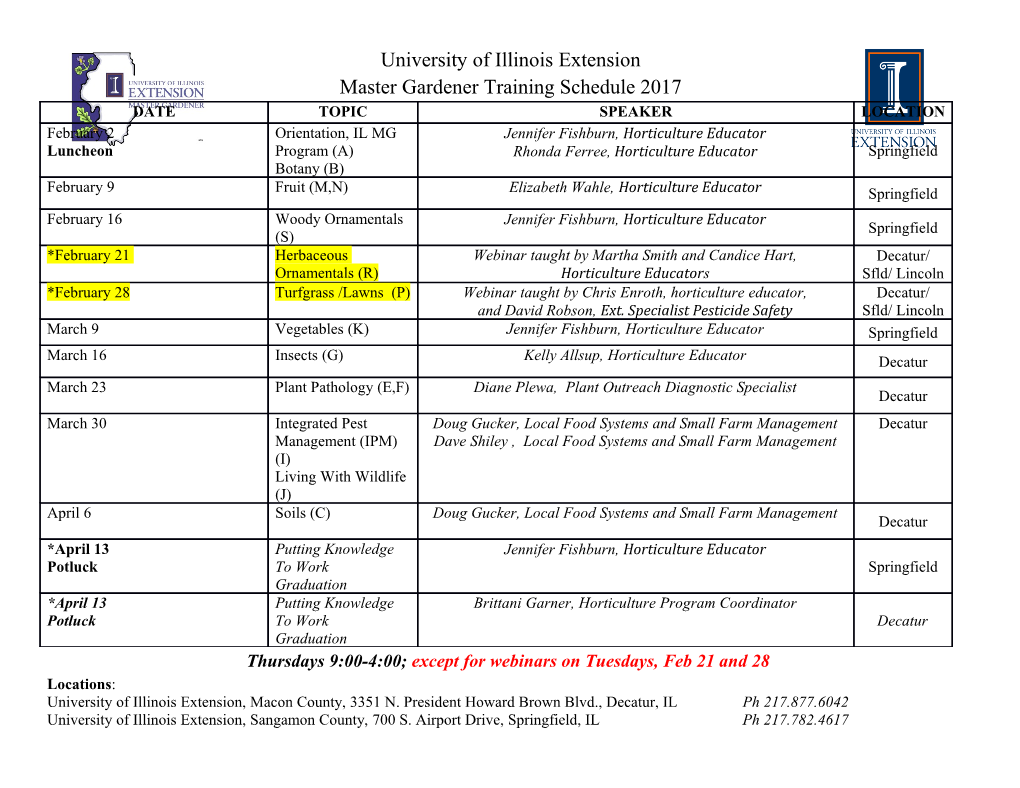
Visualisation : Lecture 4 Data Representation in Visualisation Visualisation – Lecture 4 Taku Komura Institute for Perception, Action & Behaviour School of Informatics Taku Komura Data Representation 1 Visualisation : Lecture 4 Data Representation ● We have a system architecture for visualisation – the visualisation pipeline – rendering techniques ● Data is ........ – discrete (in representation) – structured / unstructured (e.g. grid / cloud) – of a specific dimension D (e.g. 2D / 3D) Taku Komura Data Representation 2 Visualisation : Lecture 4 Discrete vs. Continuous ● Real World is continuous ● Data is discrete – Computers are good in handling discrete data – discrete representation of a real world (of abstract) concept ● Difficult to visualise continuous shape from raw discrete sampling? – we need topology and interpolation Taku Komura Data Representation 3 Visualisation : Lecture 4 Interpolation & Topology ● If we introduce topology our visualisation of discrete data improves – The topology helps the visual system to do the interpolation Taku Komura Data Representation 4 Visualisation : Lecture 4 Topology ● Topology : relationships within the data invariant under geometric transformation (i.e. Orientation, translation) i.e. the information which vertex is connected to which edge, which face is composed of which edges , etc. Taku Komura Data Representation 5 Visualisation : Lecture 4 Interpolation & Topology ● What if these colour represented spatial temperature at these 8 discrete points? – e.g. colour scale blue(=cold)→green→red( = hot) – How easy is it to visualise the temperature field over the whole cube? Taku Komura Data Representation 6 Visualisation : Lecture 4 Interpolation & Topology ● Use interpolation to shade whole cube: – Interpolation: increasing resolution of a discrete representation by producing intermediate samples — in example: producing intermediate colour pixels over cube topology from discrete vertex samples Taku Komura Data Representation 7 Visualisation : Lecture 4 How to interpolate over a rectangle? ● Will the red color dot on the right lower corner affect the color of the point near the left top? Taku Komura Data Representation 8 Visualisation : Lecture 4 Importance of representation ● What happens if we change the representation? ● Discrete data samples remain the same – topology has changed ⇒ effects interpolation ⇒ effects visualisation Taku Komura Data Representation 9 Visualisation : Lecture 4 How to interpolate in this case? ● Will the red color dot on the right lower corner affect the color of point near the left top? Taku Komura Data Representation 10 Visualisation : Lecture 4 Interpolation in digital images ● Some digital cameras use interpolation to produce a larger image than the sensor captured or to create digital zoom. ● Also using the topology data No interpolation Taku Komura With interpolation Data Representation 11 Visualisation : Lecture 4 Topological Dimension • Data has an inherent topological dimension – I.e minimum number of independent continuous variables needed to specify the location inside the data – Points : 0D, – curves : 1D, – surfaces : 2D, – volumes : 3D – Time dependent volumes : 4D Taku Komura Data Representation 12 Visualisation : Lecture 4 Data Representation ● Data objects : structure + value – referred to as datasets Abstract Concrete (e.g. VTK) Structure - Topology Consists of - Geometry Cells, points (grid) ————————— ————————— Data Attributes Scalars, vectors Normals, texture coordinates, tensors etc Taku Komura Data Representation 13 Visualisation : Lecture 4 What is a dataset? ● Dataset consists of 2 main components – structure of the data – value – attributes associated to particular parts of the structure – structure gives spatial meaning to the attributes values = { blue, green, green, green, green, green, turquoise, red} – – – attributes alone are meaningless without structure Taku Komura Data Representation 14 Visualisation : Lecture 4 Structure of Data ● Structure has 2 main parts – topology : — determines interpolation required for visualisation — “shape” of data OR – geometry — instantiation of the topology — specific position of points in geometric space Taku Komura Data Representation 15 Visualisation : Lecture 4 Concrete Representation of Datasets ● Points specify where the data is known – specify geometry in ℝN ● Cells allow us to interpolate between points – specify topology of points Point with known attribute data (i.e. colour ≈ temperature) Cell (i.e. the triangle) over which we can interpolate data. Taku Komura Data Representation 16 Visualisation : Lecture 4 Cells ● Fundamental building blocks of the shapes ● Various Cell Types – defined by topological dimension – specified as an ordered point list – primary or composite cells — composite : consists of one or more primary cells Taku Komura Data Representation 17 Visualisation : Lecture 4 Zero-dimensional cell types ● Vertex – Primary zero-dimensional cell – Definition: single point ● Polyvertex – Composite zero-dimensional cell — composite : comprises of several vertex cells – Definition: arbitrarily ordered set of points Taku Komura Data Representation 18 Visualisation : Lecture 4 One-dimensional cell types ● Line – Primary one-dimensional cell type – Definition: 2 points, direction is from first to second point. ● Polyline – Composite one-dimensional cell type – Definition: an ordered set of n+1 points, where n is the number of lines in the polyline Taku Komura Data Representation 19 Visualisation : Lecture 4 Two-dimensional cell types - 1 ● Triangle – Primary 2D cell type – Definition: counter-clockwise ordering of 3 points — order of the points specifies the direction of the surface normal ● Triangle strip – Composite 2D cell consisting of a strip of triangles – Definition: ordered list of n+2 points — n is the number of triangles ● Quadrilateral – Primary 2D cell type – Definition: ordered list of four points lying in a plane — constraints: convex + edges must not intersect Taku Komura Data Representation 20 Visualisation : Lecture 4 Two-dimensional cell types - 2 ● Pixel – Primary 2D cell, consisting of 4 points — topologically equivalent to a quadrilateral — constraints: perpendicular edges; axis aligned – numbering is in increasing axis coordinates ● Polygon – Primary 2D cell type – Definition: ordered list of 3 or more points — constraint: may not self-intersect Taku Komura Data Representation 21 Visualisation : Lecture 4 Three-dimensional cell types ● Tetrahedron – Definition: list of 4 non-planar points — Six edges, four faces ● Hexahedron – Definition: ordered list of 8 points — six quadrilateral faces, 12 edges, 8 vertices — constraint: edges and faces must not intersect ● Voxel – Topologically equivalent to Hexahedron – constraint: each face is perpendicular to a coordinate axis – “3D pixels” Taku Komura Data Representation 22 Visualisation : Lecture 4 Example Applications & Cell Types ● Lines – Segmentation of tissues from images ● Pixels – images, regular height map data ● Quadrilaterals – Finite element analysis ● Voxels : “3D pixels” – volume data : medical scanners Taku Komura Data Representation 23 Visualisation : Lecture 4 Attribute Data ● Information associated with data topology – usually associated to points or cells ● Examples : – temperature, wind speed, humidity, rain fall (meteorology) – heat flux, stress, vibration (engineering) – surface normal, colour (computer graphics) ● Usually categorised into specific types: – scalar (1D) – vector (commonly 2D or 3D; ND in ℝN) – tensor (N dimensional array) Taku Komura Data Representation 24 Visualisation : Lecture 4 Attribute Data : Scalar ● Single valued data at each location ozone levels elevation from reference plane – volume density (MRI) – simplest and most common form of visualisation data Taku Komura Data Representation 25 Visualisation : Lecture 4 Attribute Data : Vector Data ● Magnitude and direction at each location – 3D triplet of values (i, j, k) Wind Speed Force / Displacement Magnetic Field – Also Normals – vectors of unit length (magnitude = 1) Taku Komura Data Representation 26 Visualisation : Lecture 4 Attribute Data : Tensor Data ● K-dimensional array at each location – Generalisation of vectors and matrices – Tensor of rank k can be considered a k-dimensional table — Rank 0 is a scalar — Rank 1 is a vector — Rank 2 is a matrix — Rank 3 is a regular 3D array ● Tensor visualisation is an active area of research – covered later in lectures Taku Komura Data Representation 27 Visualisation : Lecture 4 Types of Dataset ● Defined by structure type: regular or irregular ● If there is a mathematical relationship between the point & cell positions it is regular – if the points are regular, the geometry is regular – if the cells are regular, the topology is regular ● Regular data can be implicitly represented – saves storage and computation (e.g. grid based representation) Taku Komura Data Representation 28 Visualisation : Lecture 4 Regular : Structured Points ● Points & cells arranged in a regular grid – axis aligned grid – for line elements (1D), pixels (2D) or voxels (3D) – e.g. images (2D), medical scanners (3D) – regular topology and geometry — uniform, equally spaced, axis aligned cells Taku Komura Data Representation 29 Visualisation : Lecture 4 Regular : Rectilinear Grid ● Points and cells arranged in a regular grid – topology is regular – geometry is partially regular – points are arranged along the axes but the spacing may vary – e.g. log-scale Taku Komura Data Representation 30 Visualisation
Details
-
File Typepdf
-
Upload Time-
-
Content LanguagesEnglish
-
Upload UserAnonymous/Not logged-in
-
File Pages38 Page
-
File Size-