ∗ Georgi Georgiev University of Pennsylvania Ontotext AD 3330 Walnut St, Philaldelphia PA 135 Tsarigradsko Ch., Sofia , Bulgaria [email protected] [email protected] Abstract main advantage of Edlin is that its code is easy The Edlin toolkit provides a machine to read, understand and modify, meaning that learning framework for linear models, variations are easy to experiment with. For in- designed to be easy to read and un- dustrial users, the simplicity of the code as well derstand. The main goal is to provide as relatively few dependencies means that it is easy to edit working examples of im- easier to integrate into existing codebases. plementations for popular learning algo- Edlin implements learning algorithms for rithms. The toolkit consists of 27 Java classes with a total of about 1400 lines linear models. Currently implemented are: of code, of which about 25% are I/O and Naive Bayes, maximum entropy models, the driver classes for examples. A version Perceptron and one-best MIRA (optionally of Edlin has been integrated as a pro- with averaging), AdaBoost, structured Percep- cessing resource for the GATE architec- tron and structured one-best MIRA (option- ture, and has been used for gene tagging, ally with averaging) and conditional random gene name normalization, named entity fields. Because of the focus on clarity and con- recognition in Bulgarian and biomedical ciseness, some optimizations that would make relation extraction. the code harder to read have not been made. This makes the framework slightly slower than it could be, but implementations are asymp- Keywords totically fast and suitable for use on medium Information Extraction, Classification, Software Tools to large datasets. The rest of this paper is organized as fol- lows: 2 describes the code organization; 3- 4 1 Introduction describes§ an integration with the GATE frame-§ § work and an example application; 5 describes The Edlin toolkit provides a machine learn- related software; and 6 discusses§ future work ing framework for linear models, designed to and concludes the paper.§ be easy to read and understand. The main goal is to provide easy to edit working exam- ples of implementations for popular learning 2 Overview of the code algorithms. To minimize programmer over- head, Edlin depends only on GNU Trove1 for The goal of machine learning is to choose from fast data structures and JUnit2 for unit tests. a (possibly infinite) set of functions mapping A version of Edlin has been integrated as a from some input space to some output space. processing resource for the GATE [7] architec- Let x X be a variable denoting an input ex- ample∈ and y Y range over possible labels for ture, and has been used in-house for gene tag- ∈ ging, gene name normalization, named entity x. A linear model will choose a label according recognition in Bulgarian and biomedical rela- to tion extraction. For researchers we expect the h(x) = arg max f(x, y) w (1) y · ∗Supported by ARO MURI SUBTLE W911NF-07- where f(x, y) is a feature function and w is a 1-0216 and by the European projects AsIsKnown (FP6- 028044) and LTfLL (FP7-212578) parameter vector. We take the inner product 1 http://trove4j.sourceforge.net/ of the feature vector with the model parame- 2 http://www.junit.org ters w and select the output y that has high- 94 International Conference RANLP 2009 - Borovets, Bulgaria, pages 94–98 est such score. The feature function f(x, y) is sentences are read from disk and converted to specified by the user, while the parameter vec- a sparse vector representing f1(x) by a class tor w is learned using training data. in the io package. For example we might ex- Even though the learning and inference algo- tract suffixes of length 2 to 5 from each word rithms are generic, and can be used for differ- in a sentence. We look these up in an al- ent applications, Edlin is implemented with an phabet that maps them to a unique dimen- natural language tasks in mind. The classes sion, and store the counts of the words in a related to classification, are implemented in sparse vector for each word. The alphabet and the classification package, while those re- sparse vector are implemented in Alphabet lated to sequence tasks are implemented in the and SparseVector respectively. The array sequence package. The code to perform gra- of sparse vectors for a sentence (recall there dient ascent and conjugate gradient is in an is one for each word) and alphabet are bun- algo package. There are three helper pack- dled together in an SequenceInstance object ages. Two (experiments and io) are code for along with the true label. Next we want to reading input and for driver classes for the ex- train a linear sequence model using the percep- amples. The final package, called types con- tron algorithm on the training portion of our tains infrastructure code such as an implemen- data. We construct a sequence.Perceptron tation of sparse vectors, elementary arithmetic object and call its batchTrain method. Fig- operations such as the inner product, and other ure 1 reproduces the implementation. The widely used operations whose implementation method takes the training data as a Java is not interesting from the point of view of un- ArrayList of SequenceInstance objects, and derstanding the learning algorithms. This code the Perceptron class has parameters for organization, as well as the data structures we whether averaging is turned on and the num- employ are similar to other learning packages ber of passes to make over the data. It also such as StructLearn [12] and MALLET [11]. contains a SequenceFeatureFunction object One attribute that distinguishes Edlin from (fxy in Figure 1) that implements f2 from both of these packages is the decomposition of above. For part of speech tagging, it is typ- (t 1) (t) the feature function into ical to let ft(x, y − , y ) conjoin f1(x) with (t) (t) (t 1) y and also conjoin y with y − , but not f(x, y) = f2(f1(x), y) (2) to have any features that look at x, y(t) and (t 1) where f maps the input into a sparse vector y − all at the same time. By contrast 1 for named entities it is typical to have fea- and f2 combines it with a possible output in or- der to generate the final sparse vector used to tures that look at all three. The linear se- assess the compatibility of the label for this in- quence model is created in the first line of the put. By contrast, many other learning frame- batchTrain method as a LinearTagger ob- ject, which has access to the alphabet used works only allow the user to specify f1 and hard-code an implementation of f as conjoin- in the initial construction of the sparse vec- 2 tors, the label alphabet (yAlphabet in Figure ing the input predicates (f1 in the notation above) with the label. By allowing the user 1) and f2 (fxy in Figure 1). It computes the prediction which is represented as an int array, to specify f2, we allow them to tie parameters and add domain information about how differ- with the interpretation yhat[t]=j as word t ent outputs are related. See the illustration has the label at position j in the label alphabet below for an example. (accessible via yAlphabet.lookupIndex(j)). The batchTrain method returns the linear se- quence model. 2.1 Example Application Perhaps the best way to describe the minimal amount to make reading the code easy is to 3 GATE integration trace how information is propagated and trans- formed in an example application. Take a POS GATE [8, 7] is a framework for engineering tagging task as an example. Suppose we are NLP applications along with a graphical de- given a collection of sentences that have been velopment environment for developing compo- manually annotated and these have been split nents. GATE divides language processing re- for us into a training set and a testing set. The sources into language resources, processing re- 95 public LinearTagger batchTrain( both Edlin and several GATE processing com- ArrayList<SequenceInstance> trainingData) { LinearTagger w = new LinearTagger(xAlphabet, ponents. The results are described in [9]. yAlphabet, fxy); Following BioNLP terminology, we use the LinearTagger theta = null; if (performAveraging) term proteins to refer to both genes and gene theta = new LinearTagger(xAlphabet, yAlphabet, products. Both trigger chunks and proteins are fxy); for (int iter = 0; iter < numIterations; iter++) { called participants. For example, the text “... for (SequenceInstance inst : trainingData) { phosphorylation of TRAF2 ...” would be a re- int[] yhat = w.label(inst.x); // if y = yhat then this update won’t change w. lation of type Phosphorylation with a theme of StaticUtils.plusEquals(w.w, fxy.apply(inst.x, TRAF2. The relation is called an event, while inst.y)); StaticUtils.plusEquals(w.w, fxy.apply(inst.x, the string “phosphorylation” is called a trig- yhat), -1); ger. Gene boundary annotations are provided if (performAveraging) StaticUtils.plusEquals(theta.w, w.w, 1); by the task organizers. In general, there are } events with multiple participants in addition to } if (performAveraging) return theta; the trigger. The event instances are organized return w; into the structure of the Gene Ontology [5]. } We separated the task in two main sub-tasks Fig. 1: Edlin’s perceptron implementation, re- (i) recognition of trigger chunks using an Edlin produced verbatim to show code organization. sequence tagger and, (ii) classification of trig- gers and proteins as either forming an event from one of 9 predefined types or not partici- sources, and graphical interfaces.
Details
-
File Typepdf
-
Upload Time-
-
Content LanguagesEnglish
-
Upload UserAnonymous/Not logged-in
-
File Pages5 Page
-
File Size-