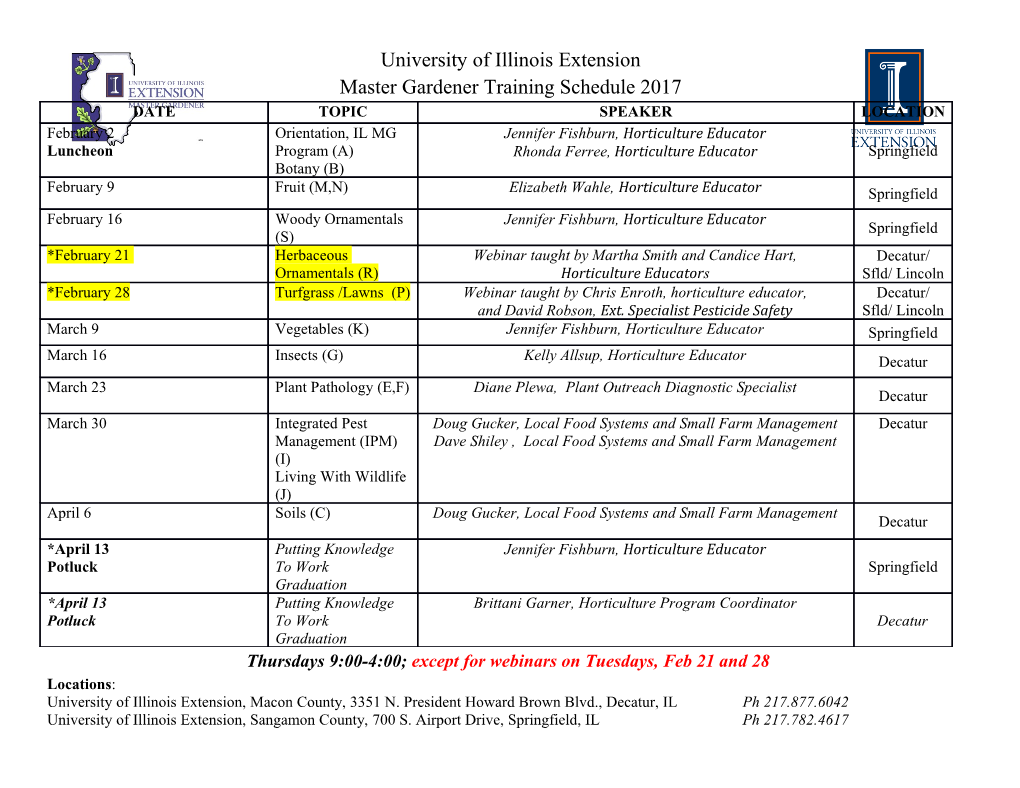
Ask the Crowd to Find Out What’s Important Sisay Fissaha Adafre Maarten de Rijke School of Computing, Dublin City University ISLA, University of Amsterdam [email protected] [email protected] Abstract Rita Grande Rita Grande (born March 23, 1975 in Naples, Italy) is a profes- We present a corpus-based method for estimating the im- sional female tennis player from Italy. portance of sentences. Our main contribution is two-fold. WTA Tour titles (8) First, we introduce the idea of using the increasing amount • Singles (3) of manually labeled category information (that is becoming available through collaborative knowledge creation efforts) – 2001: Hobart, Australia to identify “typical information” for categories of entities. – 2001: Bratislava, Slovakia Second, we provide multiple types of empirical evidence for – 2003: Casablanca, Morocco the usefulness of this notion of typical-information-for-a- • Singles finalist (1) category for estimating the importance of sentences. – 1999: Hobart (lost to Chanda Rubin) • Doubles (5) 1 Introduction – 1999: ’s-Hertogenbosch (with Silvia Farina Elia) Estimating the importance of a sentence forms the core – 2000: Hobart (with Emilie Loit) of a number of applications, including summarization, – 2000: Palermo (with Silvia Farina Elia) question answering, information retrieval, and topic detec- – 2001: Auckland (wth Alexandra Fusai) tion and tracking. Existing approaches to importance esti- – 2002: Hobart (with Tathiana Garbin) mation use different structural and content features of the Categories: 1975 births — Italian tennis players textual unit, such as position of a sentence in a document, word overlap with section headings, and lexical features Figure 1. Sample Wikipedia article such as named entities or keywords that are characteristic of the document [7, 15, 16, 1]. We investigate a corpus- based method for estimating the importance of information (such as date and place of birth) but not all (e.g., there is for a given entity. Particularly, we propose to exploit the nothing on education or marital status). On the other hand growing amount of manually labeled category information (as with other tennis players), the article contains a list of that is becoming available in the form of user-generated tournaments she has won in her career. content (such as Wikipedia articles, or tags used to label In this paper we use Wikipedia, with its rich category 1 blog posts) and/or through crowd-sourcing initiatives (such information as our starting point to explore the following as the ESP game for labeling images, [19]). Given an en- proposition: information that is typically expressed in the tity of some category, we consider other entities of the same descriptions of entities belonging to some category is infor- category and the properties that are typically described for mation that is important for entities in that category. We test them. That is, if a property is included in the descriptions of the viability of this proposition in a number of steps: a significant portion of entities in the same category as our 1. First, we determine whether there is a low divergence input entity, we assume it to be an important one. between descriptions of entities that belong to the same To make things more concrete, let us look at an example. category—lower than beween descriptions of entities In Figure 1 we display a sample Wikipedia article, on Rita that belong to different categories. Grande, an Italian tennis player. The category information for this article is at the bottom of the article (“1975 births” 2. Second, and building on a positive outcome for the and “Italian tennis players”). A few things are worth noting: 1We used the XML version of the English Wikipedia corpora made compared to descriptions of other people (not just tennis available by [4]. It contains 659,388 articles, and 2.28 categories per arti- players), the article has some typical biographical details cle, on average. first step, we test whether typical-information-for-a- frequency. More advanced techniques define importance in category coincides with important information for en- a centroid-based manner: the most central sentences in a tities in a category. We examine this issue using document (or cluster of documents) are the ones that con- Wikipedia itself, relating typical information to doc- tain more words from the centroid of the cluster [14]. More ument structure and writing style. recently, centrality-based methods have been used, whose 3. Third, we test if typical-information-for-a-category estimation relies on the concept of centrality in a cluster of coincides with important information for entities in documents viewed as a network of sentences [5]. another setting, viz. the TREC Question Answering We assume that information that is shared by multiple track; here we use typical-information-for-a-category entities of the same category is likely to be important to en- to distinguish between “vital or okay” sentences on the tities of that category. This assumption is an extension of the one hand, and “not okay” sentences on the other. idea that languages used in a specific domain are more con- strained than the general language [6]. These contraints are 4. In our fourth and final step, we take the runs sub- realized in the syntactic structure and word co-occurence mitted for the “other” questions as part of the TREC patterns in these structures. Furthermore, texts from the 2005 Question Answering track, and use our “typical- same domain have also been shown to exhibit a certain de- information-is-important-information” strategy to fil- gree of content overlap [21]. Concretely, the descriptions ter out non-important snippets. of entities of a given category in Wikipedia typically con- Our main contribution is two-fold. We introduce the idea sist of idiosyncratic information peculiar to each individual of using the increasing amount of manually labeled cate- entity, and instances of a general class of properties appli- gory information to identify “typical information” for cate- cable to all entities in the category—the properties we ex- gories of entities. And we provide multiple types of empir- ploit. In order to test the validity of our assumption under ical evidence for the usefulness of typical-information-for- different similarity measures, we apply different algorithms a-category for estimating the importance of sentences. (KL-divergence and cosine similarity). The remainder of the paper is organized as follows. In the next section, we provide background information on 3 Is There Any Typical Information? importance estimation and Wikipedia related work in lan- Before we can make use of information that is “typical” guage technology. Section 3 provides empirical results of for entities of some category, we need to establish the fact the within category similarity experiments (Step 1). Then, that such typical information exists. Working on the En- in Section 4 we relate “typical” information to “important” glish Wikipedia (see footnote 1), we proceed as follows: we information within the setting of Wikipedia itself (Step 2). compare the content of Wikipedia articles of entities within In Section 5 we do the same thing, but in the setting of the a single given category against Wikipedia articles outside TREC QA track (Step 3), and in Section 6 we detail our re- this given category. We take a set of categories and compare ranking experiments (Step 4). We conclude in Section 7. their word distributions with word distributions of a corpus constructed from a set of randomly selected Wikipedia arti- 2 Background cles. Our aim, then, is to find out whether articles within a Wikipedia has attracted much interest from researchers category look more like each other than like articles outside in various disciplines. While some study different aspects their category. To this end, we take the following steps: of Wikipedia itself, including information quality, motiva- tion of users, patterns of collaboration, network structures, • Select a random sample of C Wikipedia categories. underlying technology, e.g., [22]. Others are interested in • From each category Ci, take a random sample of N applying its content to solve research problems in different articles, which we call the category list. domains, e.g., question answering and other types of infor- • From each category Ci, take a random sample of n mation retrieval [11, 13, 9]. Wikipedia has also been used (n < N) articles, called the sample list (Si); we re- for computing semantic word relations, named-entity dis- move these articles from the category list. ambiguation, text classification and other related activities, • Take a random sample R of articles from the whole and as a document collection for assessing solutions to vari- Wikipedia; this yields the random list. ous retrieval and knowledge representation tasks, including • Construct language models using C s, S s, and R. INEX, and WiQA (CLEF 2006) Contest [3, 17, 4, 8]. i i Our interest in this paper is very specific: the task of es- • For each category, compute the KL-divergences timating the importance of a sentence. Typically, sentence between Cis and Sis (the “within-category” KL- importance is modelled in terms of the importance of the divergence), and between the Cis and R (the “outside- constituting words. A commonly used measure to assess the category” KL-divergence). importance of words in a sentence is the inverse document • Plot the resulting values. Within category Across category 12 10 8 6 KL−divergence 4 2 0 1 2 3 4 5 6 7 8 9 10 11 12 13 14 15 16 17 18 19 20 21 22 23 24 25 26 27 28 29 30 31 32 33 34 35 36 37 38 39 40 41 42 43 44 45 46 47 48 49 50 51 52 53 54 55 56 57 58 59 60 61 62 63 64 65 66 67 68 69 70 71 72 73 74 75 76 77 78 79 80 81 82 83 84 85 86 87 88 89 90 91 92 93 94 95 96 97 98 99 Topic Figure 2.
Details
-
File Typepdf
-
Upload Time-
-
Content LanguagesEnglish
-
Upload UserAnonymous/Not logged-in
-
File Pages6 Page
-
File Size-