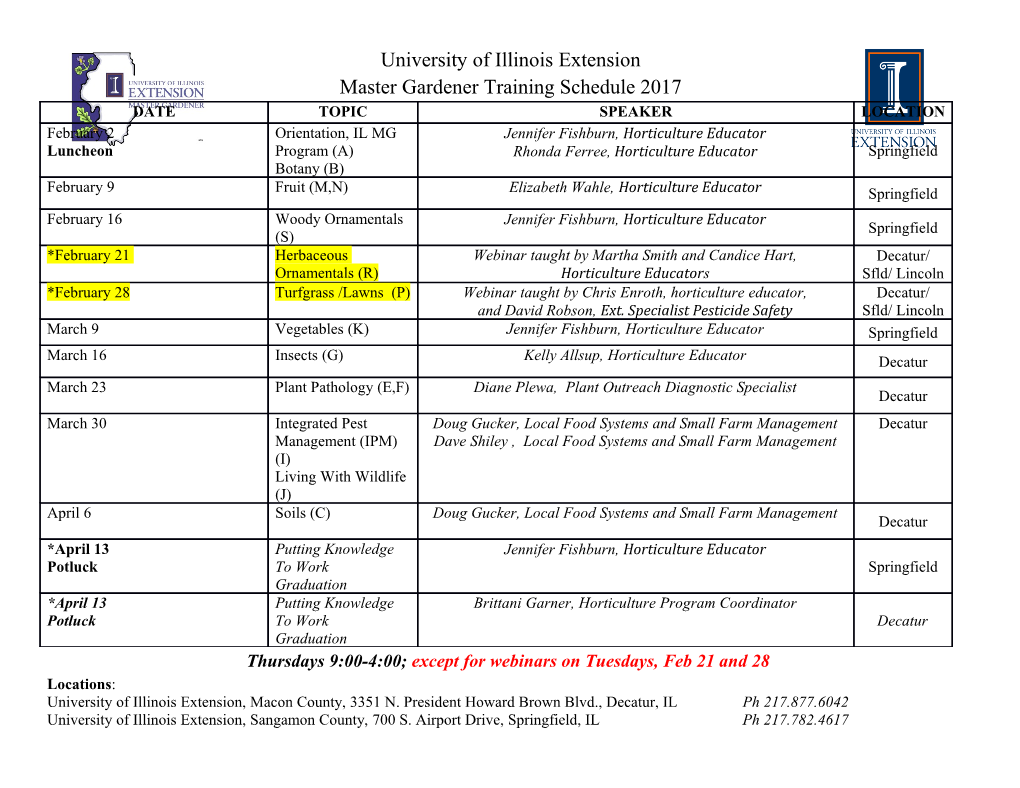
RS – EC2 - Lecture 16 Lecture 16 Unit Root Tests 1 Autoregressive Unit Root • A shock is usually used to describe an unexpected change in a variable or in the value of the error terms at a particular time period. • When we have a stationary system, effect of a shock will die out gradually. But, when we have a non-stationary system, effect of a shock is permanent. • We have two types of non-stationarity. In an AR(1) model we have: - Unit root: | 1 | = 1: homogeneous non-stationarity - Explosive root: | 1 | > 1: explosive non-stationarity • In the last case, a shock to the system become more influential as time goes on. It can never be seen in real life. We will not consider them. 1 RS – EC2 - Lecture 16 Autoregressive Unit Root • Consider the AR(p) process: 1 2 p (L) yt t where (L) 11L L 2 .... p L As we discussed before, if one of the rj’s equals 1, Φ(1)=0, or 1 2 .... p 1 • We say yt has a unit root. In this case, yt is non-stationary. Example: AR(1): yt 1 yt 1 t Unit root: 1 = 1. H0 (yt non-stationarity): 1 = 1 (or, 1 –1 = 0) H1 (yt stationarity): 1 < 1 (or, 1 –1 < 0) • A t-test seems natural to test H0. But, the ergodic theorem and MDS CLT do not apply: the t-statistic does not have the usual distributions. Autoregressive Unit Root • Now, let’s reparameterize the AR(1) process. Subtract yt-1 from yt: yt yt yt1 (1 1) yt1 t 0 yt1 t • Unit root test: H0: α0 = 1 –1 = 0 H1: α0 < 0. • Natural test for H0: t-test . We call this test the Dickey-Fuller (DF) test. But, what is its distribution? • Back to the general, AR(p) process: (L)yt t We rewrite the process using the Dickey-Fuller reparameterization:: yt 0 yt1 1yt1 2yt2 .... p1yt( p1) t • Both AR(p) formulations are equivalent. 2 RS – EC2 - Lecture 16 Autoregressive Unit Root – Testing 1 2 p • AR(p) lag Φ(L): (L) 1 1L L 2 .... p L • DF reparameterization: 2 2 3 p 1 p (1 L ) 0 1 ( L L ) 2 ( L L ) .... p 1 ( L L ) • Both parameterizations should be equal. Then, Φ(1)=-α0. unit root hypothesis can be stated as H0: α0=0. Note: The model is stationary if α0< 0 natural H1: α0 < 0. • Under H0: α0=0, the model is AR(p-1) stationary in Δyt. Then, if yt has a (single) unit root, then Δyt is a stationary AR process. • We have a linear regression framework. A t-test for H0 is the Augmented Dickey-Fuller (ADF) test. Autoregressive Unit Root – Testing: DF • The Dickey-Fuller (DF) test is a special case of the ADF: No lags are included in the regression. It is easier to derive. We gain intuition from its derivation. • From our previous example, we have: y t 1y t 1 t 0 y t 1 t • If α0 = 0, system has a unit root:: H0 :0 0 H1 :0 0 (|0 | 0) ˆ 1 • We can test H with a t-test: t 1 0 SE ˆ ˆ • There is another associated test with H0, the ρ-test:. (T-1)( −1). 3 RS – EC2 - Lecture 16 Review: Stochastic Calculus • Kolmogorov Continuity Theorem • If for all T > 0, there exist a, b, δ > 0 such that: a (1 + b) •E(|X(t1, ω) – X(t2, ω)| ) ≤ δ |t1 –t2| • Then X(t, ω) can be considered as a continuous stochastic process. – Brownian motion is a continuous stochastic process. – Brownian motion (Wiener process): X(t, ω) is almost surely continuous, has independent normal distributed (N(0,t-s)) increments and X(t=0, ω) =0 (“a continuous random walk”). Review: Stochastic Calculus – Wiener process • Let the variable z(t) be almost surely continuous, with z(t=0)=0. •Define(,v) as a normal distribution with mean and variance v. • The change in a small interval of time t is z • Definition: The variable z(t) follows a Wiener process if – z(0) = 0 – z = ε√t ,where ε〜N(0,1) – It has continuous paths. – The values of z for any 2 different (non-overlapping) periods of time are independent. Notation: W(t), W(t, ω), B(t). 1 Example: WT (r) (1 2 3 ... [Tr] ); r [0,1] T 8 4 RS – EC2 - Lecture 16 Review: Stochastic Process: Wiener process • What is the distribution of the change in z over the next 2 time units? The change over the next 2 units equals the sum of: - The change over the next 1 unit (distributed as N(0,1)) plus - The change over the following time unit --also distributed as N(0,1). - The changes are independent. - The sum of 2 normal distributions is also normally distributed. Thus, the change over 2 time units is distributed as N(0,2). • Properties of Wiener processes: – Mean of z is 0 – Variance of z is t – Standard deviation of z is √t n – Let N=T/t, then z (T ) z (0) i t i1 9 Review: Stochastic Calculus – Wiener process 1 1 Example: WT (r) (1 2 3 ... [Tr] ) S[Tr]; r [0,1] T T • If T is large, WT(.) is a good approximation to W(r); r Є[0,1], defined: W(r) = limT→∞ WT(r) E[W(r) ] =0 Var[W(r) ] =t • Check Billingsley (1986) for the details behind the proof that WT(r) converges as a function to a continuous function W(r). • In a nutshell, we need q - εt satisfying some assumptions (stationarity, E[|εt| <∞ for q>2, etc.) -a FCLT (Functional CLT). - a Continuous Mapping Theorem. (Similar to Slutzky’s theorem). 10 5 RS – EC2 - Lecture 16 Review: Stochastic Calculus – Wiener process • Functional CLT (Donsker’s FCLT) If εt satisfies some assumptions, then D WT(r) W(r), where W(r) is a standard Brownian motion for r Є [0, 1]. Note: That is, sample statistics, like WT(r), do not converge to constants, but to functions of Brownian motions. • A CLT is a limit for one term of a sequence of partial sums {Sk}, Donsker’s FCLT is a limit for the entire sequence {Sk} instead of one term. 11 Review: Stochastic Calculus – Wiener process 2 -1 • Example: yt = yt-1 + εt (Case 1). Get distribution of (X’X/T ) for yt. T T t1 T T2 (y )2 T2 [ y ]2 T2 [S y ]2 t1 ti 0 t1 0 t1 t1 i1 t1 T T2 [(S )2 2y S y2] t1 0 t1 0 t1 2 T S T S 2 t1 T1 2y T1/2 t1 T1 T1y2 0 0 t1 T t1 T t/T 2 t/T T 1 T 1 2 1/2 1 2 S[Tr] dr2y0T S[Tr] drT y0 T T t1 (t1)T t1 (t1)T 1 1 2 X (r)2dr2y T1/2 X (r) drT1y2 T 0 T 0 0 0 1 d 2 W(r)2dr, T . 12 0 6 RS – EC2 - Lecture 16 Review: Stochastic Calculus – Ito’s Theorem • The integral w.r.t a Brownian motion, given by Ito’s theorem (integral): ∫ f(t,ω) dB = ∑ f(tk,ω) ∆Bk where tk* є [tk,tk + 1) as tk + 1 –tk → 0. As we increase the partitions of [0,T], the sum →p to the integral. • But, this is a probability statement: We can find a sample path where the sum can be arbitrarily far from the integral for arbitrarily large partitions (small intervals of integration). • You may recall that for a Rienman integral, the choice of tk* (at the start or at the end of the partition) is not important. But, for Ito’s integral, it is important (at the start of the partition). 2 • Ito’s Theorem result: ∫B(t,ω) dB(t,ω) = B (t,ω)/2 – t/2. 13 Autoregressive Unit Root – Testing: Intuition • We continue with yt = yt-1 + εt (Case 1). Using OLS, we estimate : T T T yt yt 1 ( yt 1 yt 1 ) yt 1 yt 1yt 1 ˆ t 1 t 1 1 t 1 T T T y 2 y 2 y 2 t1 t1 t1 t 1 t 1 t 1 • This implies: T T yt 1yt 1 ( yt 1 / T )( t / T ) T (ˆ 1) T t 1 t 1 T 1 T y 2 ( y / T ) 2 t1 T t 1 t 1 t 1 • From the way we defined WT(.), we can see that yt/sqrt(T) converges to a Brownian motion. Under H0, yt is a sum of white noise errors. 7 RS – EC2 - Lecture 16 Autoregressive Unit Root – Testing: Intuition • Intuition for distribution under H0: - Think of yt as a sum of white noise errors. - Think of εt as dW(t). Then, using Billingley (1986), we guess that T(ˆ-1) converges to 1 W (t)dW (t) ˆ d 0 T ( 1) 1 W (t) 2 dt 0 • We think of εt as dW(t). Then, Σk=0 to t εk, which corresponds to ∫0to(t/T) dW(s)=W( s/T) (for W(0)=0). Using Ito’s integral, we have 1 W (1)2 1 T (ˆ 1) d 2 1 W (t)2dt 0 Autoregressive Unit Root – Testing: Intuition • 1 W (1) 2 1 T (ˆ 1) d 2 1 W (t) 2 dt 0 Note: W(1) is a N(0,1). Then, W(1)2 is just a χ2(1) RV.
Details
-
File Typepdf
-
Upload Time-
-
Content LanguagesEnglish
-
Upload UserAnonymous/Not logged-in
-
File Pages28 Page
-
File Size-